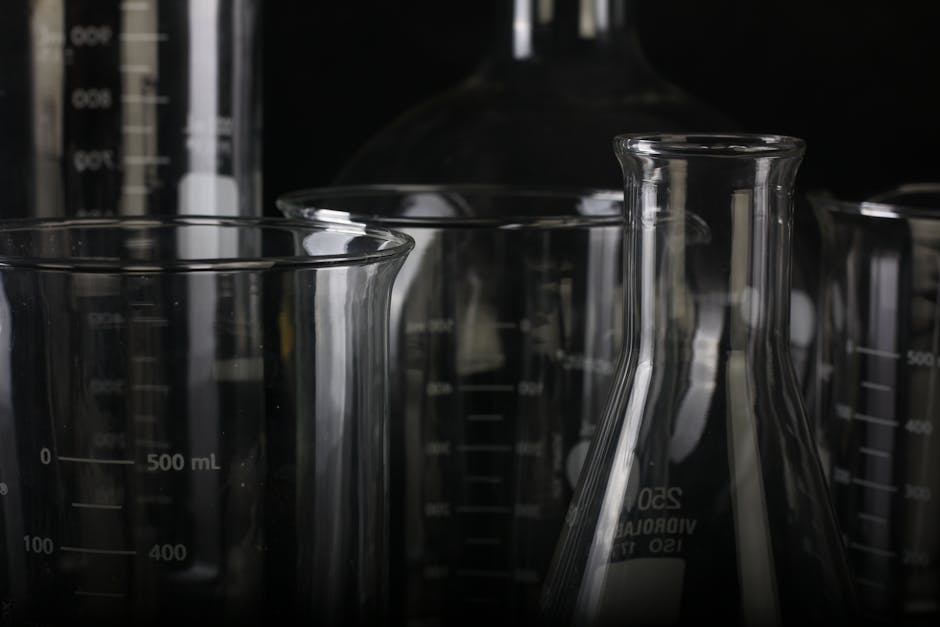
The Power of A/B Testing: Unleashing the Potential for Online Success
Before delving into the topic, let's start by acknowledging the significance of A/B testing. In today's data-driven world, online businesses are driven by the need to constantly optimize their websites, apps, and marketing strategies. A/B testing emerges as a powerful tool in this pursuit. By comparing two versions of a webpage or an element within it (A and B), businesses can objectively measure which version performs better in achieving specific goals. Here's an exploration of the basics of A/B testing and why it's crucial for success.
At its core, A/B testing is about experimenting with different versions of a variable to observe the impacts on user behavior and their corresponding outcomes. These versions, often referred to as variations or variants, are randomly divided among users to ensure statistical validity. Simply put, A/B testing enables businesses to make data-backed decisions through controlled experiments.
One key aspect to understand is that there must be a hypothesis behind each A/B test. It could be based on market research, user behavior insights, or even intuition. A robust hypothesis springs from a detailed analysis of the data and a thorough understanding of user preferences. By establishing clear objectives for every test, businesses can derive meaningful conclusions from the experimentation process.
To illustrate further: let's imagine an e-commerce website aiming to increase its sign-up rate for newsletters on their landing page. They choose to test two forms—a longer one with additional input fields (Version A) and a shorter one requiring minimal information (Version B). By running this A/B test on a fraction of their users, they can determine which variant results in higher engagement levels.
The data collected during an A/B test is essential for interpreting user behavior patterns accurately. Statistical significance influences the credibility of the results obtained. Typically, a larger sample size decreases the likelihood of false positive or false negative outcomes. Determining the correct duration of an experiment depending on sample size and traffic is crucial since insufficient data may lead to inconclusive outcomes.
Additionally, A/B testing allows businesses to identify and improve upon individual elements causing bottlenecks or specific objectives. Bottlenecks could include low click-through or conversion rates on certain pages or overall poor user engagement. By tweaking variations of those problematic elements, such as call-to-action buttons or headline text, valuable insights for optimization can be gained.
Ultimately, A/B testing plays a pivotal role in efficiently allocating resources towards optimizing performance. Both small businesses and large corporations employ this practice to ensure maximum returns from their online properties. With every successful test, a business can better understand its target audience, proactively respond to their needs, and significantly improve its digital presence.
In conclusion, A/B testing serves as a cornerstone for evidence-based decision-making in the digital landscape. It enables businesses to derive valuable insights from controlled experiments, leading to informed optimizations and ultimately, brand growth. Without understanding the basics of A/B testing and embracing its importance, digital success would largely rely on guesswork rather than reliable data-driven methods.
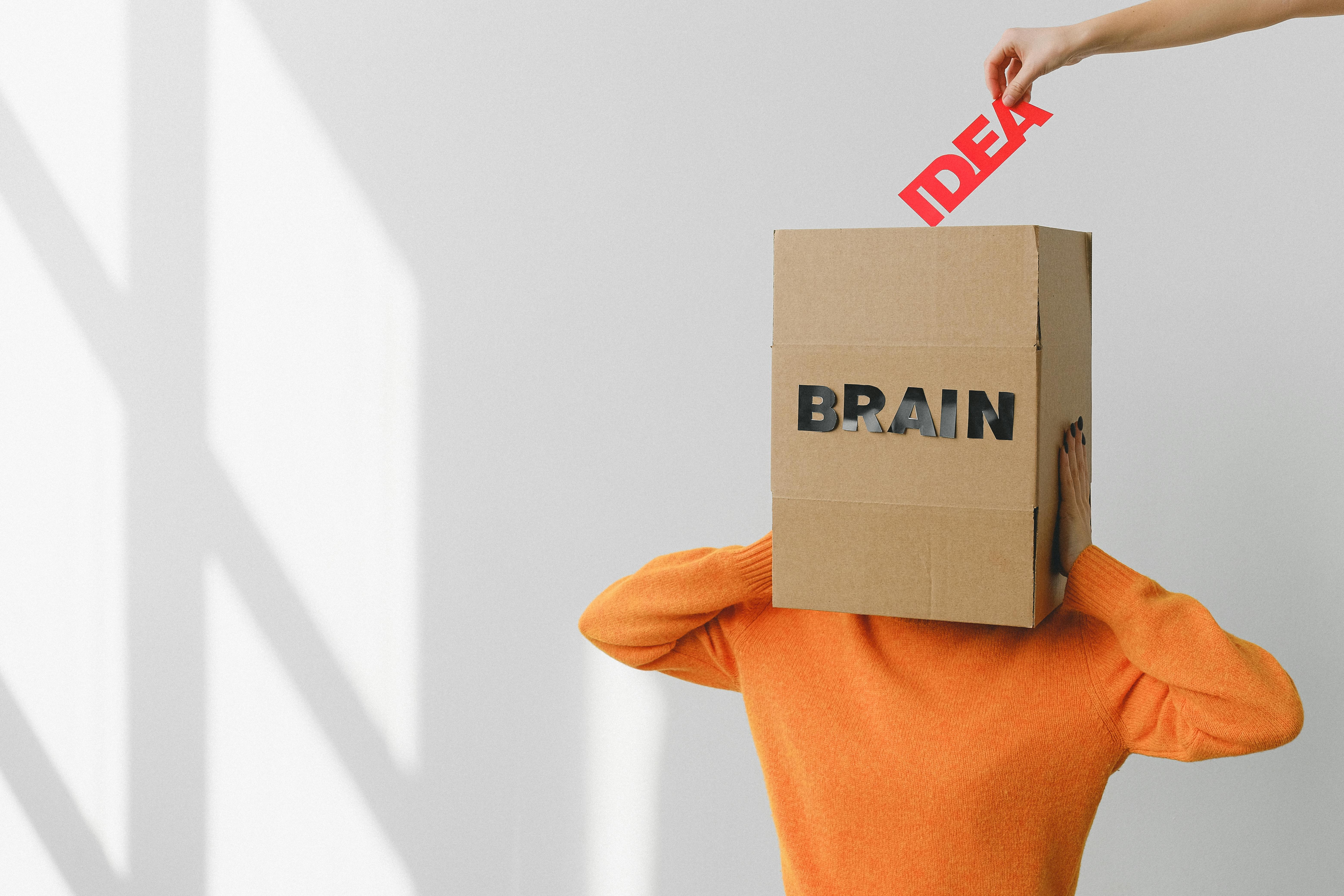
Essentially, A/B testing involves comparing two versions of a webpage or element within that page to see which performs better in terms of conversions. It enables businesses to make data-driven decisions by evaluating the impact of changes made on user behavior, whether it's changing the color of a button, altering the position of an image, or modifying the content layout.
One significant advantage of A/B testing is that it provides concrete evidence for optimization decisions. Instead of relying on assumptions or guesswork, A/B testing is based on statistical analysis that provides accurate insights into what actually influences user behavior and engagement. By gathering quantitative data, A/B testing eliminates subjective opinions and identifies the most effective design choices.
Website conversions are often measured by specific goals such as making a purchase, subscribing to a newsletter, or filling out a form. A/B testing allows businesses to test different variations of their webpages for these goals and determine which version leads to the highest conversion rate. By optimizing the website through continuous A/B testing, businesses can enhance overall performance and drive more action from their users.
Another crucial aspect of A/B testing is its ability to identify areas of improvement within a website. Visitors are more likely to leave a site if they encounter difficulties navigating or experience slow loading pages. By performing split testing on critical elements like navigation menus, search bars, and forms, businesses can identify pain points that hinder smooth user experience. By making incremental changes based on these insights, website owners can enhance overall user satisfaction and reduce the risk of losing potential customers.
In addition to improving conversion rates, A/B testing increases confidence in design decisions by mitigating risks associated with major website updates or overhauls. Instead of making significant changes based on assumptions, businesses can use A/B testing to validate design modifications incrementally and only implement those that have proven success.
To carry out an effective A/B test, it is important to experiment with variations on a single element at a time. This ensures that the impact observed directly corresponds to the tested change. Testing multiple elements simultaneously can lead to confounding variables and invalid conclusions.
A/B testing is an ongoing process that grows along with a business. It involves continuously refining and optimizing various web elements to maintain optimal performance. By investing time and resources in A/B testing, businesses can enhance their website conversions and foster long-term success.
In conclusion, A/B testing is a powerful tool that allows businesses to gather data-driven insights and optimize their websites for better conversions. Its ability to determine what works best for target audiences not only improves user experience but also boosts bottom-line results. An iterative process, A/B testing empowers businesses to make informed design decisions, leading to a more successful online presence.
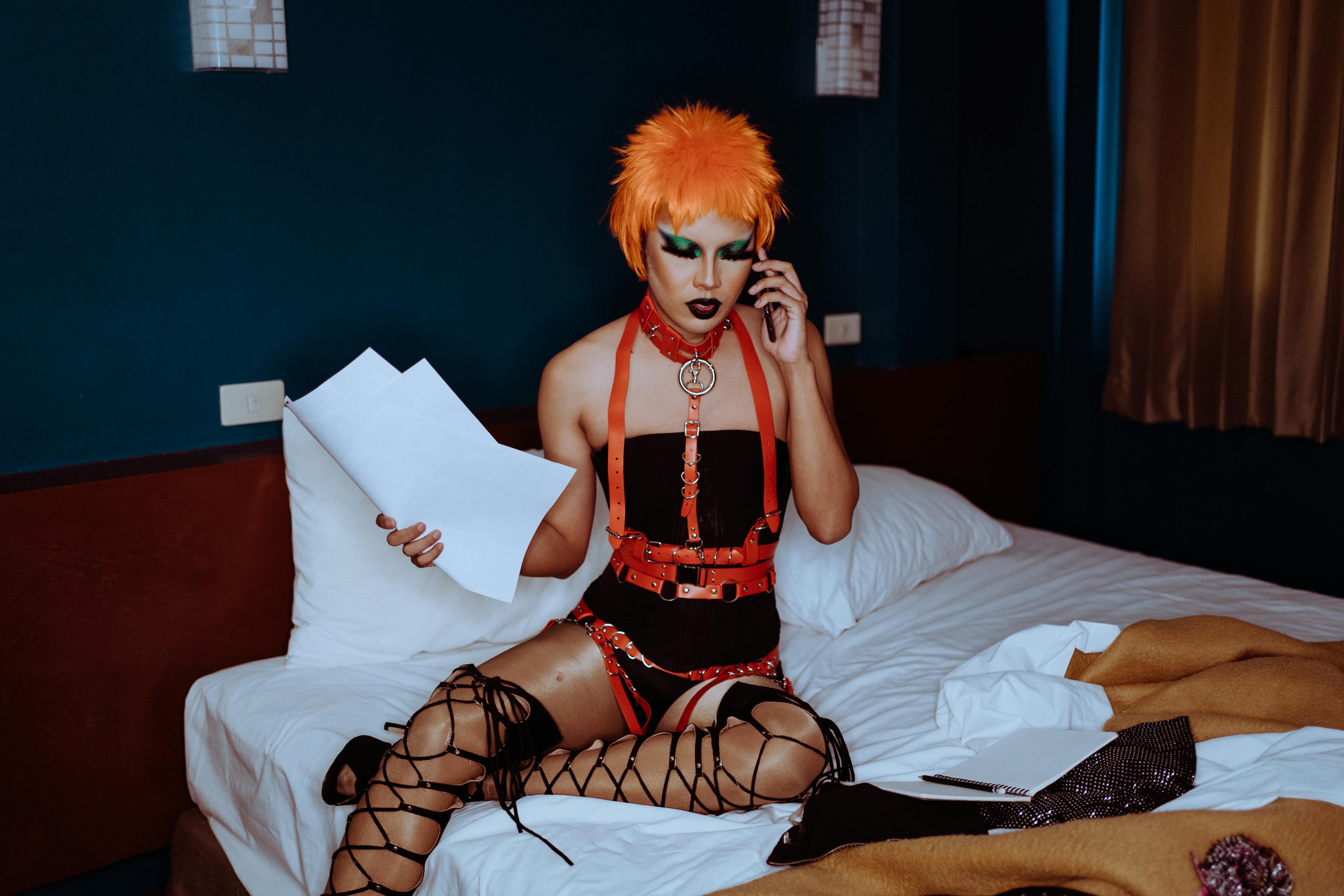
Marketing Emails: By tweaking different elements such as subject lines, call-to-action buttons, or visual layout, businesses witnessed substantial changes in email campaign success rates. For instance, a changed subject line resulted in higher open rates and engagement, ultimately leading to increased conversions.
Website Design: Even subtle changes to website elements can affect user behavior and overall performance. Modifying the color or placement of 'Buy Now' buttons or simplifying the checkout process markedly impacted conversion rates for online retailers.
Landing Pages: Tweaks to landing pages can significantly increase sign-ups or purchases. Adjustments like reducing form fields, altering headlines or visuals, and enhancing clarity in the value proposition have furnished surprising results regarding conversions.
Homepage Optimization: Minor upgrades to the homepage layout, copywriting, navigation structure, or incorporating social proof like testimonials saw noteworthy improvements in engagement metrics, ultimately influencing lead generation or sales volume.
Pricing Strategy: By modifying pricing structures subtly (e.g., displaying prices without commas, altering font size), companies observed variations in customers' likelihood to purchase certain products/services. Even fractional pricing changes targeting psychological aspects of purchasing decisions brought positive transformations.
Button Text: Replacing generic button texts such as 'Submit' with more action-oriented alternatives ("Get Started," "Join Now") led to drastic spikes in click-through rates and successful interactions.
Mobile App Enhancements: Adjusting elements like color schemes, icon layouts, or simplifying registration processes caused significant increases in user retention rates for mobile apps. Subtle adjustments often played a vital role in establishing a successful user experience.
Headline Optimizations: Minor changes to headlines can catch users' attention and arouse curiosity, resulting in higher click-through rates for articles, blog posts, or advertisements. Testing different narrative angles or emotional triggers has often yielded surprising wins.
By examining these case studies, it's evident that substantial outcomes can be achieved by making small changes and conducting precise A/B tests. The key lies in focusing on specific elements relevant to the desired outcome, being open to exploring alternatives, and rigorously evaluating the results to make data-driven decisions.
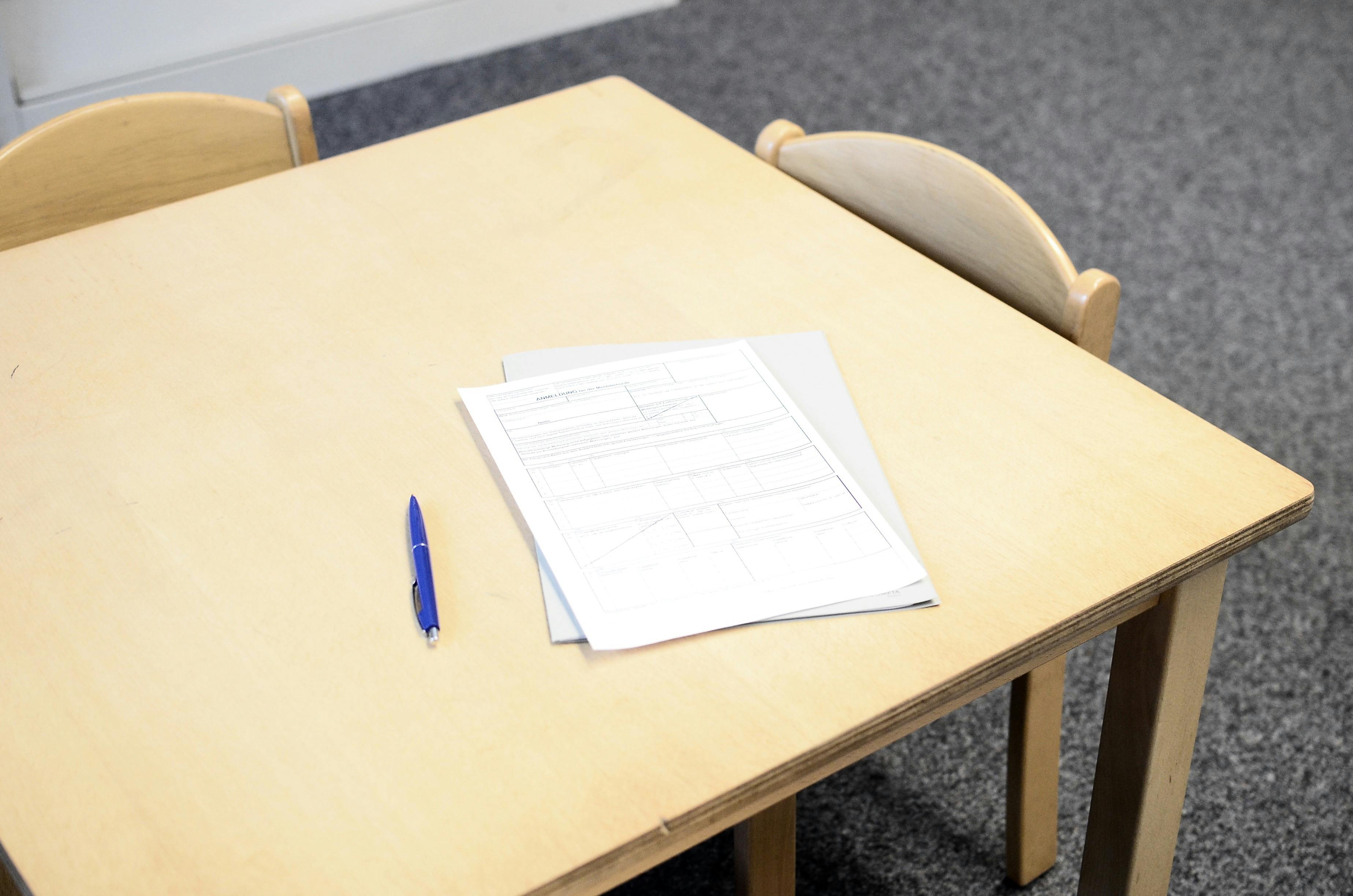
When it comes to conducting A/B tests, crafting thorough and well-defined hypotheses is crucial to ensure effective testing strategies. A hypothesis serves as a statement or prediction that helps guide the test and validate or negate the desired outcome. Without a properly crafted hypothesis, an A/B test can easily be aimless and inconclusive. Here are some important points to consider when crafting hypotheses for effective A/B testing strategies:
1. Start with a clear objective:
Clearly define the objective or problem you want to solve through the A/B test. Identifying a specific goal will help you focus your efforts and generate meaningful insights. It could be anything from increasing website conversions, improving user engagement, enhancing click-through rates, or optimizing marketing campaigns.
2. Formulate a clear hypothesis:
Craft a clear and concise hypothesis that directly reflects what you wish to achieve or investigate in your A/B test. The hypothesis should consider both the control and experimental variations (designated as A and B), allowing you to accurately compare which version performs better against your objective.
3. Specify variables and metrics:
Clearly identify the variables that you plan to change between the control and experimental groups. Variables can be anything from design elements, headline text, call-to-action buttons, pricing structures, or any other area relevant to your goals. These variables serve as inputs that might impact the desired outcome metric(s).
4. State expected impacts:
State the expected impact of the changes made in the variables on the target metrics. For instance, if you believe that changing the color of a button will enhance user engagement, outline how this alteration is expected to improve specific metrics like click-through rates or conversion rates.
5. Consider sample size:
When crafting your hypothesis, it's essential to consider the appropriate sample size required for reliable results. Large enough sample sizes increase statistical power and prevent drawing misleading conclusions due to random variations. Carefully calculate the size of your groups pre-test to ensure statistical significance.
6. Define success metrics:
Define the metrics you will use to evaluate the success or failure of each variant in your A/B test. These metrics depend on your objective and may include things like conversion rates, revenue per user, bounce rates, engagement rates, or any other metrics directly related to your goal.
7. Time duration considerations:
Keep in mind the time duration required for your A/B tests. Longer tests have more data points which increase statistical significance but might slow down decision-making processes. Conversely, running short tests may not provide sufficient data for reliable results. Take into account factors such as seasonality and traffic patterns that might affect test outcomes.
Crafting hypotheses for effective A/B testing strategies requires careful consideration so that you can accurately measure the effects of changes made and draw actionable conclusions. Incorporating these guidelines will help ensure that you establish clear goals, generate meaningful insights, and make informed decisions from the results obtained during your A/B tests.
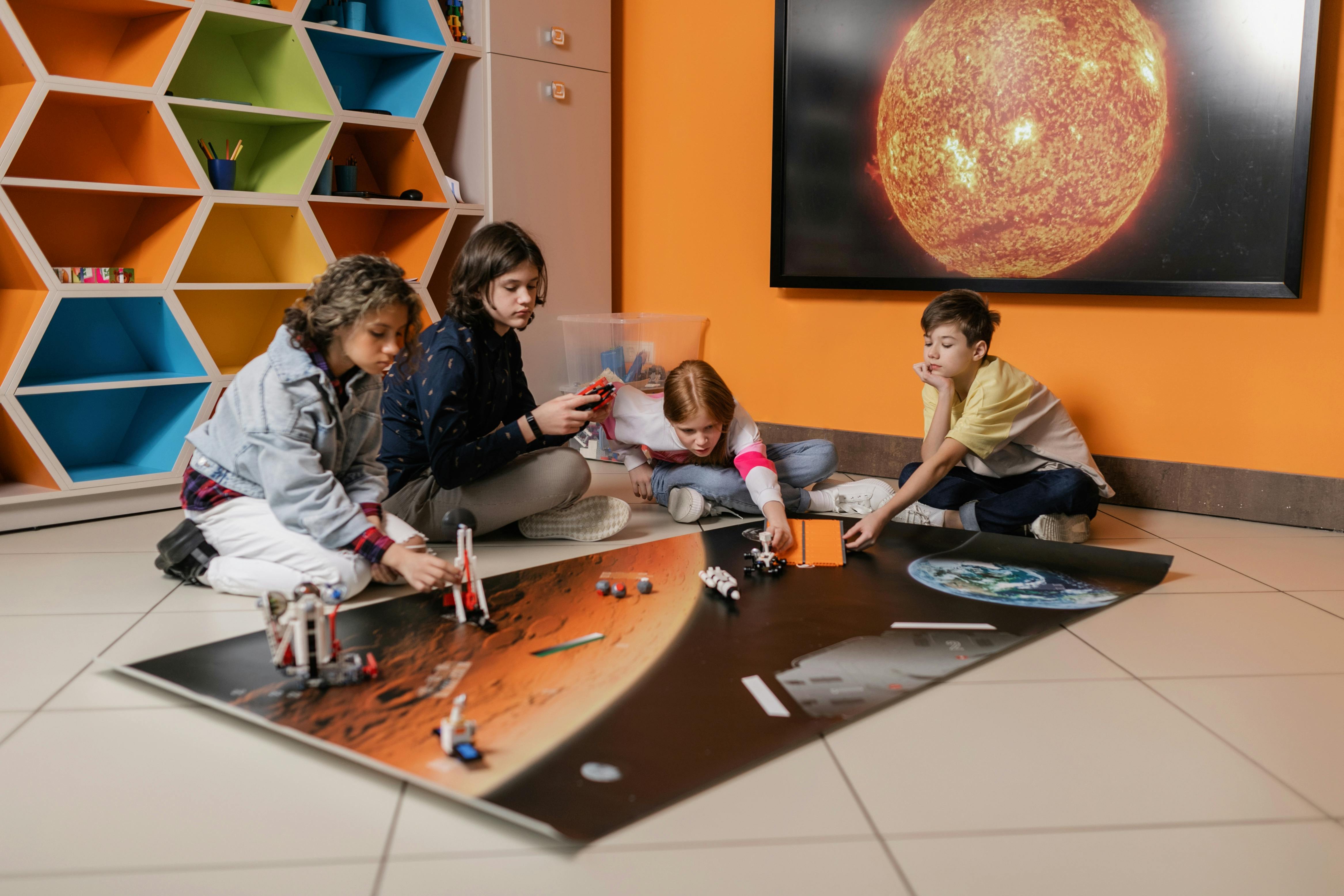
One prominent digital space where A/B testing can be utilized is mobile applications. By testing different features, layouts, colors, or calls-to-action within an app, developers and marketers can understand users' preferences and assess which version generates better engagement or drives more conversions. For instance, by analyzing the impact of variation A's button placement versus variation B's on-screen style, it becomes possible to determine the configuration that yields higher interaction rates.
Furthermore, A/B testing is not solely reserved for web-based or mobile interfaces. It can be extended to email marketing campaigns as well. Through experimentation with diverse elements such as subject lines, sender names, email copy, imagery, or the placement of CTAs (Call to Actions), marketers can identify email components that resonate best with their target audience. Splitting an email recipient list into two groups - one receiving variation A and the other receiving variation B - allows for tracking open rates, click-through rates (CTRs), and ultimately myriads of conversions effectively.
Social media platforms are also fertile grounds for exploring A/B testing. Brands can investigate different strategies like using specific hashtags or captions in Instagram posts to analyze which approach garners more engagement. Similarly, by running parallel advertising campaigns with distinct creatives on Facebook, marketers can optimize their targeting settings by monitoring the performance metrics of each version, such as click-through rates or conversion rates.
Another avenue where A/B testing proves pivotal is landing pages optimization. These pages are crucial for driving visitors towards desired actions like making a purchase or submitting a form. Experimenting with variations of headlines, copy, images, colors, layout, or forms can provide valuable insights into which page configuration leads to higher conversions. By running simultaneous tests with equivalent traffic segments and comparing the conversion rates yielded by the variations, marketers can fine-tune the content for maximum effectiveness.
Lastly, A/B testing can be employed effectively in paid search or search engine marketing (SEM) campaigns. Altering ad copies, headlines, or even landing page URLs can make a significant impact on click-through rates and consequently conversions. For instance, comparing different ad formats or Call-to-Action text using identical search audience segments enables businesses to tailor their messaging to align with user preferences based on empirical data.
In conclusion, while A/B testing has predominantly been associated with websites, its efficacy extends beyond traditional website optimization. Mobile apps, email marketing campaigns, social media platforms, landing pages, paid search campaigns, and other digital touchpoints all offer opportunities for in-depth experimentation using this methodology. By employing A/B tests across various digital platforms intelligently and astutely analyzing the resulting data insights, companies can make sound decisions to continually enhance their digital strategies and deliver more impactful user experiences.
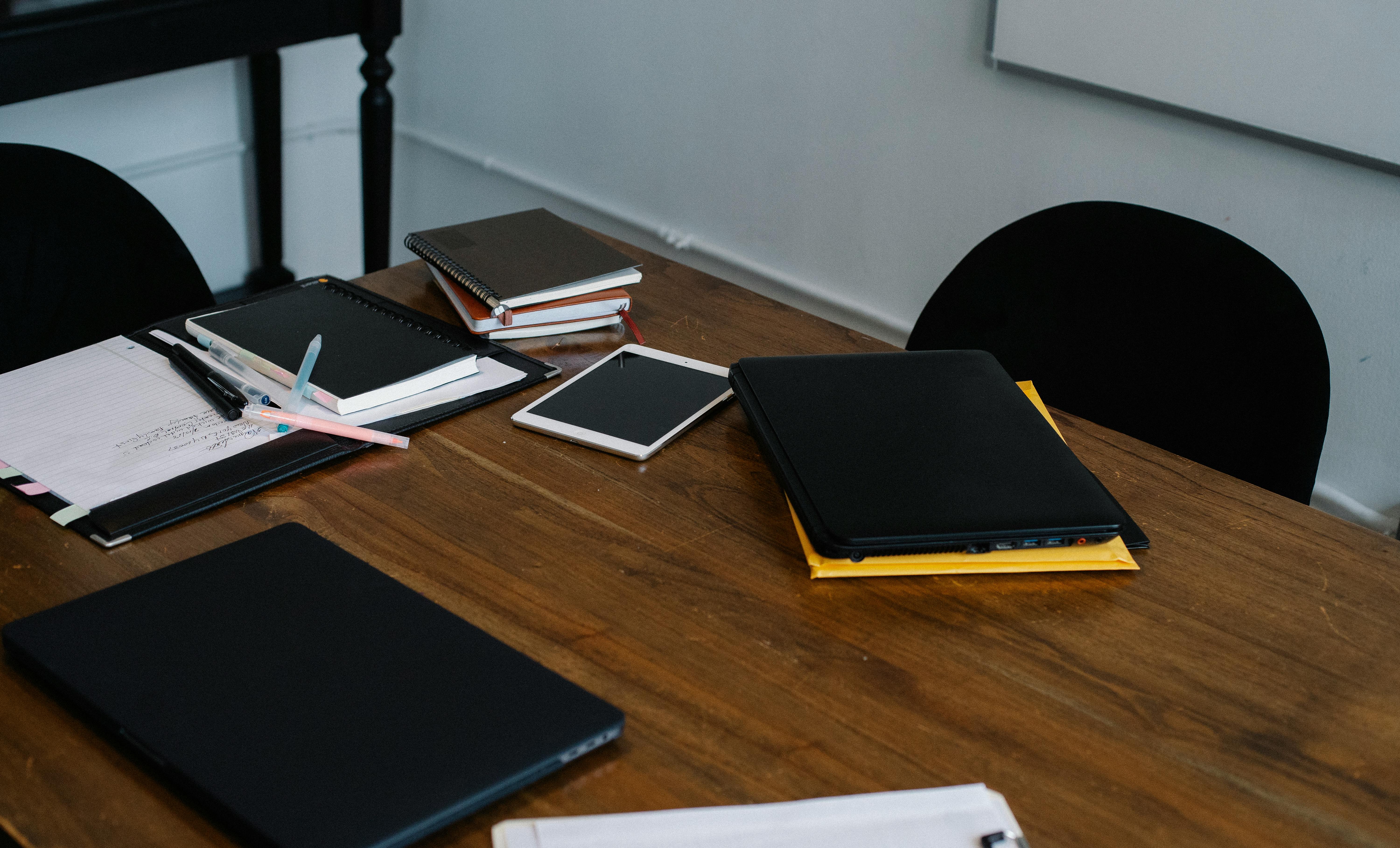
- Clearly define objectives: Start by understanding the goals you want to achieve through the A/B test. Clearly define the questions you want to answer or the improvements you aim to make.
- Identify a specific target audience: Determine who your target audience is and segment them appropriately. This allows you to tailor each variant of your test to different user groups, improving the relevance and accuracy of your results.
- Conduct proper research: Before designing your A/B test, conduct thorough research and gather data about your audience and their preferences. Understanding their behavior and needs will help you create informed variants.
- Ensure statistical significance: A critical aspect of A/B testing is achieving statistical significance, which means that the sample size is large enough to make confident conclusions about the results. Calculate the required sample size in advance to minimize false positives or negatives.
- Test one element at a time: To accurately measure the impact of a change, isolate a single variable or element per test. Testing multiple variables simultaneously can lead to confusion and inaccurate conclusions.
- Develop clear hypotheses: Clearly articulate what you expect to happen when making a change. These assumptions help guide your test design and allow for meaningful interpretation of outcomes.
- Randomize and distribute evenly: Randomizing assignments helps eliminate biases and ensures fairness in your test. Additionally, evenly distribute traffic between variants to increase reliability.
- Track essential metrics: Determine appropriate metrics for assessing success (such as conversions, bounce rate, or click-through rates), track them diligently throughout the test, and compare across variants.
- Monitor the duration of tests: Collect data for a sufficient duration to account for variations over time, such as daily or weekly patterns that could influence results.
- Continuously analyze and iterate: Regularly review data during the course of your A/B test – don't wait until it concludes. Analyze interim results to identify any trends or opportunities for improvement, ensuring your test is effective in reaching its objectives.
- Document and share findings: Once your A/B test concludes, document the results, insights, and conclusions you obtained. Share them with stakeholders to promote knowledge-sharing and inform decision-making for future tests or marketing campaigns.
- Learn from failures: Even if a test doesn't yield the desired outcomes, it doesn't mean it was unsuccessful. Use any failures or unexpected results as learning opportunities to refine your future tests and optimize strategies.
By following these best practices, you can design effective A/B tests that inform data-driven decisions, improve user experience, and drive the success of your blog or website.Analyzing A/B Testing Results: Turning Data into Actionable InsightsAnalyzing A/B testing results is a crucial step in turning data into actionable insights. It allows you to understand the impact of changes made during the test and make informed decisions based on this analysis. Here are some key points about analyzing A/B testing results:
1. Define clear goals: Before starting an A/B test, clearly define what you want to achieve. Clearly understanding your goals will help in analyzing the results effectively.
2. Collect relevant data: Ensure that you collect relevant and meaningful data throughout your A/B test. This may include tracking user behavior, conversion rates, engagement metrics, and other key performance indicators.
3. Statistical significance: When analyzing the A/B test results, focus on statistical significance. This helps determine if the observed differences in metrics between variations are real or just due to random chance.
4. Key metrics: Identify the key metrics that are most important for your business goals and analyze how they vary between different variations of the test. For example, if the goal is to increase conversions, closely examine conversion rates.
5. Segment analysis: Segmenting your data analysis enables you to dig deeper into understanding how different user groups respond to your A/B test variations. By segmenting your audience based on attributes like demographics or behavior patterns, you can gain insights on specific segments.
6. Data visualization: Utilize visual aids like charts and graphs to present the results of your A/B test analysis more effectively. Visualizations make it easier to identify trends, patterns, and differences between variations.
7. Comparison and significance tests: Use appropriate statistical tests like t-tests or chi-square tests to compare the performance and measure the statistical significance of differences between variants.
8. Consider sample size and duration: Make sure you have a sufficient sample size and test duration to ensure statistical validity. Inadequate sample sizes or insufficient durations may lead to unreliable results.
9. Qualitative feedback: Incorporate qualitative feedback by gathering user feedback or conducting surveys to gain a deeper understanding of user preferences and opinions regarding the variations being tested.
10. Learn from failed tests: Not every A/B test will yield positive results. Analyzing unsuccessful tests is equally important as successful ones, as it provides valuable insights on what doesn't work and helps avoid potential mistakes in future experiments.
11. Iterate and optimize: Use the insights gained from analyzing A/B testing results to identify optimizations and refine your strategy. Apply the lessons learned to future tests and keep improving your website, app, or product.
In summary, analyzing A/B testing results is about gaining actionable insights and informed decision-making by carefully examining relevant data, identifying key metrics, ensuring statistical significance, utilizing visualization techniques, considering qualitative feedback, and learning from both successful and failed tests. Continuous iteration and optimization will drive meaningful improvements based on these insights.
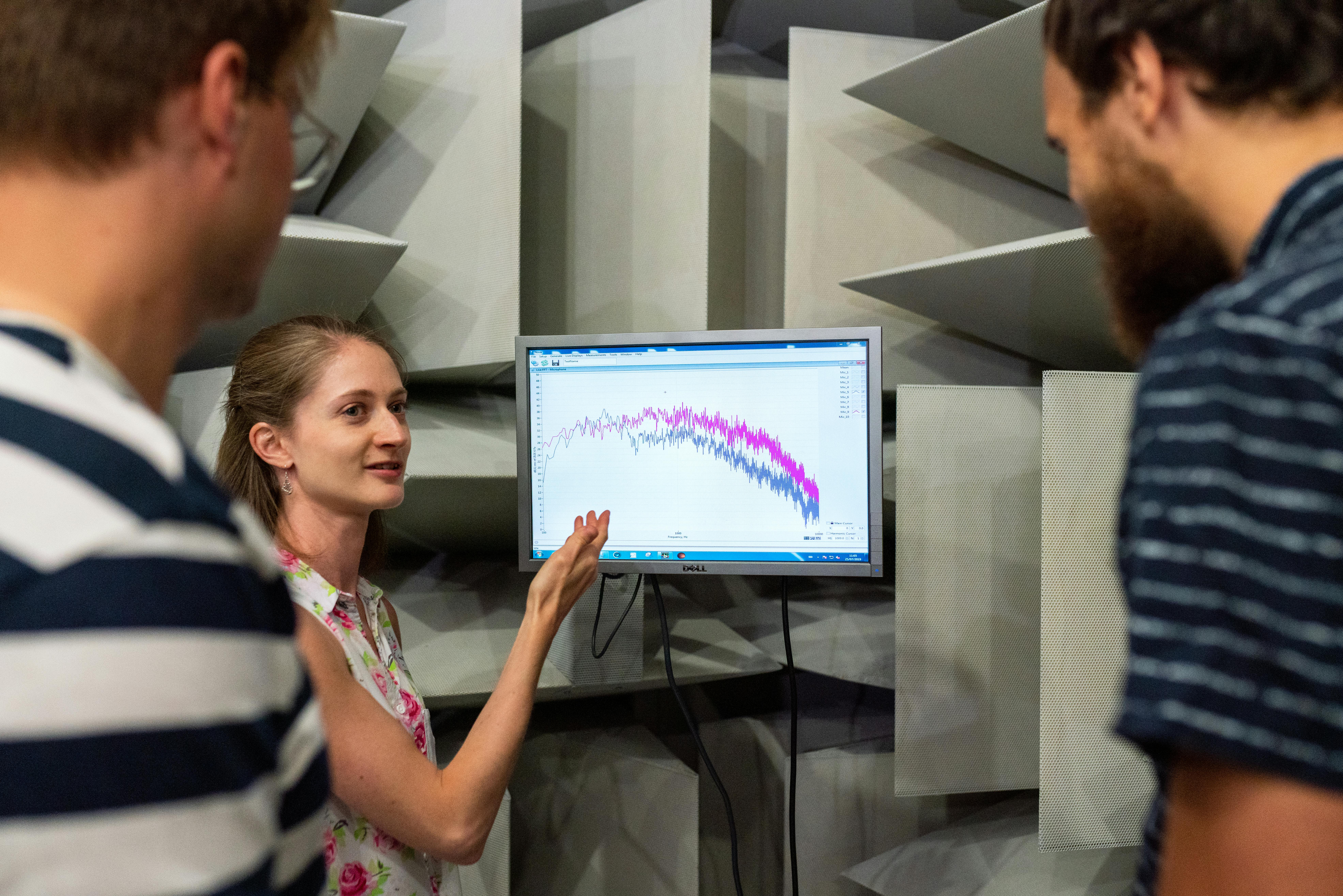
1. Insufficient Sample Size: One major pitfall is conducting A/B tests with insufficient sample sizes, which may lead to flawed conclusions or false positives/negatives. It's crucial to calculate the statistical power needed and run tests for an appropriate duration to generate reliable data.
2. Rushing into Conclusions: Jumping to conclusions too soon is another pitfall. Analyzing results prematurely before achieving statistical significance can lead to misguided decisions. Give tests ample time to run and collect sufficient data before drawing valid inferences.
3. Multiple Comparisons: Engaging in multiple comparisons within a single A/B test without accounting for multiplicity issues can significantly increase the likelihood of false positives. Utilizing statistical methods like Bonferroni correction can help mitigate this challenge.
4. Simpson's Paradox: When averages from different segments of a population are combined, Simpson's Paradox might arise, providing a contradictory conclusion compared to individual subgroups. To avoid misleading results, analyze segment-level data alongside aggregate statistics.
5. Biased Samples: Another principal pitfall is having biased samples due to improper randomization techniques or participants self-selecting into groups. Ensure random assignment and consider stratification based on relevant variables (e.g., demographics) to minimize potential bias effects.
6. Novelty Effects: Users may respond differently due to the novelty factor of a new design or feature being tested, leading to unreliable outcomes during A/B tests. To mitigate this, consider using a control group or implementing long-term tests to minimize short-lived effects.
7. Inconsistent Traffic Allocation: Unequal distribution of traffic between variants might result in uneven exposure and imbalanced testing conditions. Make sure to allocate equal proportions of traffic to all variants throughout the test for accurate comparison.
8. Non-Representative Timeframes: Generation of biased results due to disparities among weekdays, holidays, or varying periods is another pitfall. To avoid this, ensure that the test covers a representative timeframe and accounts for seasonal variations.
9. Ignoring External Factors: External factors beyond the tested webpage or app can influence user behavior, potentially affecting the accuracy of A/B test results. Monitor external influences, document changes, and consider conducting parallel control experiments to isolate such effects.
10. Overlooking Heterogeneous Treatment Effects: Failing to recognize that treatment effects may differ among various subgroup demographics can lead to overlooking crucial insights. Analyze results by different customer segments to validate consistency across diverse user groups.
In conclusion, A/B testing provides substantial benefits when executed carefully. By being aware of these common pitfalls and implementing appropriate methodologies, you can enhance the reliability and significance of A/B test results, leading to data-driven decisions that optimize your webpages or apps for improved user experience and better business outcomes.Integrating A/B Testing into Your Overall Marketing StrategyIntegrating A/B testing into Your Overall Marketing Strategy
A crucial element of optimizing your marketing efforts is the practice of A/B testing, a highly effective method that helps evaluate and improve your strategies. By comparing two versions (A and B) of a particular element or campaign, A/B testing allows you to understand which variant performs better and make data-driven decisions to achieve higher conversions and engagement. Here are some important aspects to consider when integrating A/B testing into your overall marketing strategy.
Begin With Clear Objectives: Before conducting any A/B tests, establish specific objectives that align with your marketing goals. Whether it's improving click-through rates on ad campaigns or enhancing user experience on your website, being clear about what you want to accomplish is crucial for designing effective experiments.
Target Specific Areas for Testing: Choose the elements you wish to test carefully, focusing on those that have significant impact potential. These elements could include ad headlines, landing page layouts, call-to-action buttons, email subject lines, or even entire marketing campaigns. Prioritize areas that generate substantial traffic or usually receive high user interactions.
Formulate Hypotheses: For each element you decide to test, it's important to formulate reasonable hypotheses based on data insights or industry best practices. Hypotheses should be specific and measurable and can cover aspects such as user behavior patterns, preferences, or expectations.
Divide Audiences: To accurately compare the performance of different variants, split your target audience into separate groups. Group A will be exposed to Variant A, while Group B will see Variant B. Ensure that the split is random and appropriately proportioned to ensure validity and statistical significance in your results.
Monitor Results Closely: During the testing phase, track key metrics such as conversion rates, click-through rates, bounce rates, or engagement levels closely. Regularly analyze data from both variants to determine which is outperforming the other based on pre-defined success metrics.
Time and Sample Size: Allow sufficient time for tests to run, making sure they reach an adequate sample size to generate reliable results. Ending tests prematurely or with small sample sizes may lead to inaccurate conclusions. Set a predetermined duration or required sample size before evaluation.
Draw Insights and Implement Changes: Once you have enough data, analyze the comparison between variants to draw actionable insights. Identify patterns, trends, or statistically significant results that support one variant over the other. Incorporate these findings into your future marketing campaigns and make necessary changes based on improved strategies.
Continuous Testing and Improvement: A/B testing should not be a one-time effort. To continuously optimize your marketing strategy, embrace a culture of testing by experimenting systematically. With each A/B test, aim to enhance conversion rates, increase customer satisfaction, and fine-tune your marketing approaches based on real user behavior.
In conclusion, integrating A/B testing into your overall marketing strategy helps elevate performance by making well-informed decisions supported by data insights. By following the steps outlined above, you can leverage the power of A/B testing to refine your marketing efforts over time and achieve better results in engaging your target audience.
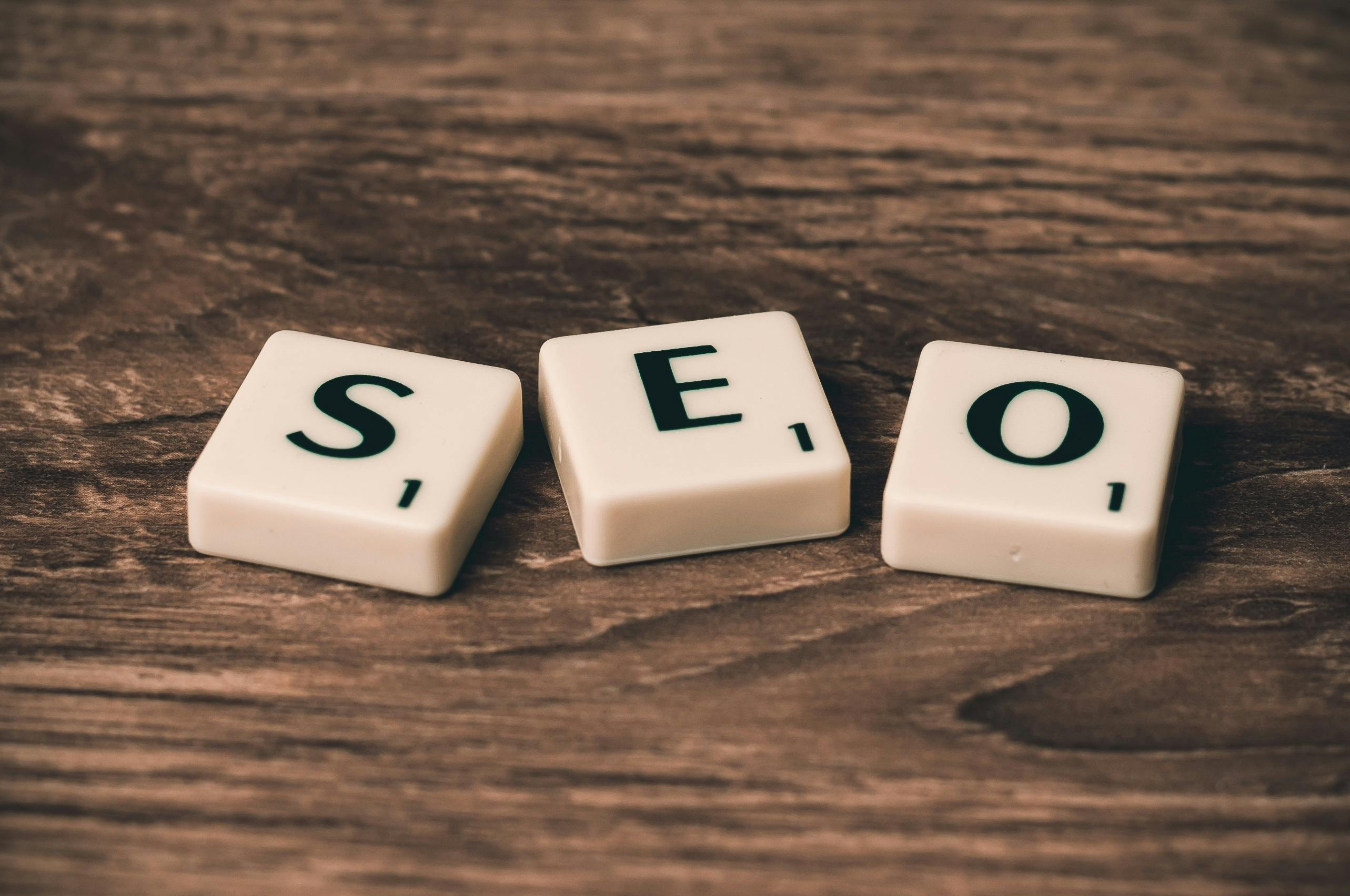
One popular tool is Google Optimize, which allows you to easily create and manage A/B tests on your website without any coding knowledge. It provides a visual editor for creating variations of web pages and supports various targeting options, making it user-friendly for marketers and analysts.
Optimizely is another widely used platform that offers A/B testing capabilities combined with advanced targeting options. It provides an intuitive visual editor for creating experiments and comes with powerful analytics and reporting features, making it ideal for teams of different sizes.
VWO (Visual Website Optimizer) is a comprehensive conversion optimization platform that enables businesses to conduct A/B tests on websites, landing pages, forms, and more. It offers a drag-and-drop editor for test creation, heatmaps to analyze user behavior, surveys for gathering feedback, and supports audience segmentation.
Unbounce is specifically designed for optimizing landing pages through split testing. It helps marketers create separate landing page variants and assess their performance to improve conversion rate. Its built-in features include AMP page support, dynamic text replacement, integrated form builder, and an extensive template library.
Hotjar provides usability testing tools such as heatmaps, session recordings, survey tools, and funnel analysis. It assists in visualizing user behavior on various test variants by generating heatmaps based on user interactions. This valuable information can guide optimizations to improve conversion rates.
Kissmetrics offers behavioral analytics in addition to A/B testing capabilities. By understanding how users behave at each stage of their journey on your website or app, you can identify areas that need improvement and make data-driven decisions. It enables deep segmentation, funnel analysis, cohort reports, and more, helping you identify trends and preferences within your user base.
Segment is a powerful customer data platform that provides the foundation for better A/B testing. It allows you to collect, store, and manage user data from various sources, which can be utilized in designing tests targeted at specific segments. Effectively managed data provides crucial insights into user behavior and helps drive effective optimization.
In addition to these specialized tools, various testing frameworks like React Testing Library (RTL) and Selenium WebDriver provide robust options for conducting A/B tests with code. These allow developers to write automated scripts to interact with web elements and test various variations of a webpage. Such frameworks facilitate efficient scripted A/B testing and integration into software development pipelines.
Overall, these tools and technologies greatly enhance the power of A/B testing by simplifying experiment creation, analyzing user behavior, delivering actionable insights, and facilitating seamless integration into different workflows. Whether you are a marketer, analyst, or developer, having an arsenal of these resources is crucial for successfully driving improvements through A/B testing.The Future of Online Experiments: Beyond Basic A/B TestsIn the rapidly evolving landscape of technology and data analytics, online experiments have become a fundamental tool for businesses seeking to optimize their digital presence. While basic A/B tests have long been the go-to method for fine-tuning websites and improving performance metrics, the future of online experiments offers a broader horizon. This article explores the paradigm shift shaping the trajectory of experimentation beyond basic A/B tests.
One key aspect revolutionizing online experiments is the increasing popularity of multivariate testing (MVT). Unlike A/B tests that only compare two variants of a web page or element, MVT allows for multiple variants to be tested simultaneously. This enables businesses to gain deeper insights into user behavior by assessing the individual impact of various combinations across different elements of their online properties.
Moreover, as machine learning algorithms continue to advance, predictive modeling is becoming central to online experimentation. These models can analyze vast amounts of user data and render predictions on future user behavior and preferences, allowing businesses to prioritize variants with higher potential for success while also reducing the time and resources needed for experiments.
Another critical trend influencing the future of online experiments is personalization. With user demands becoming increasingly diverse, tailoring experiences to individuals has become imperative. Advanced experimentation techniques are now emerging that enable businesses to experiment with personalized content for different user segments. By measuring the impact of these tailored experiences, companies can improve engagement, retention, and ultimately, conversions.
Parallelly, the rise of automation tools empowers organizations to conduct experiments at scale more efficiently. Automated experimentation platforms streamline the iterative process of designing experiments, running them across various customer touchpoints, and processing results swiftly. These tools not only alleviate human effort but also reduce potential biases in experiment setup.
Furthermore, as mobile devices continue to dominate Internet usage globally, adapting experimentation approaches for mobile platforms will play an integral role in driving successful digital strategies. Mobile-centered experimentation encompasses app-specific optimizations and tailoring experiences specifically towards mobile users' behaviors, creating limitless possibilities for innovations in online experiments.
Finally, ethical considerations are poised to play an increasingly prominent role in the future of online experiments. With increasing awareness about data privacy and ethical experimentation, businesses must ensure that experiments adhere to guidelines and safeguards that protect user interests. Ethical experimentation practices will not only bolster the credibility and trustworthiness of organizations but also reinforce positive user experiences.
In a nutshell, the future of online experiments extends beyond basic A/B tests, integrating advanced methodologies, automation, personalization, mobile-centric strategies, predictive modeling, and ethical considerations. As organizations strive to maximize their digital performance while valuing user satisfaction, embracing these evolutions will be crucial to staying at the forefront of progressive digital experiences.
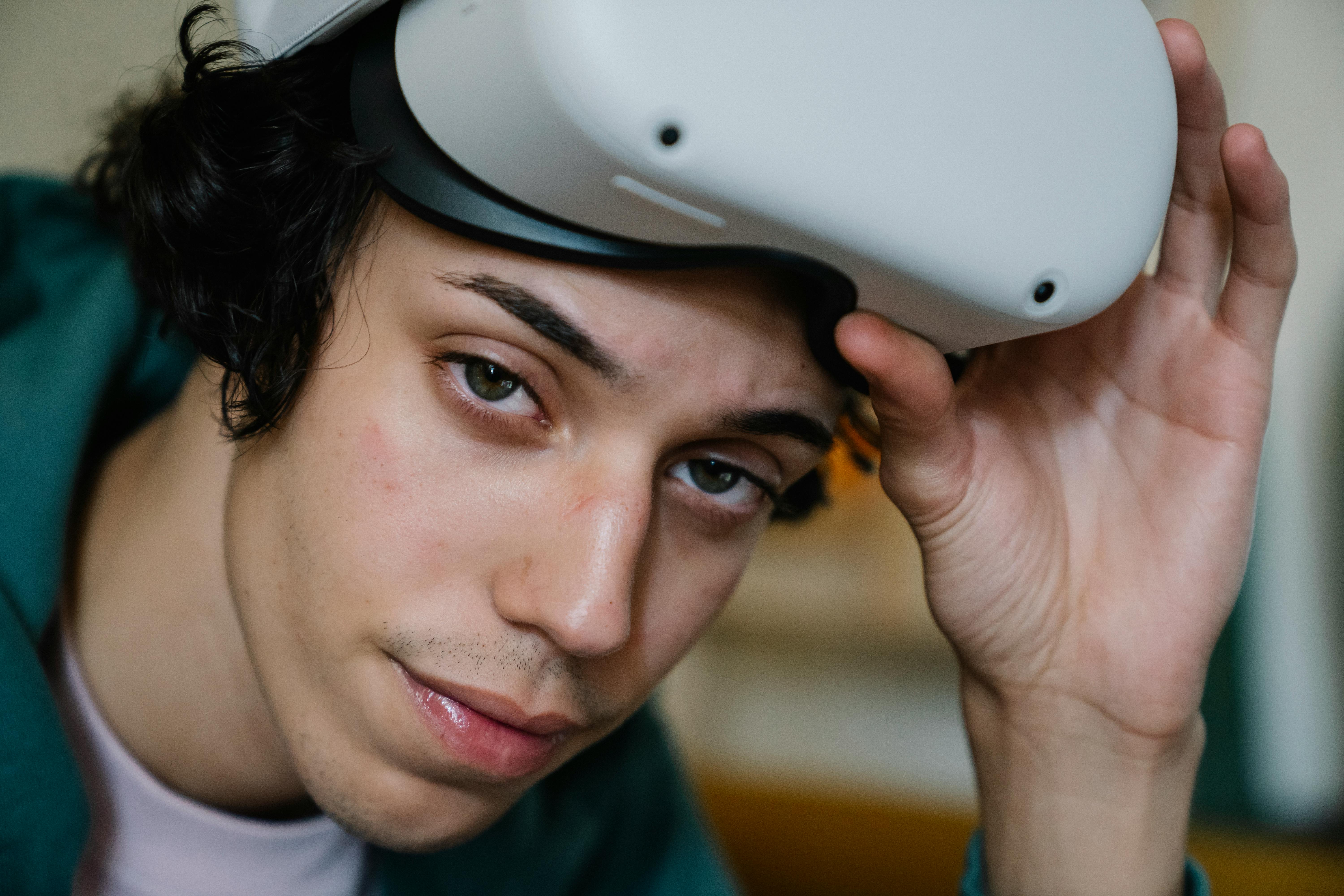
A/B testing has become an integral component of the modern digital landscape as businesses seek to optimize their websites or applications. It involves presenting users with different versions of a webpage or feature to determine which one performs better. However, conducting A/B tests raises various ethical considerations that should not be overlooked. In particular, safeguarding user privacy and establishing trust are critical aspects for any organization involved in this practice.
User privacy is of utmost importance when implementing A/B tests. It is vital to ensure that sensitive user information, such as personal details or browsing habits, remains confidential throughout the testing process. Taking measures to anonymize data by stripping away personally identifiable information helps protect individuals' privacy rights and prevent potential misuse of the collected data.
Moreover, transparency plays a central role in upholding ethical standards in A/B testing. Organizations must clearly communicate to users that they are participating in an experiment and outline what data is being collected. Providing detailed information on how the data will be used or if third parties will have access ensures users can make informed decisions about their participation.
Seeking explicit informed consent from users is another ethical consideration necessitating attention. Users should be provided with the option to opt-in or opt-out of participating in an A/B test, without facing repercussions no matter their choice. This empowers individuals to maintain control over their own data and allows them to shape their online experiences according to their preferences.
When analyzing results from A/B testing, it's crucial to rely on aggregate data rather than focusing on individual user behavior. Aggregated data protects the anonymity of participants, preventing potential harm or discrimination resulting from targeting specific individuals based on their actions during the test. This practice reassures users that their privacy rights are respected while seeking to derive meaningful insights for improving the tested aspects.
Lastly, building trust between organizations and users is foundational in ethical A/B testing. Openly sharing the purpose and benefits of conducting these experiments fosters trust and credibility. Being transparent about intentions, outlining the overall goals, and ensuring the collected data is used solely for improving user experiences further strengthens this bond. Organizations must also demonstrate adherence to legal regulations, such as data protection laws or standards like GDPR, to instill confidence in users.
In conclusion, ethical considerations are paramount in A/B testing to protect user privacy and ensure trust. Organizations should implement measures to safeguard user information, apply transparency throughout the process, seek informed consent, use aggregated data for analysis, and cultivate a trustworthy relationship with their users. By treating individuals' privacy and trust with utmost importance, businesses can conduct A/B tests responsibly while optimizing user experiences in an ethical manner.Personalization Through A/B Testing: Tailoring User Experiences for Better EngagementPersonalization Through A/B testing: Tailoring User Experiences for Better Engagement
Personalization has become a significant aspect of effective digital marketing and user experience strategies. By tailoring user experiences to meet individual needs and preferences, businesses can enhance engagement and ultimately drive better results. One popular way to achieve this is through A/B testing.
A/B testing is a powerful technique that allows marketers to compare two or more variations of a webpage, email, app, or any other type of digital experience in real-time. By dividing users into different groups and presenting them with distinct versions, businesses can gather valuable data on what elements and experiences resonate the most with their audience. This data-driven approach helps in making informed decisions and enhancing user engagement effectively.
There are various elements businesses can personalize through A/B testing. Starting with something as basic as headline copy, companies can test variations to gauge which one generates higher click-through rates or engagement. Similarly, different visual designs can be tested to identify the most impactful visuals that lead to increased conversions.
Apart from these visual elements, personalizing calls-to-action (CTAs), button placements, and even navigation options can significantly affect user experience. For instance, changing the text or color of a CTA button can influence click-through rates and conversion rates. Navigation options can also be modified by providing personalized menus or site maps based on a user's previous interactions with a website.
Tailoring content is another key area for personalization. Businesses can conduct A/B tests to determine the impact of different types of content — short versus long-form blog posts or videos versus infographics. Personalized content allows businesses to cater to users' individual needs, boosting engagement and making it more likely for users to stay on their site longer.
To utilize A/B testing for better personalization successfully, it is important to define clear goals and metrics beforehand. Without clear objectives in mind, it becomes challenging to gauge the effectiveness of variations accurately. Metrics such as click-through rates, bounce rates, time on page, and conversion rates can help in measuring the success of personalized experiences.
It is essential to highlight that A/B testing should not be a one-time activity; instead, it should be an ongoing process. Businesses should continuously test new ideas and variations to iteratively improve user engagement. By analyzing collected data and iterating on initial results, companies can steadily optimize their personalization strategies to deliver highly engaging user experiences.
In conclusion, personalization through A/B testing is a vital strategy for businesses aiming to maximize user engagement and drive better results. By continuously experimenting with various elements and content choices, marketers can create tailored experiences that resonate with individual users and lead to higher conversions. Effectively leveraging A/B testing allows businesses to stay ahead of the competition and provide experiences that truly connect with their audience.
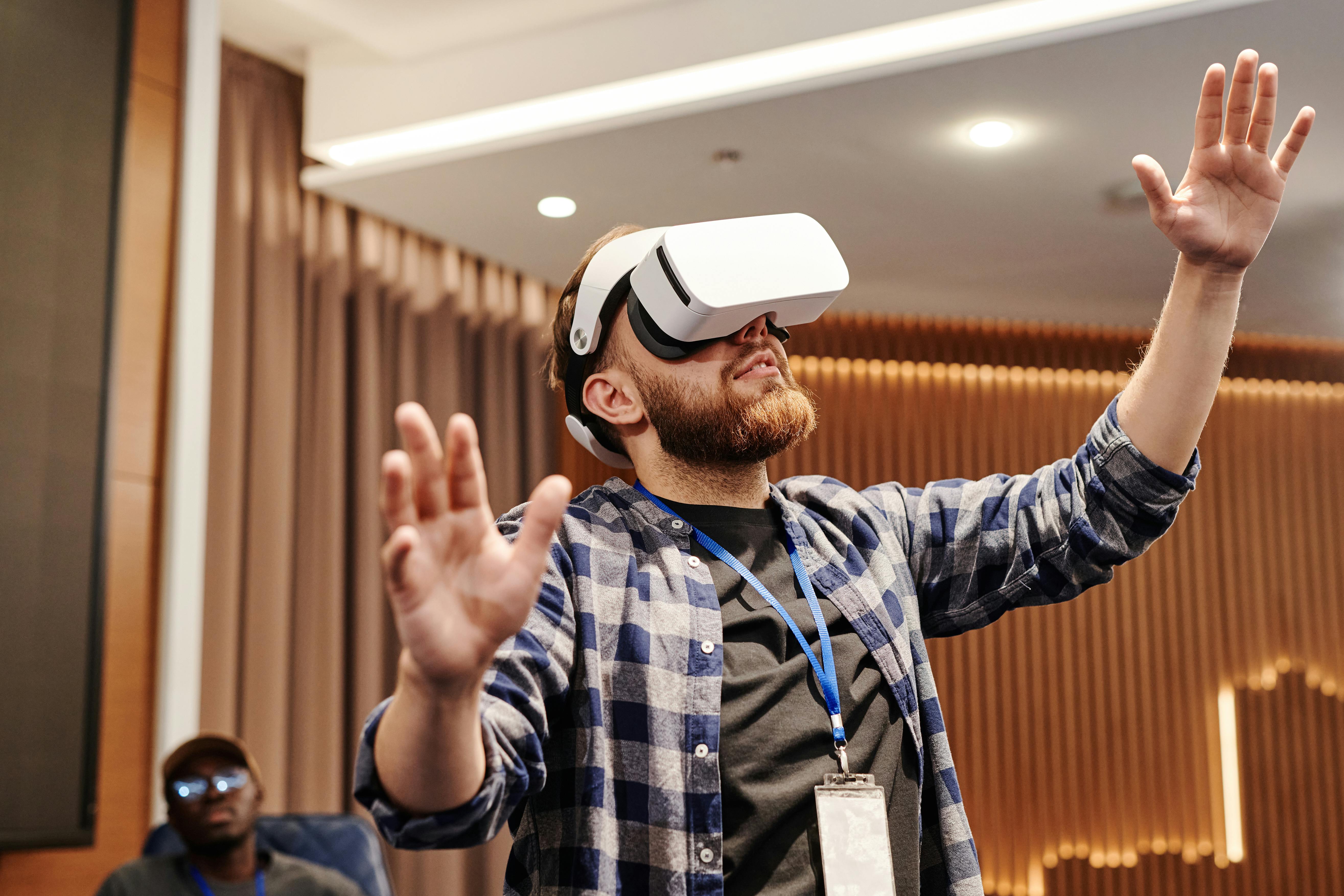
When it comes to experimentation, AI and ML can significantly enhance A/B testing through smart data analysis. These technologies excel at processing enormous amounts of data, allowing robust analysis of user behavior, conversion rates, click-through rates, and other critical metrics collected during A/B tests. With sophisticated algorithms acquiring patterns from the data, organizations gain a deeper understanding of user preferences and behavior.
Targeted audience segmentation is another area where AI and ML can leverage A/B testing. By employing clustering algorithms on user data, organizations can segment users based on various demographic and behavioral criteria. This leads to refined A/B test group compositions, ensuring that experiments reveal more efficient results as they are tailored to specific user segments.
Personalization is a powerful technique made possible by AI & ML that amplifies A/B testing efforts. Personalizing content or experiences based on individual preferences identified through predictive models enhances engagement and boosts conversion rates. By implementing personalized A/B tests shaped by machine learning-based personalization models, organizations can evaluate the effectiveness of their tailored experiences.
Real-time automated decision-making during A/B testing can be enhanced with the help of AI and ML technology. Advanced algorithms can assess ongoing test results dynamically, continuously analyzing evolving data patterns and making efficient decisions based on defined criteria. In this way, organizations can reduce the time required to reveal statistically significant results and make informed decisions promptly during live A/B tests.
Furthermore, AI-powered automation software assists in managing and executing simultaneous multivariate testing efficiently. It allows testers to run multiple experiments simultaneously while dynamically adjusting traffic allocation based on observed conversions. This synergy allows for rigorous testing of numerous combinations across multiple dimensions with reduced testing periods.
Combining AI and ML with A/B testing can aid in preemptive identifying of drop-offs or failures within the experiments. By continuously monitoring multiple variables and parameters, organizations can be alerted to anomalous trends, enabling prompt corrective actions. As a result, A/B testing efforts become more efficient, iterative, and adaptable to ever-changing user behaviors.
In conclusion, leveraging AI and ML technologies enriches A/B testing capabilities, enabling organizations to extract meaningful insights from vast sets of data. These advancements facilitate precise audience segmentation, personalization, real-time decision-making, and management of multivariate simultaneous tests. The fusion of AI, ML, and A/B testing ultimately leads to a more streamlined and optimized experimental process, helping organizations enhance their products or services based on empirical results.The Psychological Aspects Behind User Responses in A/B TestsWhen conducting A/B tests, it is important to consider the psychological aspects that influence user responses. User behavior and decision making can vary considerably, and understanding these factors will enable you to obtain more accurate data and insights. Here are some key psychological aspects to take into account:
1. Anchoring effect: Users tend to rely heavily on the first piece of information they come across. In A/B testing, this means that the version presented first may have a disproportionally large influence on users' judgments and responses.
2. Confirmation bias: Users have a natural inclination to seek out information or experiences that confirm their existing beliefs or preconceptions. They may be more likely to respond positively to a version that aligns with their expectations or biases, leading to biased results.
3. Cognitive load: Users have limited cognitive resources for evaluating options and making decisions. Therefore, if an A/B test introduces additional complexity, such as multiple variations or intricate design elements, it may overwhelm users and impact their decision-making process.
4. Social validation: People tend to conform to the behaviors or opinions of others they perceive as similar, especially in situations where they lack confidence or feel uncertain. Demonstrating popularity or positive social proof for a version can significantly influence user responses in A/B tests.
5. Loss aversion: Individuals place greater importance on avoiding losses compared to achieving similar gains. Test variations that are perceived as causing a loss or negative change may generate stronger negative reactions from users compared to other versions.
6. Decision framing: How information is presented can influence how users perceive and respond to different options in an A/B test. Framing the value proposition differently, emphasizing potential benefits, utilizing urgency, or presenting options in terms of relative advantage can impact user responses.
7. Priming effects: The order or context of information can "prime" users' subconscious minds, influencing their subsequent perceptions and behaviors. For example, a prior visual concept or language can subtly shape users' responses to a subsequent A/B test variation.
8. Habit formation: Users develop ingrained habits from repeated experiences and familiarity. Introducing change through an A/B test may disrupt user habits, leading to resistance or negative reactions to the variation.
9. Paradox of choice: Offering too many options can lead to decision paralysis or reduced satisfaction with the chosen option. Limiting the number of variations in an A/B test simplifies decision-making for users and improves the quality of responses.
10. Emotional triggers: Emotions play an integral role in decision making. Design elements, color schemes, images, or text in an A/B test may evoke different emotional responses in users, impacting their overall preference for a specific variation.
Understanding these psychological aspects is fundamental for designing effective A/B tests and interpreting the results accurately. Considering how these factors influence user responses will allow you to develop better strategies and optimizations for your website or product based on robust insights gained from successful A/B tests.