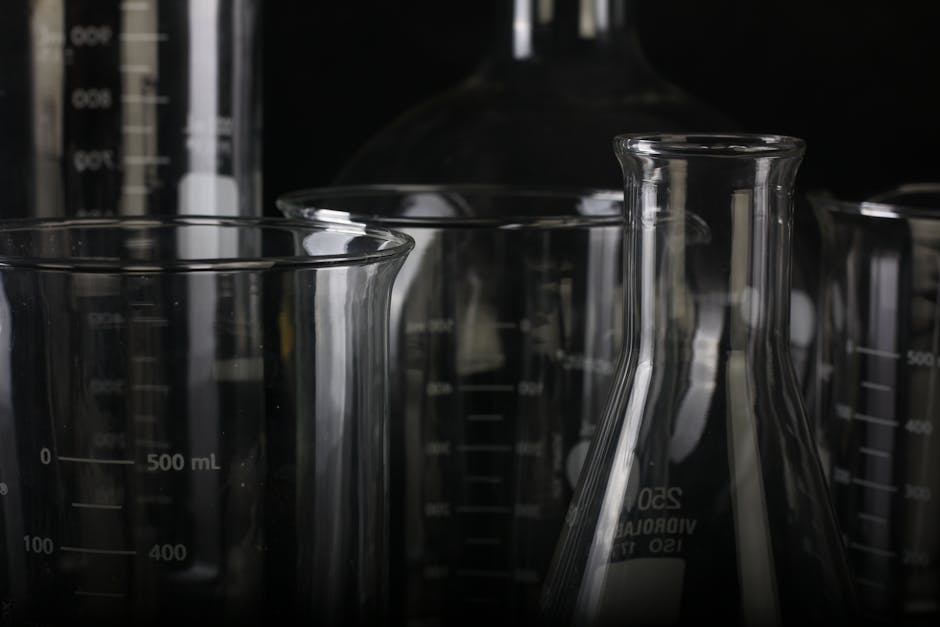
The Power of A/B Testing: Unleashing the Benefits and Weighing the Pros and Cons
A/B testing is an essential tool used in various fields to make informed decisions based on empirical evidence. It involves testing two different versions, namely Version A and Version B, of a particular element to determine which performs better. This method helps identify the impact of changes and allows for data-driven decision making.
The process of an A/B test begins with defining a clear hypothesis or research question. For example, a website owner may wonder if changing the color of a call-to-action button will increase conversion rates. The hypothesis is then divided into two versions: the original (Version A) and the change (Version B).
To ensure a reliable test, it's crucial to keep all other factors consistent between both versions except for the element being tested. This way, any differences observed can be attributed directly to the changes made. Randomizing which version is shown to participants further minimizes bias and increases the validity of results.
The test is typically conducted by splitting participants into two groups: one exposed to Version A and the other exposed to Version B. Data is collected regarding user behavior, such as click-through rates, conversions, or metrics related to user experience. Statistical analysis is then performed on this data to determine if there is a significant difference between Version A and Version B.
Interpreting the results correctly is essential for effective decision making. Invalidation of the null hypothesis (no significant difference between versions) indicates that one version outperforms the other. However, statistical significance alone should not be the sole basis for decision making. It's vital to consider both practical significance and business goals to draw meaningful conclusions.
A/B testing holds immense importance in decision making because it eliminates guesswork and intuitions by relying on objective data. It enables businesses, marketers, and researchers to optimize their products, services, or strategies based on evidence-backed insights rather than assumptions. By eliminating subjective bias, biases, and guesswork, organizations can better cater to user preferences, increase customer satisfaction, and drive desired actions effectively.
Moreover, A/B testing allows for iterative improvement. By constantly refining the tested elements based on results, one can fine-tune performance over time. It provides an opportunity to iron out inefficiencies continuously and adapt strategies accordingly.
A successful A/B testing strategy includes setting clear goals, analyzing relevant metrics, and properly implementing changes based on test outcomes. Careful planning, carrying out controlled experiments, and objectively evaluating the results are imperative for meaningful decision making through A/B testing.
In conclusion, A/B testing is a powerful tool that enables data-driven decision making by objectively comparing different versions of a particular element. Its importance lies in eliminating assumptions, reducing biases, and allowing for iterative improvements. By leveraging A/B testing insights successfully, businesses and individuals can make informed decisions that lead to enhanced user experiences and desired outcomes.
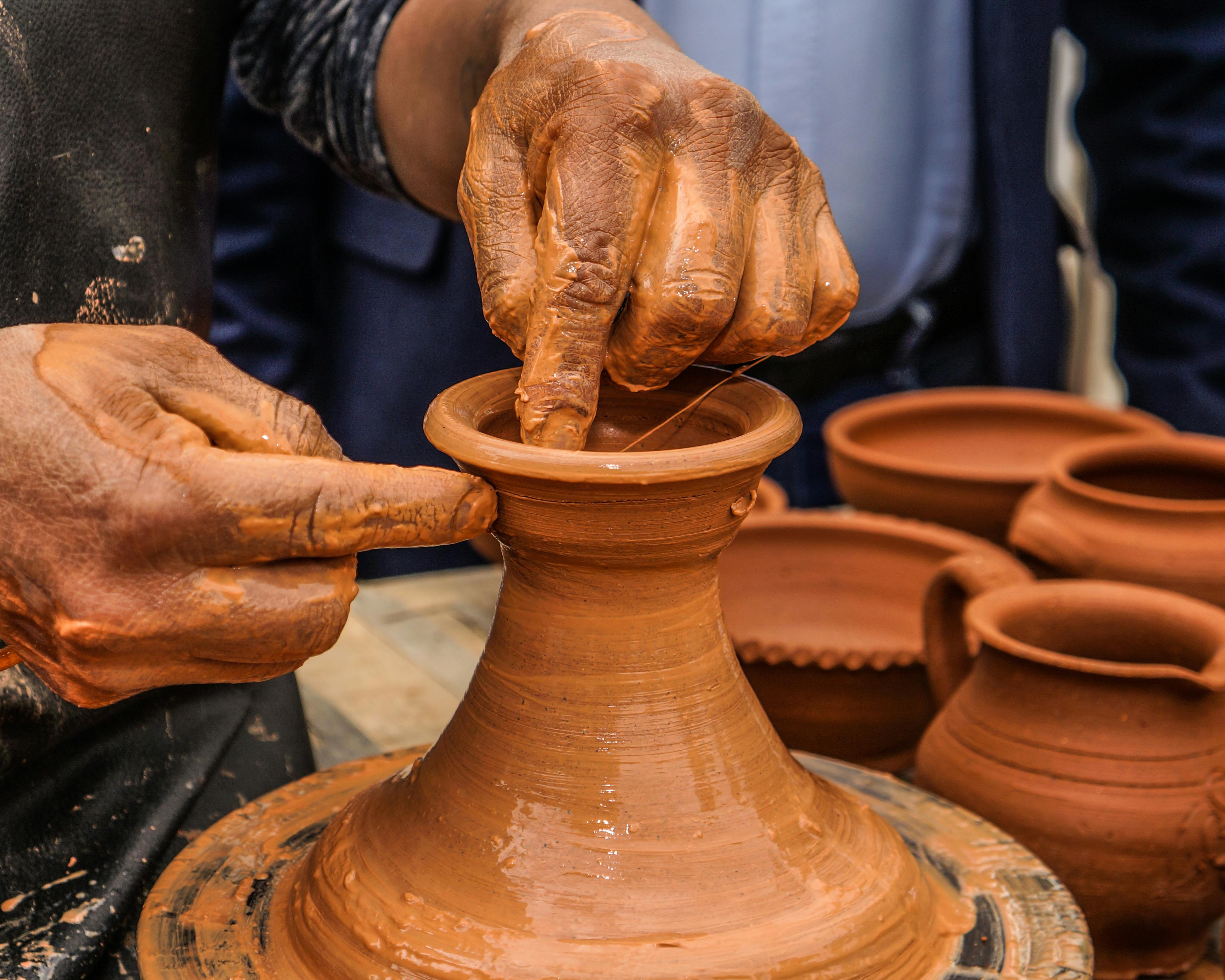
One of the key benefits of A/B testing is its ability to eliminate guesswork. It allows product teams to test different variations simultaneously and collect data on user behavior, preferences, and responses. When done right, this type of testing serves as an objective tool for analyzing the impact of changes on user experience and overall performance.
A/B testing generates crucial insights into user behavior and preferences, thereby guiding innovation initiatives. By splitting users into different groups randomly, the test provides statistically meaningful data on how various features or designs perform. This enables companies to make well-informed decisions about optimizing their products based on real user feedback rather than assumptions.
Moreover, A/B testing empowers product development teams to experiment with different ideas and innovations more rapidly. Traditional development cycles often involve significant time investments, resource allocation, and risks. However, with A/B testing, small tweaks or big changes can be quickly evaluated through experimentation without disrupting the entire product. It offers an iterative approach where ideas can be tested and validated sequentially or simultaneously.
By enabling continuous measurement of user response, A/B testing allows for fine-tuning and optimization at each step of the product development cycle. This iterative process encourages experimentation by giving teams more confidence in validating ideas before committing resources to full-scale implementation.
Furthermore, A/B testing encourages a culture of data-driven decision making within organizations. Instead of relying solely on intuition or opinion-based decisions, companies can leverage quantitative data generated through rigorous testing. This shift promotes evidence-based conversations and collaborative discussions across multiple stakeholders involved in product development.
A/B testing also helps minimize the risk associated with introducing new features or changes to a product. Instead of making substantial investments based on assumptions, businesses can take an incremental approach by testing new ideas with a small subset of users first. This reduces the likelihood of costly mistakes and allows teams to validate concepts before scaling them up.
Successful A/B testing fosters innovation by providing continuous feedback loops that inform product development. The insights gained through these tests can reveal potential areas for improvement, highlight unexplored opportunities, or even identify new customer segments. Such actionable data reinforces a proactive approach towards innovation, enabling teams to evolve their products effectively over time.
In conclusion, A/B testing fuels innovation in product development by eliminating guesswork, providing rapid feedback loops, promoting data-driven decision-making, and minimizing risks associated with change. It serves as a powerful tool that drives continuous improvement and optimization based on objective user feedback. Ultimately, by leveraging the insights gained from A/B testing, businesses can foster innovation and deliver products that cater to user needs while remaining competitive in the market.
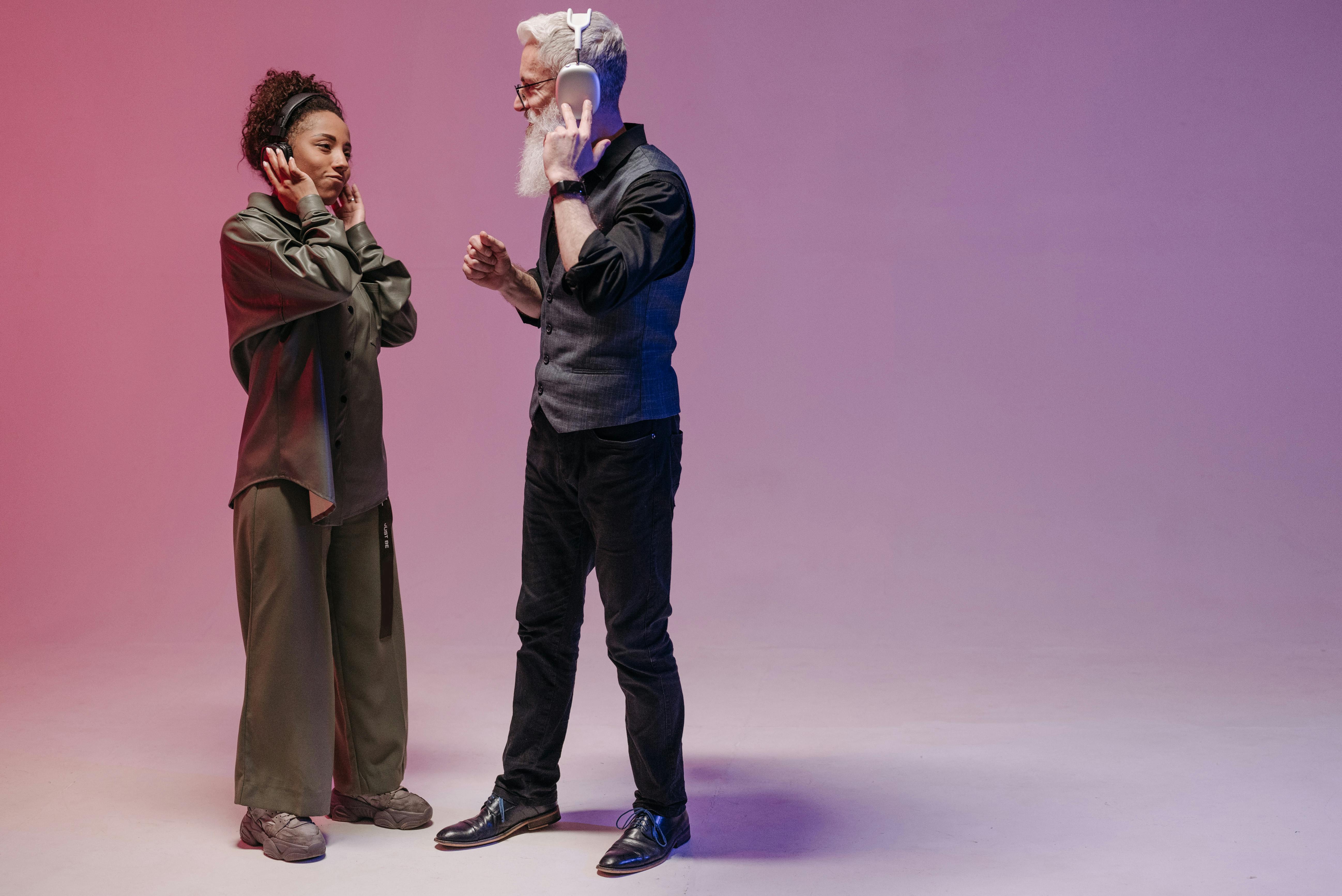
Pros:
- Improved Conversion Rates: One of the significant advantages of A/B testing is its potential to enhance conversion rates. By testing different variations of an element, such as a webpage layout or a call-to-action button, marketers can identify which version generates more conversions. This data-driven approach enables the refinement of marketing materials for optimal results.
- Enhanced Customer Engagement: With A/B testing, businesses can experiment with various content formats and presentation styles to determine which ones elicit higher levels of customer engagement. This enables brands to deliver content that resonates better with their target audience and fosters long-lasting relationships.
- Better Decision Making: A/B testing provides access to actionable insights that inform decision-making processes. It allows marketers to make data-backed decisions, enabling them to minimize the risks associated with launching unsuccessful campaigns or initiatives.
- Cost Efficiency: Implementing A/B tests in your digital marketing strategies can contribute to cost reduction. By gaining insights into what works best for consumers without extensively investing in unproven ideas, companies can prioritize their budgets and allocate resources efficiently.
Cons:
- Reliability on Sample Size: One of the limitations of A/B testing relates to sample size requirements. In order to obtain statistically significant results, a sizable sample needs to be tested. Smaller businesses or niche markets might struggle with achieving a sufficiently large enough audience or traffic to obtain reliable insights.
- Time Constraints: Conducting A/B tests requires sufficient time frame to collect and analyze data accurately. While larger companies often have the resources and patience for running such tests over extended periods, smaller businesses operating with tight timelines might find the prolonged testing period a challenge.
- Difficulty in Test Isolation: To attribute changes in results accurately, it is crucial to isolate variables being tested. However, in complex digital marketing strategies involving multiple interlinked elements, ensuring pure isolation becomes challenging. This may blur the effectiveness of A/B testing as the impact might not only stem from the variable tested but also from other connected factors.
- Potential for Biased Interpretations: Human bias in analyzing test results can influence decision-making processes. It is essential to approach A/B testing with an open mindset and consider how personal preferences or professional biases can sway interpretation, potentially translating into flawed decision-making.
In conclusion, A/B testing offers beneficial capabilities within digital marketing strategies. While it allows businesses to enhance conversion rates, engage customers effectively, and inform decision-making with data-driven insights, it is important to acknowledge its limitations. These include a reliance on sample size for reliable results, time constraints for smaller businesses, challenges in isolating variables, and the potential for biased interpretations. By considering both the pros and cons critically, marketers can leverage A/B testing effectively to drive success in their digital marketing endeavors.Case Studies: Successful Outcomes Powered by A/B TestingCase studies are a powerful way to showcase successful outcomes that stem from A/B testing. A/B testing is a method commonly used in marketing and product development to compare two versions of a webpage, email campaign, or any other digital asset to determine which one performs better.
One remarkable example of an A/B test leading to successful outcomes can be seen in an e-commerce website. The company aimed to increase the click-through rate (CTR) on their product landing page. Through careful planning, they created two versions: the control version, which retained their original design and layout, and the variation version, which introduced some modifications.
Over a specific period, the company redirected half of the website traffic randomly to each version, analyzing user behavior, engagement, and conversion metrics carefully. By doing so, they were able to accurately measure the impact of each variation on visitor behavior.
After trending analysis, it was discovered that the variation version significantly outperformed the control version in terms of CTR. This key insight prompted the company to implement the changes made in the variation version permanently throughout their website. As a result, click-through rates soared by 30%, leading to an increase in sales and revenue.
Another interesting case study involves an online booking platform that wanted to improve their conversion rates. They focused on their page layout and tried out different variations involving button placement and color schemes. Thanks to A/B testing, they could understand how these small modifications influenced user interactions and ultimately conversions.
Carefully monitoring user engagement metrics for both versions, they noticed that one particular variation attracted much higher visit duration and reduced bounce rates compared to others. Based on confirmed results from statistical significance testing, they decided to implement this winning variation across their platform.
As a direct result of this change powered by A/B testing, the platform experienced a significant boost in conversion rates, with users being more inclined to complete bookings due to the optimized design. This case study serves as a testament to the incredible impact A/B testing can have on improving user experiences and driving business outcomes.
In conclusion, case studies showcasing successful outcomes through A/B testing play a crucial role in validating the effectiveness of this methodology. By comparing different variations systematically and fully assessing the generated data, organizations can make informed decisions that optimize their digital assets, enhance user experiences, and drive positive financial results.
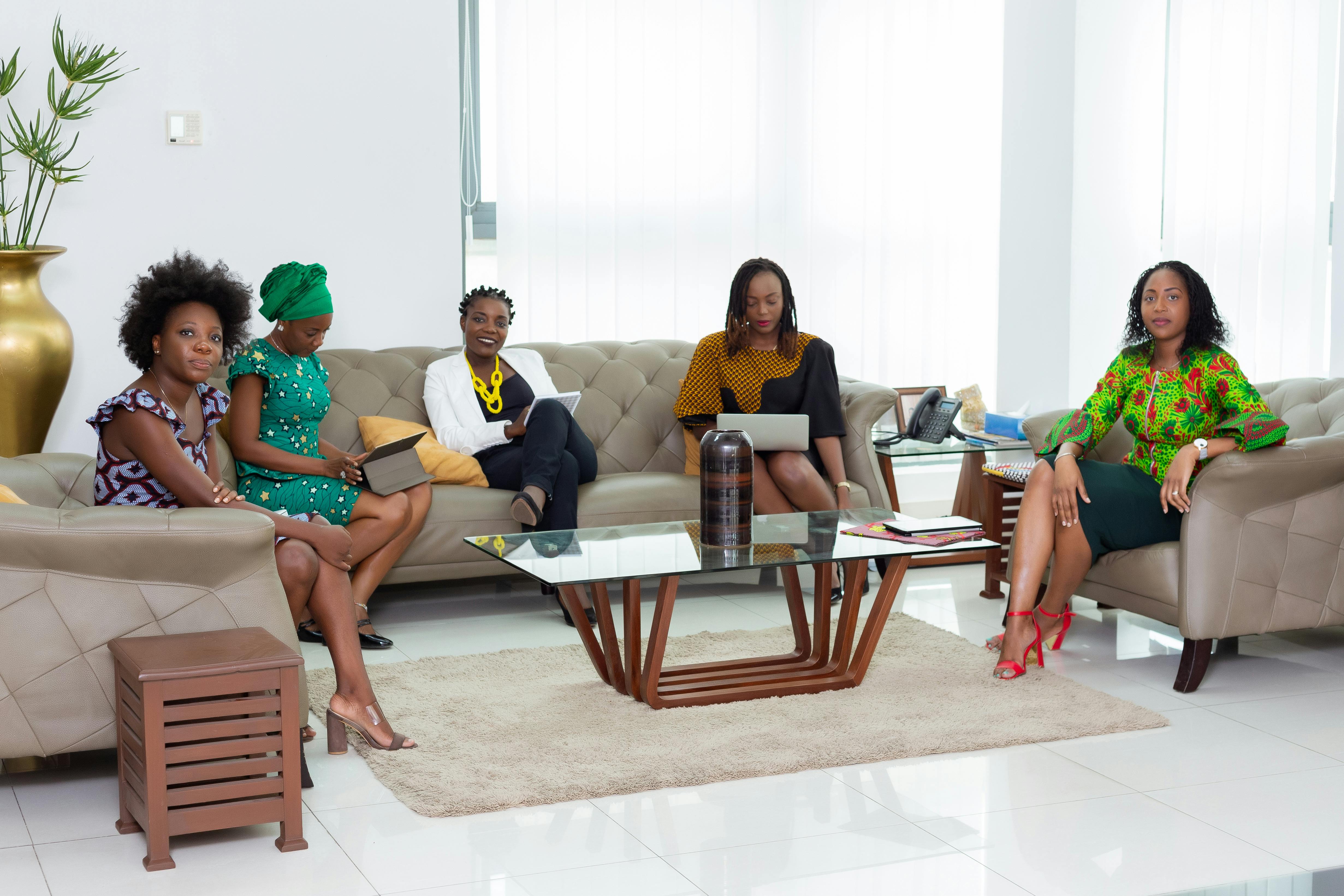
One crucial aspect of A/B testing is statistical significance, which measures the reliability and credibility of the test results. Statistical significance is vital because it helps researchers and analysts make confident and accurate decisions based on the data collected during the A/B testing process.
At its core, statistical significance indicates whether an observed difference between two versions (say A and B) can be attributed to the variations itself rather than random chance or fluctuation. In other words, it helps identify if the difference likely represents a reliable effect or just happened randomly by chance. The statistical significance level usually set at 95% confidence level imparts a high degree of certainty or confidence that the observed difference is indeed significant.
To determine statistical significance, analysts use mathematical techniques such as hypothesis testing and p-values. Hypothesis testing enables researchers to evaluate two mutually exclusive hypotheses: the null hypothesis (effect due to chance) and the alternative hypothesis (effect due to actual variation). By examining the sample data collected from the A/B test, statisticians evaluate if the null hypothesis should be rejected based on predefined criteria like p-value thresholds.
A p-value conveys the probability that an observed result could have occurred by random chance alone under the assumption that there is no true difference between versions A and B. If the calculated p-value falls below a predetermined threshold (typically 0.05), it suggests that the observed difference is statistically significant, supporting rejection of the null hypothesis. On the contrary, if p-value exceeds this threshold, it implies that any differences observed are likely due to random chance alone rather than actual variation.
Importantly, achieving statistical significance does not automatically mean practical or meaningful significance. Statistical significance ensures confidence in the findings' accuracy but shouldn't be regarded as the sole indicator of importance. The magnitude or size of the effect observed should also be considered alongside statistical significance. Sometimes, relatively small differences could be statistically significant due to large sample sizes, but might have marginal practical significance in real-world scenarios.
Understanding and emphasizing statistical significance in A/B testing helps businesses and researchers make informed decisions based on strong evidence. By ensuring that test results are not due to random chance, it empowers them to confidently implement changes, launch better-performing versions, and ultimately optimize their strategies. As such, statistical significance plays a pivotal role in the scientific process of A/B testing and focuses on translating data into actionable insights.Common Pitfalls in A/B Testing and How to Avoid ThemA/B testing, often referred to as split testing, is a powerful tool utilized by businesses to evaluate different versions of a webpage or feature to determine which one generates better results. However, there are several common pitfalls that can undermine the effectiveness and accuracy of A/B tests if not properly addressed. By being aware of these pitfalls and implementing strategies to avoid them, organizations can ensure more reliable and actionable test outcomes. Let's delve into some of these common pitfalls:
1. Insufficient sample size: Conducting A/B tests with a small sample size can lead to inconclusive or unreliable results. It is crucial to have an adequately sized sample in order to observe statistically significant differences between variants and make informed decisions.
2. Running tests for insufficient duration: Test results should be collected over a meaningful period of time to factor in temporal variations and give the experiment enough exposure. Terminating tests prematurely might skew the results and lead to inaccurate conclusions.
3. Multiple comparisons problem: When performing multiple tests simultaneously, the likelihood of witnessing false positives increases. Maintaining a control group and applying statistical correction techniques like the Bonferroni adjustment helps account for the multiple comparisons problem.
4. Biased visitors selection: The selection of participants for A/B tests must be conducted randomly or using informed techniques to avoid introducing any bias that may distort the results. Biased sample populations can lead to misguided conclusions.
5. Hopping on “winnersâ€: Choosing a variant prematurely just because it appears to have won during a short test interval is ill-advised. It is important to validate longer-term effects as behavioral patterns may not stabilize immediately, potentially resulting in inaccurate winner identification.
6. Ignoring external factors: Failing to account for confounding factors or external variables that may influence test results can diminish their validity. Unforeseen circumstances such as seasonal variations or marketing campaigns need contextual analysis within an A/B testing framework.
7. Neglecting business goals: A/B testing should always be aligned with the organization's goals and objectives. Prioritizing metrics that are not genuinely aligned with these goals can lead to inconclusive or suboptimal test outcomes.
8. Misinterpreting statistical significance: Understanding statistical significance is fundamental in A/B testing. It is crucial to comprehend what p-values signify, which hypothesis you're evaluating, and interpret confidence intervals correctly to draw valid conclusions.
9. Not monitoring after implementation: Implementing changes based on an A/B test's results and then failing to monitor future performance can undermine the efforts that went into experimentation. Tracking the real-world impact of the modification ensures continued success and validates the initial findings.
By avoiding these common pitfalls, organizations can conduct more reliable A/B tests and extract meaningful insights that facilitate data-driven decision-making. Testers need to exercise caution, employ appropriate statistical tools and methodologies, and ensure tests are conducted with robust research principles, making A/B testing a valuable asset for continuous improvement and growth.
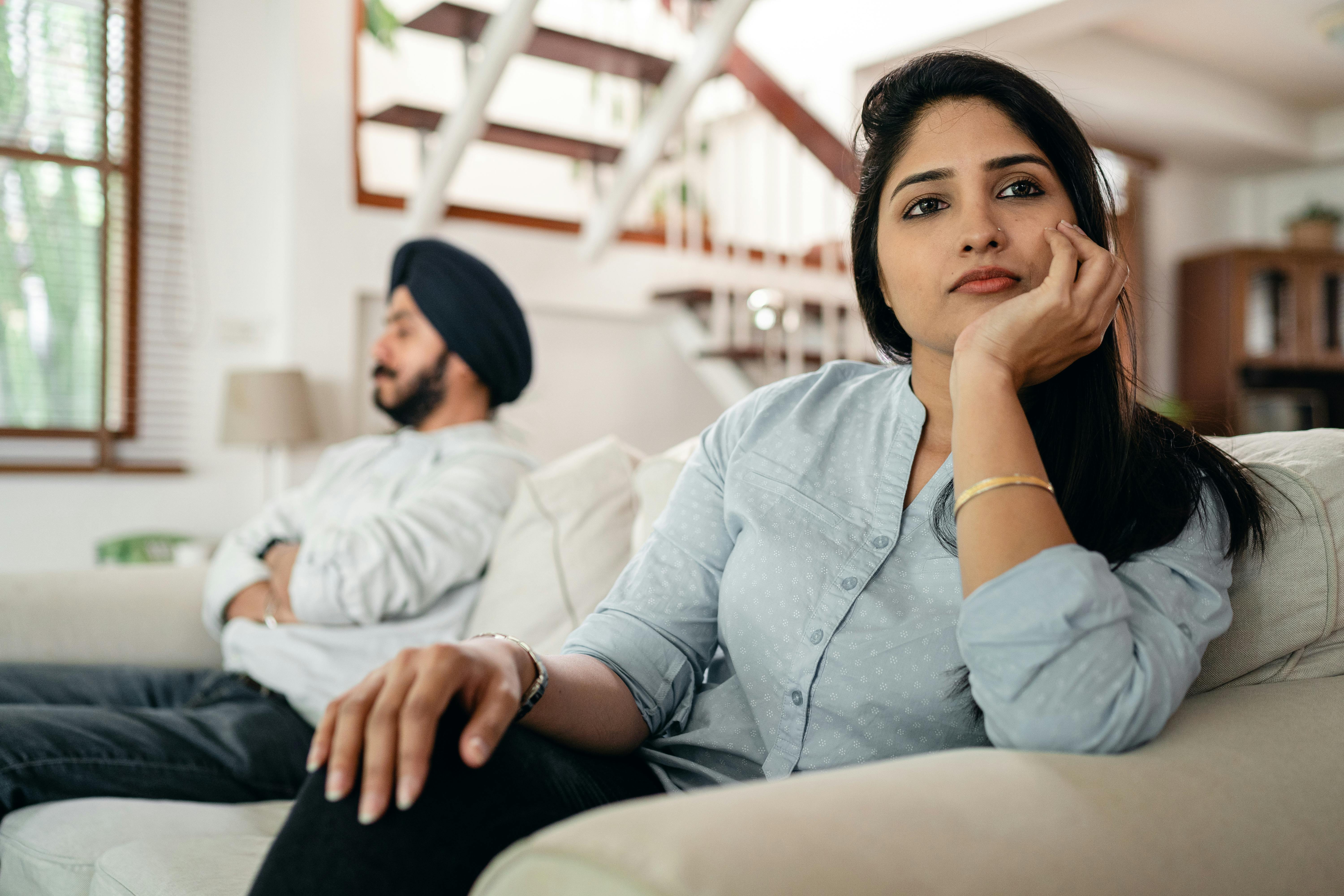
When it comes to testing various design elements and features using the A/B testing method, there are essential ethical considerations that must be taken into account, primarily centered around the impact on users. A balanced focus on achieving business goals and ensuring users' well-being is crucial.
Firstly, privacy concerns arise while conducting A/B tests on users. Collecting and analyzing data to improve user experience can potentially involve observing users' behavior, which can intrude upon their privacy rights. Therefore, it is important to be transparent about such data collection practices, obtain proper consent from participants, and to anonymize and protect their personal information throughout the entire testing process.
Another crucial aspect that must be considered is the potential for harm or negative effects on users during A/B testing. Altering a user's experience without their knowledge or consent can lead to confusion, frustration, or even turn them away from using a product or service. This calls for carefully thought-out testing procedures that minimize harm and prioritize user well-being throughout.
A/B testing should also avoid exploiting user biases or vulnerabilities. Exploitative practices such as nudging or manipulating users into specific actions for business gains undermine their autonomy and can create an ethical dilemma. Valid consent needs to be obtained, ensuring that participants have full disclosure and awareness of any test interventions applied during the process.
Testers must also consider the long-term effects of A/B testing in terms of user trust and overall credibility of the product or service. Misuse of data, misleading experiments, or repetitive tests solely focused on generating revenue could erode trust between companies and their users. Maintaining transparency and open communication about the purpose and outcomes of A/B tests is vital in maintaining that trust.
Lastly, it is necessary to remain mindful of any potential biases that may arise during A/B testing. Bias could emerge from sample selection, test design, or interpretation of results. It is important to approach testing with a commitment to equal representation, avoiding discrimination or exclusion of any user groups in the testing process. An inclusive and fair testing environment should be fostered by taking necessary precautions.
In conclusion, the ethical considerations surrounding A/B testing encompass several vital aspects. Respecting privacy, ensuring transparency, minimizing harm, avoiding exploitation, maintaining user trust, and eliminating bias are paramount when conducting A/B tests on users. Adhering to these considerations guarantees a responsible testing approach that centers around both business goals and the well-being of the users involved.A/B Testing Tools: An Overview of What’s Available and How to ChooseA/B testing tools are an invaluable asset for businesses looking to optimize their websites, marketing campaigns, and user experiences. These tools enable you to test different variations of a webpage or digital asset, such as headlines, images, layouts, buttons, colors, and more, in order to identify the most effective version that can ultimately enhance conversions, user engagement, and overall performance.
When selecting an A/B testing tool, it's important to consider a few key factors. First and foremost, you should evaluate the features offered by each tool. Some popular options include Visual Editors that allow you to make changes without coding knowledge, Goal Tracking which enables you to define specific objectives for your tests, and Statistical Engines that help analyze the results accurately.
Another crucial aspect is integration compatibility – ensure that the tool can seamlessly integrate with your existing website or content management system (CMS). This will make implementation much smoother and streamline the entire A/B testing process.
Consider the scalability of your chosen tool as well. As your business grows, you would ideally want a solution that can handle increased traffic and users without affecting performance.
Moreover, examine the reporting and analytics capabilities of the tool. The way your data is presented should be easy to comprehend and visually appealing. Robust reporting allows you to draw accurate conclusions from your experiments easily.
Don't forget about support. Before finalizing any decision, look into the available support options provided by the A/B testing tool. Responsive and knowledgeable customer support can be vital when encountering technical issues or needing guidance in fully utilizing the functionalities of the tool.
Lastly, keep pricing in mind. Different tools offer various pricing models – some may have flat-rate plans while others base their pricing on factors like visitor volume or features utilized. Additionally, check if they provide any free trials or demos to evaluate whether the tool meets your expectations before committing financially.
When selecting an A/B testing tool suitable for your requirements, rightly weighing these considerations will ensure that you make an informed decision and find a tool that aligns with your business goals.
Remember, deciding on an A/B testing tool can be crucial for optimizing your digital presence efficiently. Opt for one that offers robust features, seamless integration, scalability, comprehensive analytics, reliable customer support, and fits within your budget.Integrating A/B Testing into Agile Development ProcessesIntegrating A/B testing into agile development processes can be a powerful way to validate design decisions and optimize user experiences. By leveraging the flexibility of agile methodologies, teams can iterate quickly and implement data-driven improvements. Here's what you need to know about integrating A/B testing into your agile development process:
A/B testing refers to a controlled experimentation technique where two or more versions (variants) of a webpage, application feature, or interface are compared to identify the version that performs better. This is done by randomly dividing users into separate groups and exposing each group to a different variant. Each group's behavior, such as click-through rates, conversion rates, or user feedback, is then analyzed to determine which variant performs better.
A key principle of agile development is an iterative approach with frequent releases of small yet functional increments. You can weave A/B testing into this approach by treating it as a built-in feedback loop for validating hypotheses and determining design improvements. Rather than relying solely on assumptions or guesswork, A/B testing encourages evidence-based decision-making.
Firstly, it's crucial to identify specific goals and metrics for your A/B tests. Determine what you want to achieve or optimize – whether it is increasing conversion rates, improving user engagement, reducing bounce rates, or any other measurable outcome relevant to your product or service. Keep these goals aligned with your agile development objectives to ensure that the tests support your wider strategies and business priorities.
Next, integrate A/B testing into the product development lifecycle by defining testable features and use cases in collaboration with your team members. Embed hypothesis-driven thinking from the beginning of the process and structure experiments to address specific questions about possible improvements. Embrace an incremental mindset so that smaller changes can be tested quickly and iteratively refined throughout the sprint cycles.
Leverage prototyping and wireframing tools to create alternative designs or versions for comparison in your A/B tests without affecting the core functionality currently in development. These prototypes can be built in parallel with the actual feature development, allowing for efficient and rapid testing while minimizing disruptions to the overall development flow.
During your sprint planning, allocate a portion of the team's capacity to implementing and conducting A/B tests. Define test acceptance criteria and determine how long an A/B test will run to gather sufficient data for significant results. Collaborate with developers, designers, and stakeholders to ensure clear communication about the purpose and expected outcome of each A/B test.
Integration of A/B testing into agile development necessitates strong analytics capabilities or tools for data collection and analysis. Utilize analytics platforms or appropriate tools that provide real-time insights and reports to track the results of your tests. Establish mechanisms to analyze data, interpret patterns and trends, assess statistical significance, and extract meaningful learnings to inform future iterations.
Be prepared to adapt or pivot based on the outcomes of A/B tests. Agile methodologies teach us that flexibility is key – if a test indicates that an alternative design or version yields better results, embrace the change and incorporate it into subsequent iterations. Gaining this flexibility through A/B testing helps teams move away from subjective decision-making driven by opinions or personal preferences.
Remember, A/B testing is not a one-time effort; rather, it should be an ongoing process integrated smoothly within your agile development cycle. Continuously using data-driven insights from A/B tests will allow you to iterate, refine, and continuously optimize your product or service, ultimately leading to improved user experiences and business outcomes.
By integrating A/B testing into agile development processes, teams can combine the benefits of fast-paced iterative development with evidence-backed decision-making. This integration promotes a culture of experimentation, encourages collaboration across roles, fosters continuous learning, and empowers teams to create user-centric products with significant impact.
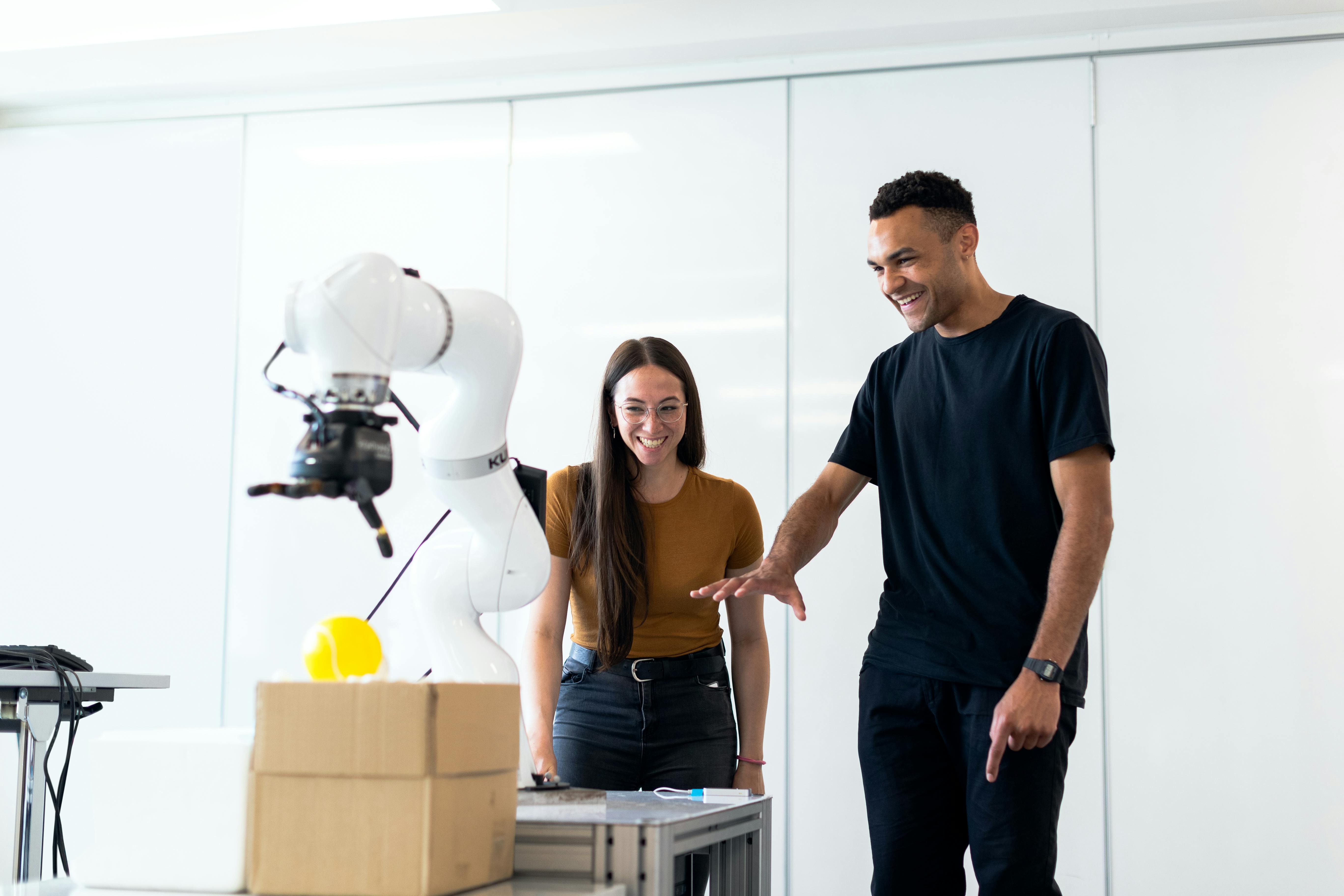
A/B testing refers to the process of comparing two or more versions (variants) of a webpage, user interface, or element to determine which one performs better. By dividing users into different segments, companies can identify the variant that resonates best with each group, tailoring the experience to their needs and goals.
There are numerous ways in which personalization through A/B testing can enhance the user experience. Firstly, it enables businesses to tailor content, products, or services specifically for different target audiences. By identifying users' demographics, interests, or preferences through analytics tools, companies create customized experiences that lead to higher engagement and conversion rates.
Furthermore, personalization improves relevance. When users feel that a website or app understands their unique requirements, they are more likely to stay engaged. By continually iteratively testing and fine-tuning various elements such as headlines, CTAs (calls to action), or visuals, companies can offer highly relevant experiences to each user segment.
A/B testing also lets businesses identify and resolve pain points across the user journey. By examining how different variations mitigate common issues such as confusing navigation or slow load times, brands can optimize UX and reduce barriers in the conversion funnel. This results in smoother, more seamless experiences for users at every touchpoint.
Additionally, customized experiences foster brand loyalty by creating a sense of relevance and empathy towards customers. Users appreciate when brands acknowledge their individuality and provide tailored solutions that align with their preferences. By utilizing A/B testing along with data-driven insights, businesses can showcase a deeper understanding of their audience and build meaningful relationships.
Implementing a successful personalization strategy through A/B testing requires proper planning and analysis. It is crucial to establish clear goals and metrics before conducting experiments to ensure accurate interpretation of results. For example, defining relevant key performance indicators (KPIs) like click-through rates, conversion rates, or session duration helps measure the impact objectively.
Regularly monitoring A/B test results and incorporating user feedback is also crucial as it allows refinement towards continuously improving the experience. Tests can encompass various elements such as design, copy, layout, or even pricing strategies. By iterating, learning, and adapting based on achievable insights, companies can create personalized experiences that meet the constantly evolving needs of their users.
In conclusion, personalization through A/B testing plays a vital role in enhancing user experiences. By crafting experiences optimized for different user segments through tailored content, resolving pain points in the user journey, and building closer relationships with customers, businesses can maximize engagement and conversions. With careful planning and analysis of quantitative and qualitative data, brands can continually refine their approach to ensure optimal personalization outcomes.The Role of Artificial Intelligence in Automating A/B TestsArtificial Intelligence (AI) has become a game-changer in various fields, and the realm of A/B testing is no exception. A/B testing refers to the process of comparing two variations of a webpage or marketing campaign to identify which one performs better. Traditionally, this process required considerable manual effort, expertise, and time. However, with the introduction of AI, automating A/B tests has emerged as an efficient and powerful approach to optimize marketing strategies.
One significant advantage of using AI is the ability to conduct tests simultaneously across multiple variables. While manual A/B testing focuses on assessing one variable at a time, AI allows for the examination of countless variables concurrently. This automation ensures that marketers can rapidly identify winning combinations while reducing experimentation cycles and saving valuable time.
Moreover, AI algorithms facilitate the automated analysis of immense sets of data that would be virtually impossible for humans to process manually. By sifting through copious amounts of data efficiently and accurately, AI algorithms provide reliable insights into user behavior, preferences, and trends. This insight helps marketers make informed decisions based on statistical significance rather than mere assumptions.
Through machine learning techniques, AI identifies patterns in user behavior from previous test data. This innate capability allows it to recognize trends and optimize future experiments accordingly. By identifying user segments with similar behaviors and preferences, AI can create personalized experiences and targeted campaigns tailored to specific audiences.
Another crucial role played by AI in automating A/B tests is interaction optimization. AI goes beyond measuring performance constructs like conversion rates; it predicts numerous user interactions throughout the entire customer journey. This prediction helps marketers prioritize key elements on a website or promotional material, allowing effective fine-tuning to maximize desired user actions.
AI-driven automation also enables dynamic allocation of traffic during A/B tests based on preliminary results. Instead of splitting traffic evenly across variations as done traditionally, applications of AI automate real-time allocation adjustments based on predicted outcomes. This adaptive allocation helps maximize conversions by allocating more visitors to the variation deemed most likely to succeed.
Additionally, AI can carry out multivariate testing - a more advanced version of A/B testing. This methodology assesses multiple variations simultaneously, allowing for complex experiments involving multiple factors. AI algorithms enable the discovery of interactions and correlations between variables that can provide profound insights into user behavior patterns.
Lastly, AI strengthens A/B tests by combining data from various sources such as web analytics, CRM software, or customer behavior tools. This integration enhances test accuracy and provides a holistic view of users' preferences and behaviors. It allows marketers to generate actionable insights that support data-driven decision-making and foster continuous improvement.
In conclusion, the role of AI in automating A/B tests revolutionizes the digital marketing landscape. Its ability to analyze vast volumes of data, simultaneously test multiple variables, predict user behavior, optimize interactions, dynamically allocate traffic, conduct multivariate testing, and combine various data sources provides powerful capabilities to transform and enhance marketing strategies. By incorporating AI into A/B tests, businesses can make smarter decisions faster and stay ahead of the competition in an increasingly data-driven world.
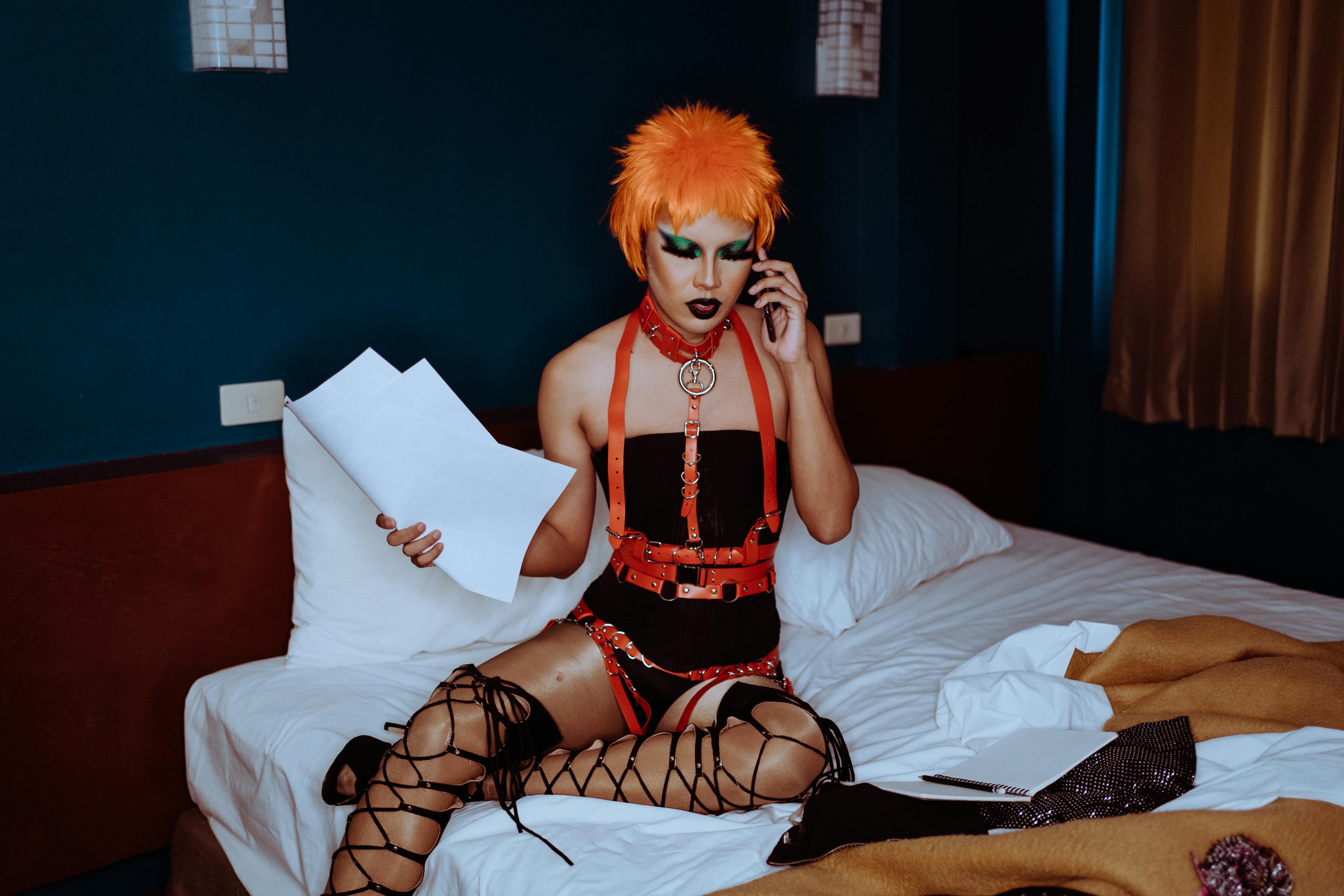
In today's rapidly changing business landscape, it has become crucial for organizations to foster a culture that embraces experimentation and learning from failure. This is especially true when it comes to testing new ideas and strategies. Here are some key points to consider in building such a culture:
Creating Psychological Safety: One of the first steps in fostering experimentation and learning from failure is creating an environment where team members feel safe to take risks. Cultivate a safe space where individuals can freely share their ideas, knowing that they won't be harshly judged or punished for failures.
Encouraging Risk-Taking: Encourage team members to explore new approaches and take calculated risks. Promote the mindset that failures are not seen as personal shortcomings but rather as opportunities for growth and learning. Provide them with support, resources, and autonomy to experiment without fear of negative consequences.
Leading by Example: Leaders play a crucial role in shaping a culture of experimentation and learning. They should exemplify vulnerability by sharing their own failures and lessons learned openly. By doing so, they break down barriers and inspire others to also embrace failure as a stepping stone toward success.
Rewarding Learning Opportunities: Offering recognition and positive reinforcement for individuals who actively seek out opportunities to learn from both successes and failures can foster a culture of continuous improvement. Celebrate learning experiences, provide public praise or rewards, and create spaces where shared knowledge is valued.
Iterative Processes: Prioritize iterative approaches within your organization. Encourage teams to break down projects into smaller, manageable experiments or iterations. Feedback loops should be built into the process so that insights gained from feedback can be promptly incorporated into future iterations.
Learning through Feedback: Emphasize the importance of actively seeking out feedback from customers, end-users, and colleagues throughout the experimentation cycle. Encourage open discussions around feedback, highlighting its value in refining ideas and uncovering blind spots.
Documentation and Sharing: Encourage individuals and teams to document their learning journey, capturing the lessons learned throughout the experimentation process. This documentation creates a shared knowledge base that can be readily accessible by others, helping to reinforce a culture of continuous learning.
Transparency and Communication: Foster transparent communication across teams and departments regarding experiments, successes, and failures. Encourage open dialogue where team members can freely discuss findings and learnings, enhancing collective understanding.
Learning from failure is an important ingredient in driving innovation, agility, and growth within organizations. By building a culture that embraces experimentation and treats failure as a stepping stone towards success, businesses can position themselves for long-term success in today's ever-changing landscape.Analyzing the Impact of A/B Testing on SEO and Online VisibilityAnalyzing the Impact of A/B testing on SEO and Online Visibility
A/B testing is a widely-used technique in the field of digital marketing and website optimization. It involves comparing two (or more) versions of a webpage or an element within the webpage to determine which one performs better in terms of achieving specific goals.
When it comes to SEO (Search Engine Optimization) and online visibility, A/B testing can have a significant impact. By carefully analyzing the results of these tests, marketers can optimize their websites to improve rankings on search engine result pages (SERPs) and increase overall visibility.
One key aspect of A/B testing is understanding how different page elements may affect SEO. For example, you can test variations of meta titles, descriptions, headings, and images to see what attracts more organic traffic or leads to higher click-through rates. This knowledge can help in fine-tuning these components to align with best practices for better search engine visibility.
Another important aspect is testing different website structures or layouts. Elements such as menu navigation, sidebar placement, or the placement and wording of call-to-action buttons can greatly impact the user experience, which directly affects SEO. By analyzing the data obtained from these tests, marketers can make informed decisions about optimizing their website's structure to drive higher engagement and overall visibility.
In addition to individual page elements, A/B testing can also be used to analyze different content formats. It allows marketers to experiment with text length, multimedia (videos, images), and even formatting styles like bullet points or subheadings. The results can help determine which content format delivers better SEO results in terms of increased traffic or longer on-page dwell time.
Furthermore, A/B testing can also shed light on non-textual elements impacting SEO and online visibility. Testing different page load times, mobile responsiveness, or even font choices may provide valuable insights into how these factors influence user behavior and consequently affect search engine rankings.
To fully analyze the impact of A/B testing on SEO and online visibility, it is crucial to measure a variety of metrics and key performance indicators (KPIs). Monitoring organic traffic, click-through rates, bounce rates, conversion rates, and SERP rankings are all representative of the effectiveness of different test variations.
Overall, by conducting thoughtful and comprehensive A/B tests, marketers can gain valuable insights into which design choices, content formats, or website optimizations can have the greatest impact on SEO and online visibility. Analyzing the data obtained through these tests leads to more informed decision-making, ultimately helping websites improve their search engine rankings and drive higher organic traffic and conversions.
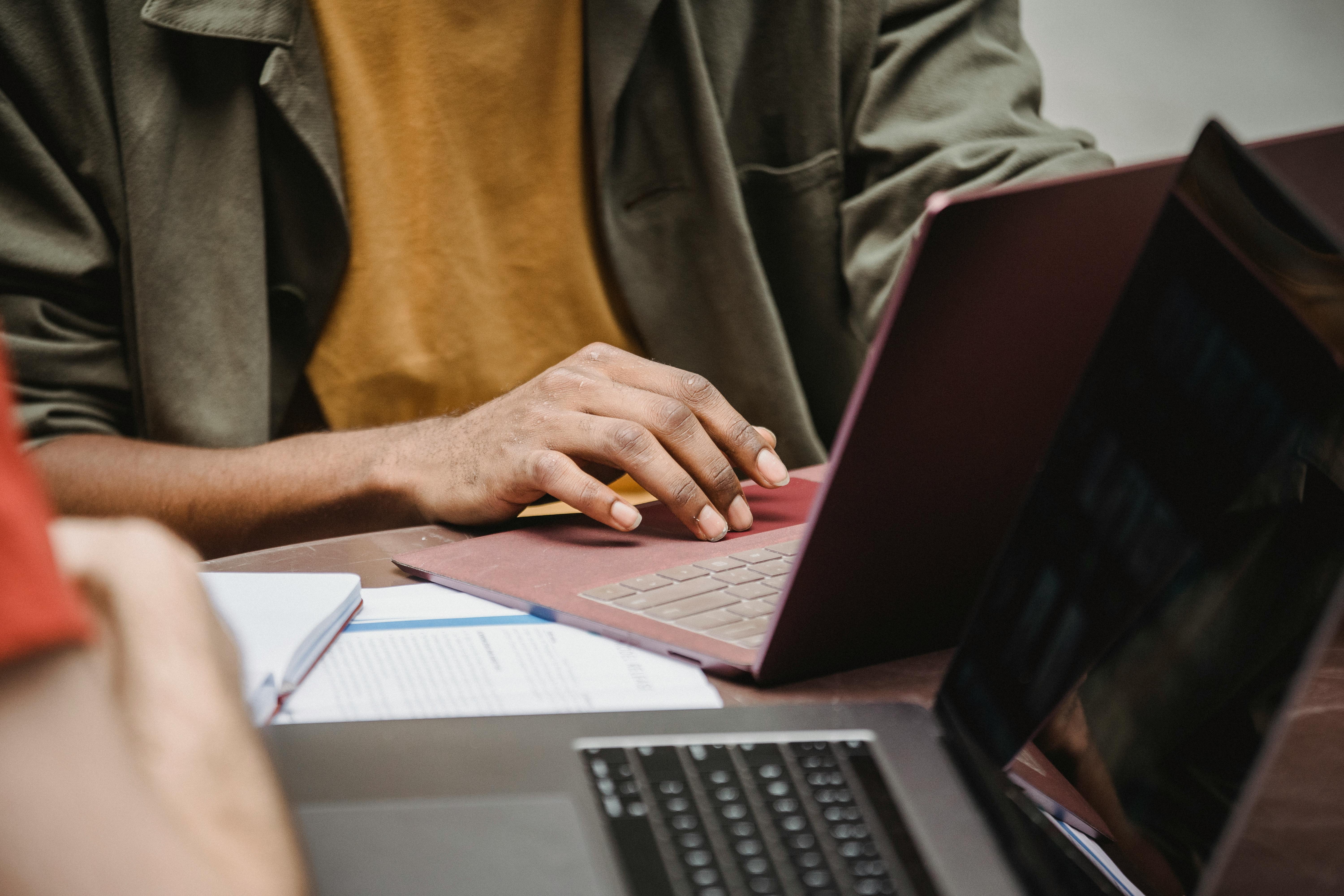
1. Personalization: One major trend is the increasing emphasis on personalized user experiences. Instead of generic A/B tests that consider an entire user base, tailored experiments are becoming more prevalent. By segmenting audiences based on demographics, preferences, or behavior patterns, businesses can create unique variations and track their impact more accurately.
2. AI-powered Testing: As artificial intelligence (AI) continues to advance, we can expect to see a shift towards AI-driven A/B testing tools. Machine learning algorithms can aid in automating certain aspects of experiment analysis, making testing more efficient and enabling real-time continuous optimization.
3. Multi-armed Bandit Approach: The traditional A/B testing method requires splitting users into equal groups between control and variant versions. However, the multi-armed bandit approach balances traffic dynamically by assigning more users to the better-performing variant over time. This technique reduces potential revenue loss associated with the initial stages of traditional A/B testing.
4. Testing on Multiple Devices and Platforms: With the increasing use of smartphones, tablets, smartwatches, and other internet-enabled devices, running A/B tests across multiple devices and platforms has become crucial. Ensuring consistent user experiences across all digital touchpoints is vital for maintaining relevance and engagement.
5. Ethical Considerations: In response to growing concerns about data privacy and digital ethics, conducting A/B tests with ethical considerations will become essential. Striking the right balance between collecting valuable insights and being transparent while respecting user privacy rights will be a significant aspect to focus on.
6. Democratization of A/B Testing: As the use of A/B testing expands, it's predicted that the tools and techniques will become more accessible to a wider range of organizations and professionals. This democratization enables smaller businesses and individuals to leverage this powerful testing method to make informed decisions and enhance user experiences without extensive technical knowledge.
7. Beyond Conversion Rates: Moving beyond conversion rates, future A/B testing may focus on evaluating long-term customer value, retention rates, or even intangible factors like user satisfaction. Expanding the scope of metrics measured can provide a more holistic picture of the impact of variations on overall success.
By paying attention to these future trends in A/B testing, businesses can effectively adapt their strategies, gain deeper insights from experiments, and offer personalized experiences in an ever-evolving digital ecosystem. To remain competitive in the market, continuous learning and leveraging cutting-edge technological advancements are crucial to maximizing the effectiveness of A/B testing methodologies.
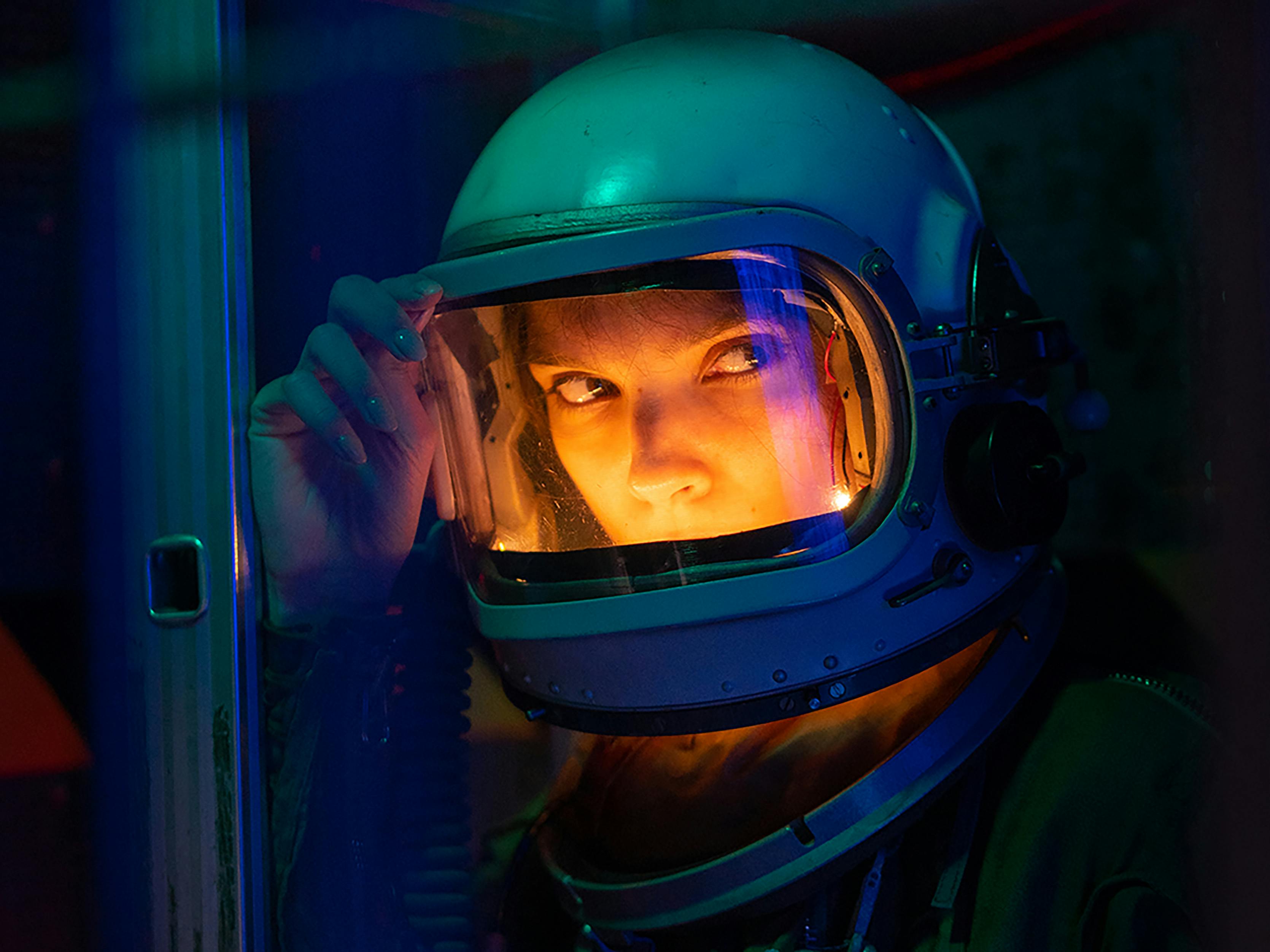
A/B testing, a popular method in the field of marketing and user experience research, allows businesses to compare two versions of a webpage or app to determine which one performs better. This testing methodology primarily focuses on immediate metrics, such as click-through rates, conversion rates, and engagement levels. However, these metrics only provide a snapshot of user behavior at a particular point in time. To gain deeper insights into the long-term impact of these changes, it is essential to go beyond the click and measure the continued effects over an extended period.
One crucial aspect of measuring long-term effects through A/B testing lies in understanding user behavior beyond the initial interaction. By tracking behaviors such as repeat visits, product purchases or subscriptions after the initial test period, businesses can gain insights into user loyalty and ongoing engagement. These metrics provide valuable information about how sustainable the changes made during A/B testing are.
It is also important to consider the potential ripple effects that A/B testing may have on other user actions and experiences. Focusing solely on immediate metrics may not reveal any unforeseen negative outcomes caused by the changes made to improve short-term results. By monitoring additional metrics like bounce rates or customer support inquiries following A/B tests, businesses can assess if alterations negatively impact other aspects of user experience.
Furthermore, analyzing the influence of changes made through A/B testing on key business drivers can lead to a better understanding of their long-term effects. For example, if an alteration demonstrates increased conversions or revenue shortly after implementation, it is critical to examine whether these improvements are sustained over time. Robust data analysis can help ascertain if temporary gains are just short-lived spikes or if they actually establish new baselines for future growth.
To accurately measure long-term effects, it is necessary to define appropriate time frames for evaluation. While some changes may exhibit lasting impacts immediately after implementation, others may require weeks or even months to manifest significant effects. By establishing clear evaluation periods and regularly revisiting data, the true longevity of A/B test improvements can be assessed.
In addition to quantitative analysis, qualitative research methods can also offer insights into the long-term effects of A/B testing. Collecting post-test feedback from users, conducting surveys, or organizing user interviews can reveal how changes made through A/B testing have influenced user perceptions, satisfaction levels, and brand loyalty over time. This cumulative user feedback enables businesses to validate or adjust their hypotheses formed during A/B testing further.
Finally, a comprehensive approach to analyzing the long-term effects of A/B testing involves measurement beyond individual experiments. Effective tracking of iterations over time provides more nuanced insights into overall trends and patterns. Comparing multiple A/B tests conducted at different times can uncover recurring themes that contribute to sustainable success or highlight areas for improvement.
In conclusion, going beyond immediate metrics and measuring the long-term effects of changes made through A/B testing is crucial for businesses seeking sustained success in marketing and user experience optimization. By understanding user behavior beyond the initial click, assessing ripple effects on other metrics and experiences, monitoring key business drivers, setting appropriate evaluation time frames, incorporating qualitative research, and analyzing collective data across numerous experiments, businesses can ensure their efforts lead to lasting improvements that yield considerable benefits in the long run.
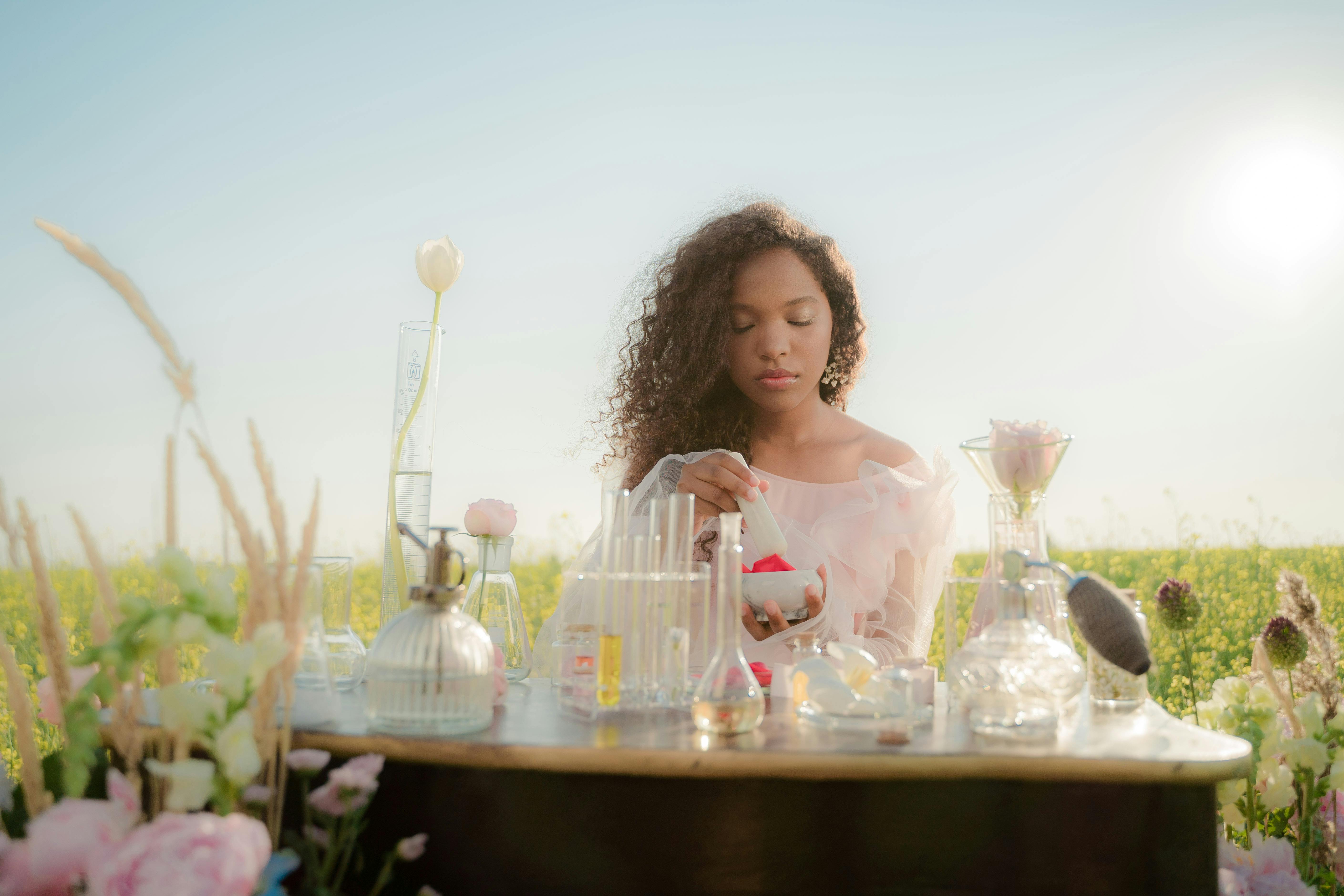