Understanding the Fundamentals of A/B TestingUnderstanding the Fundamentals of A/B testing
A/B testing is an essential component of a data-driven marketing strategy. It allows businesses to experiment with different variations of a webpage, email campaign, or advertisement to identify which version performs better. By comparing two versions – A and B – marketers can make informed decisions backed by quantitative insights. To grasp the fundamentals of A/B testing, consider the following key points:
1. Objective: Before embarking on an A/B test, it is crucial to define a clear objective. Whether it's increasing click-through rates, improving conversions, or enhancing user engagement, a specific goal will shape the entire test.
2. Hypothesis: Based on your objective, develop a hypothesis – an educated guess about which variation will achieve the desired outcome. This forms the foundation for your A/B test and provides a direction for analysis.
3. Variables: Identify the variables you want to test within your content or interface, such as headline, call-to-action button color, layout, or pricing structure. Choose one variable at a time for accurate measurement and conclusive results.
4. Test Groups: Split your audience into two randomly assigned groups – one exposed to the control version (A) and the other to the variation (B). It is crucial to ensure both groups are representative and equal in size to draw meaningful conclusions.
5. Duration: Determine the appropriate duration for your A/B test based on sufficient sample size and statistical significance. Running tests too briefly might not provide reliable results, while excessively long tests can delay decision-making processes.
6. Measurement Metrics: Define which metrics you will track during the testing phase to measure performance accurately. These metrics could include click-through rates, conversion rates, bounce rates, form completions, or revenue generated.
7. Statistical Analysis: Once the test is complete, conduct statistical analysis on the accumulated data to ascertain any significant differences between versions A and B of your content or interface. Statistical significance helps determine if any observed variations are reliable or merely random results.
8. Learnings and Insights: Analyze the test results to uncover insights on user behavior, preferences, and their impact on conversions or engagement. Learning from every A/B test gives valuable guidance for future optimization strategies.
9. Documentation: Record and organize your A/B testing processes, including hypotheses, variables tested, metrics measured, and results obtained. This documentation enables an informed understanding of previous tests and establishes a reproducible framework for future experiments.
10. Iterative Testing: A/B testing is an ongoing process; it should be embraced as an iterative practice aimed at continuous improvement. By consistently experimenting with different ideas, marketers can steadily refine their content or interfaces to obtain optimal results.
11. Factors to Consider: Lastly, keep in mind various factors while conducting A/B tests, such as traffic volume, target audience segments, potential biases, test frequency, and the limitations of platforms or tools used for testing.
By understanding these fundamentals of A/B testing, marketers can make well-informed decisions driven by data rather than assumptions. This empirical approach empowers businesses to optimize their digital presence, enhance user experiences, and achieve better outcomes in a constantly evolving market landscape.
A/B Testing: The Key to Enhancing User ExperienceA/B testing is a powerful tool used in the world of web design and digital marketing. It is an experimental method in which two variations, A and B, of a webpage or app are shown to users at random to determine which one performs better. By conducting A/B tests, businesses gain insights into user preferences, behaviors, and expectations to make data-driven decisions aimed at enhancing the overall user experience.
The primary goal of A/B testing is to compare variations in real-world scenarios and identify which one produces statistically significant results. This testing technique allows organizations to understand how changes like different button colors, altered layouts, revised headlines, or even modified pricing strategies can impact conversions, click-through rates (CTRs), engagement levels, or other key performance indicators (KPIs).
To conduct an A/B test, companies need to create two versions of their webpages (A and B) that differ only in the specific element or feature they want to evaluate. Elements commonly tested include design aspects, content positioning, call-to-action (CTA) wording, navigation menus, and more. Randomly dividing visitors into two groups (with an equal audience size) ensures unbiased results.
To measure the performance of these two versions accurately, it's imperative to define clear conversion goals and decide which metrics to observe closely during the test. Whether it be newsletter sign-ups, purchases made, or time spent on a page, having predefined objectives and metrics allows for better interpretation of the test results.
One essential principle in A/B testing is conducting experiments over a certain period to ensure diverse user behaviors and minimize external factors that could influence the outcomes. Seasonal trends or special promotions might affect user behavior disproportionately if the testing period is too short.
The success of an A/B test hinges on the accurate collection and analysis of reliable data. Fortunately, there are numerous tools available for implementing A/B tests efficiently. These tools assist businesses in monitoring user interactions and generating reliable statistics, ensuring statistical significance and reliable conclusions.
While A/B testing can significantly enhance user experience and drive conversion rates, it is crucial to understand that it is an ongoing process. Once a test yields conclusive results, companies should implement the preferred version and continue iterating to find further improvements. Regularly conducting A/B tests allows businesses to stay up-to-date with user expectations and preferences, ensuring their websites or apps continuously evolve for optimum user satisfaction.
In conclusion, A/B testing is a valuable technique that enables businesses to make informed decisions based on actual user feedback. By implementing this method effectively, companies can optimize their digital assets and enhance the overall user experience, leading to increased engagement, conversions, and measurable success.Balancing Cost vs. Benefit in A/B Testing StrategiesBalancing Cost vs. Benefit in A/B testing Strategies
A fundamental aspect of conducting successful A/B testing is finding the delicate balance between the costs and benefits associated with implementing and running your experiments. In this process, you strive to optimize your outcomes while maintaining a reasonable expenditure of resources. Here's what you need to know about balancing cost versus benefit in A/B testing strategies.
1. Defining Costs:
Costs in A/B testing refer to the resources you invest, such as time, money, manpower, and technology. Identifying and estimating the upfront and ongoing costs involved will allow you to make informed decisions throughout the testing process.
2. Understanding Benefits:
Benefits encompass the positive impact or uplift achieved through effective A/B testing. These benefits vary depending on your objective, whether it involves increasing conversion rates, improving user experience, boosting revenue, or achieving other key performance indicators for your business.
3. Finding the Right Balance:
A successful A/B testing strategy requires finding an equilibrium that maximizes benefit while keeping costs under control. This balance can be approached from two perspectives: cost control and benefit maximization.
4. Cost Control:
To control costs effectively, start by carefully crafting your hypotheses and identifying areas of focus to ensure you're not wasting resources on irrelevant variables. You can also prioritize test ideas based on their potential impact and feasibility in order to allocate resources wisely.
5. Ensuring Statistically Significant Results:
Minimizing costs means conducting tests long enough to gather statistically significant results without unnecessarily prolonging them. This requires estimating sample sizes accurately with statistical confidence levels in mind.
6. Simplicity and Iteration:
Keeping tests simple can help reduce costs and speed up iterations. Complex A/B tests may require more extensive engineering effort or additional technological resources, which may not always be justifiable. Adopting an iterative approach where you continually learn from previous experiments can maximize benefits without unnecessary expenses.
7. Analyzing Results Efficiently:
Perform detailed post-test analyses to extract meaningful insights without wasting time or resources. Prioritize the analysis of metrics directly linked to your main objectives, ensuring that any actionable findings can be exploited to your advantage.
8. Strategic Roadmapping:
Balance cost and benefit by having a well-defined roadmap that outlines the order and priority of tests. This type of strategy can prevent overlapping experiments, optimize resource allocation, and provide a clear path for achieving desired outcomes.
9. Continuous Monitoring and Adaptability:
Constantly monitor your A/B tests to detect any significant deviation from your expectations or emerging trends. This allows for timely adaptation and minimizes costs related to chasing false positives or negatives.
10. Learning from Failed Tests:
Failed A/B tests can also generate valuable knowledge. Instead of considering them as a complete loss, analyze them carefully to understand why they failed and how you can refine future experiments based on the lessons learned.
In conclusion, balancing the cost versus benefit in A/B testing strategies is essential for optimizing outcomes within the allocated resources. By controlling costs, ensuring statistically significant results through efficient test setups, emphasizing simplicity, analyzing results strategically, and continuously learning from experiments, you can strike the right equilibrium between cost-effectiveness and the achievement of desired benefits.Navigating the Pros and Cons of A/B TestingA/B testing is a vital component of the testing and optimization process for any website or digital marketing campaign. It involves comparing two versions of a webpage or an ad to determine which one performs better in terms of engagement, conversions, or other predefined goals.
On the positive side, A/B testing allows businesses to make data-driven decisions. By splitting traffic between two variations and measuring user behavior, companies can obtain valuable insights into customer preferences. This data serves as evidence for implementing changes that have a high probability of generating positive results.
One major advantage of A/B testing is that it helps optimize user experience. By analyzing user interactions on different versions, businesses can fine-tune their websites or applications by making changes that resonate better with their target audience. Whether it's adjusting button placements, color schemes, or font sizes, A/B testing allows for continuous improvement and enhances overall user satisfaction.
Another pro is that A/B testing helps improve conversion rates. By understanding which version of a webpage attracts higher engagement and a greater number of conversions, businesses can adapt their strategies accordingly. This approach significantly reduces the risk involved in making site-wide changes based solely on assumptions or guesswork.
Additionally, A/B testing enables companies to explore creative avenues by experimenting with different design elements, content layouts, or even promotional messages. By monitoring user response to these variations, businesses can identify innovative and effective ways to communicate their offerings and capture the attention of potential customers.
However, there are some cons and challenges to consider when embarking on A/B testing. One limitation is that significant traffic volumes are required to achieve statistically significant results. When dealing with low-traffic websites or specialized niche markets, obtaining actionable insights from A/B tests becomes more challenging due to decreased sample sizes.
Time constraints can also pose a drawback. Properly planning and executing an A/B test demands adequate time and resources. From formulating hypotheses to designing variations, setting up tracking mechanisms, acquiring statistically sound samples, and analyzing results, the process requires patience and diligence. Rushed or poorly designed tests might yield inconclusive or misleading findings.
Conditions may also change over time. Visitors' preferences and behavior can evolve, rendering previously successful A/B testing results irrelevant or outdated. Continuous monitoring and retesting become necessary to ensure ongoing optimization.
Another potential drawback is that A/B testing can lead to a conservative mindset, limiting innovation. Relying solely on incremental changes based on past data might hinder exploration of entirely new ideas or strategies that have yet to be proven.
Despite these challenges, navigating the pros and cons of A/B testing intelligently allows companies to harness its benefits successfully. A data-informed decision-making process, combined with creativity and sound judgment, paves the way for continued improvement of user experiences, increased conversions, and more effective marketing efforts overall.Real-Life Success Stories: Transformative Impact of A/B Testingtestimonials of individuals or companies achieving remarkable success through A/B testing are proof of its transformative impact. Through analyzing user behavior and manipulating variables, A/B testing evaluates different versions of a webpage or app to determine which performs better in terms of conversions, engagement, or revenue generation.
One such success story involves a popular e-commerce company. By implementing A/B testing to modify their checkout process, they significantly increased their conversion rates. By carefully testing iterations and analyzing user feedback, they were able to refine the purchasing experience, remove potential friction points, and boost their overall sales.
Another example is a content-based website that relied heavily on advertising revenue. They utilized A/B testing to optimize ad placement, format, and design. This exploration resulted in notable increases in click-through rates (CTRs) and revenue per page view. By continuously experimenting with different arrangements and studying analytical data, they ultimately reached a more effective and profitable advertisement setup.
A mobile app development company utilized A/B testing to improve user engagement and reduce churn rate. They experimented with various push notification strategies and designs to entice users to take certain actions within the app. After analyzing test results and learning from user preferences, they managed to personalize notifications while minimizing annoyance, leading to substantially higher app usage and reduced customer churn.
In yet another instance, a large retailer implemented A/B testing on their search algorithm to enhance their website's functionality. By conducting tests on alternative search result layouts and algorithms, they improved search accuracy and relevance. This overhaul resulted in customers finding products more efficiently, driving up overall sales and customer satisfaction levels.
Notably, a subscription-based service was facing a significant drop-off rate during the onboarding stage. Upon implementing A/B testing to explore different registration forms and modifications, they successfully decreased churn and achieved higher user retention rates. Refined onboarding experiences not only retained more users but also guided them toward successful outcomes within the platform.
Each of these real-life success stories highlights the power of A/B testing to revolutionize business operations. By diligently experimenting, measuring, and adapting, these companies transformed their conversions rates, engagement metrics, revenue figures, and overall success levels.
In conclusion, A/B testing has a transformative impact on businesses by unlocking their full potential through data-driven decision-making. Companies can learn from these success stories and implement similar strategies to identify areas of improvement, tailor experiences to individual preferences, and drive both customer satisfaction and financial gains.
How A/B Testing Fuels Data-Driven Decision MakingA/B testing is an invaluable technique that fuels data-driven decision making. It enables businesses to make informed decisions based on empirical evidence rather than mere assumptions or guesswork. By leveraging this approach, companies can optimize various aspects of their operations and increase their chances of success.
At its core, A/B testing involves the creation of two or more variants (A and B) to test against each other. These variants typically differ in a single variable, such as website layout, call-to-action buttons, or email subject lines. The goal is to determine which variant performs better by comparing the results from both groups.
Data lies at the heart of A/B testing. A variety of metrics can be tracked and analyzed depending on the nature of the experiment. This can include conversion rates, click-through rates, bounce rates, average revenue per user, and many others. By quantifying these metrics for each variant and analyzing the data collected, businesses gain valuable insights into the effectiveness of their changes.
A/B testing delivers reliable and tangible results that eliminate speculation and assumptions. Through data collection and analysis, it provides objective evidence for decision making. It allows companies to understand how changes impact user behavior and overall performance, leading to optimized strategies with proven outcomes.
This scientific approach fosters iterative improvement since A/B testing should be an ongoing process rather than a one-time event. By constantly refining and experimenting with different variations, businesses strive for continuous success through incremental enhancements.
Moreover, A/B testing democratizes decision making. Instead of relying solely on intuition or biases from key stakeholders, organizations can rely on solid evidence provided by statistical analysis. This reduces internal dispute and supports a culture of data-driven decision making within companies.
Ethical considerations play a crucial role in utilizing A/B testing effectively. Ensuring proper consent and transparency with users involved in experiments is essential. Respecting privacy concerns and ensuring data protection should be prioritized when designing, conducting, and analyzing A/B tests.
Overall, A/B testing underscores the importance of data-driven decision making for businesses striving for success. It allows companies to make informed choices based on evidence-backed results, leading to optimized strategies and improved outcomes. By democratizing decision making and driving iterative refinement, A/B testing contributes substantially to a company's growth and achievement objectives.
Avoiding Common Pitfalls in A/B Testing CampaignsTitle: Avoiding Common Pitfalls in A/B testing Campaigns
Introduction:
A/B testing campaigns can be highly effective in optimizing marketing strategies by leveraging data-driven insights. However, these campaigns can easily fall prey to common pitfalls, leading to inaccurate results and potentially detrimental decisions. In this blog, we will discuss vital tips to help you avoid these common pitfalls and make the most out of your A/B testing campaigns.
1. Insufficient Sample Size:
When conducting an A/B test, it is crucial to ensure that your sample size is sufficiently large for statistically significant results. Insufficient sample sizes can result in unreliable outcomes that may not accurately represent your target audience's preferences, leading to flawed conclusions and decision-making.
2. Testing Multiple Variables Simultaneously:
Testing more than one variable at a time within a single A/B test often arises as a significant pitfall. This approach hinders effective analysis since it becomes challenging to attribute changes in outcomes to specific variables. To generate meaningful insights, it is best to focus on a single variable while keeping other elements constant.
3. Randomization Failure:
Failure to implement randomization properly can introduce biases into your A/B tests. Random assignments ensure that each group has an equal likelihood of being exposed to different variants, making the results fair and reliable. Failing to adhere to this principle may yield skewed or false conclusions.
4. Misinterpreting Results:
Analyzing data from an A/B test requires a thoughtful approach. Rushed or biased interpretations can adversely impact decision-making processes. Remember that correlation does not always imply causation, so exercise caution when deriving insights from your results.
5. Ignoring Long-term Impact:
A successful A/B testing campaign should account for potential long-term implications of changes made during the testing phase. It may be essential to examine different metrics beyond immediate gains or losses to understand the broader effects on customer behavior, retention, or overall conversion rates.
6. Overlooking Contextual Factors:
A/B testing campaigns conducted in isolation, disregarding broader contextual factors, may not deliver accurate results. External factors like seasonality, holidays, or other ongoing marketing initiatives can significantly influence user behavior, rendering your A/B test conclusions misleading. Considering these factors is crucial for obtaining meaningful and actionable insights.
7. Prejudiced Sample Selection:
During participant selection for your A/B tests, avoiding biased subject recruitment is vital. Biases stemming from demographic imbalance or selective sampling can taint your test's validity by introducing unique characteristics that are not representative of the target audience. Strive for randomness to avoid skewed outcomes.
8. Premature Conclusions:
Resisting the urge to reach premature conclusions during an A/B testing campaign is crucial. It is advisable to wait for tests to run their full course and collect sufficient data before drawing definitive results. Premature decisions based on limited data can lead to suboptimal strategies and missed opportunities.
Conclusion:
A/B testing can be a potent tool, aiding marketers in refining tactics and strategies to enhance user engagement, conversions, and overall business growth. By considering these common pitfalls and adopting best practices, you can enjoy greater success in your A/B testing campaigns and leverage them effectively to boost your marketing efforts. Remember, compelling insights come from careful execution and unbiased interpretation of results.
Ethical Considerations in A/B Testing PracticesEthical considerations play a vital role in the A/B testing practices adopted by companies and organizations. When conducting experiments that involve capturing user data or influencing their behavior, it is crucial to address concerns related to privacy, transparency, consent, and fairness. Ignoring ethical considerations not only undermines trust with users but also exposes businesses to legal repercussions and reputational damage. Thus, when conducting A/B tests, it is essential to adhere to certain ethical principles and guidelines.
Transparency:
To ensure transparency, it is important to clearly inform users about the ongoing A/B test. Communicate how their data will be used, what changes they might notice, and their ability to opt-out if desired. By providing this information, users become aware of their participation in an experiment rather than being subjectively tested without their knowledge.
Informed Consent:
Prioritize informed consent by making sure users understand the purpose and potential impact of the A/B test before participating. Obtain explicit permission from users to include them in any such experimental procedures. By respecting users' autonomy and allowing them to choose whether to participate or not, you maintain ethical standards.
Minimal Risk:
A/B tests should ideally pose minimal risk to participants’ privacy and well-being. Minimize the collection of personally identifiable information (PII) unless necessary for the experiment. Always ensure that data collected is treated with the utmost confidentiality, protected from unauthorized access or misuse.
Avoidance of Harm:
Prevent any harm resulting from the A/B testing process. Monitor the potential adverse consequences of any changes being evaluated and take measures to mitigate negative impacts on users. Assess and minimize disruption or frustration caused by altered user experiences.
Data Protection:
Protect user data by utilizing appropriate security measures to prevent unauthorized access, manipulation, or sharing of sensitive information. Anonymize user data whenever possible while preserving the integrity of the experiment results.
End-to-End Encryption:
Employ end-to-end encryption when transmitting user information during A/B tests to ensure data security. Use secure protocols and established encryption techniques to safeguard user privacy and data.
Fairness:
Ensure fairness when designing A/B tests by distributing changes and exposures evenly across different user segments, preventing an unjust disadvantage for certain individuals or groups. Avoid discriminatory practices or biased outcomes that could negatively impact certain sections of your user base.
Data Deletion:
When an A/B test is finished, delete unnecessary user data collected during the experiment promptly. Only retain anonymized information essential for analysis and interpretation of results while adhering to applicable data protection regulations.
Clear Impact Assessment:
Always assess and analyze the potential impact of each A/B test beforehand. Consider the ethical implications, consider alternatives, and predict possible consequences before implementing any changes. Regularly evaluate the ongoing experimentation processes to address emerging ethical concerns.
Continuous Improvement:
Maintain an ongoing commitment to ethical considerations in your A/B testing practices. Continuously review and upgrade your privacy policies, data handling processes, and consent mechanisms based on evolving regulations, industry standards, and input from human rights advocacy groups.
By incorporating these ethical practices into your A/B testing routines, you can promote trust with users, safeguard their privacy, and provide fair experiences while collecting valuable insights for improving business outcomes.
A/B Testing Tools and Technologies: Simplifying the ProcessA/B testing tools and technologies have become imperative for businesses seeking to improve their websites, apps, or marketing campaigns. These robust platforms simplify the process, allowing companies to optimize their strategies and make data-driven decisions without a hassle.
One commonly-used tool is Google Optimize, a web testing and personalization platform. It integrates seamlessly with Google Analytics, presenting a user-friendly interface that simplifies the creation and execution of A/B tests. With its editor mode, marketers can make changes directly on the website without the need for coding skills.
Optimizely is another popular A/B testing tool utilized across various industries. Its powerful features enable businesses to experiment product improvements, test different user experiences, and optimize content performance effortlessly. The platform supports multivariable testing, allowing teams to examine multiple variations at once.
VWO (Visual Website Optimizer) is a versatile A/B testing software known for its dynamic and comprehensive functionalities. This tool encompasses heatmaps, click maps, and advanced targeting options, enabling marketers to deep dive into user behavior. Alongside its seamless integration, VWO offers quick test setup through a visual editor.
Adobe Target combines A/B testing capabilities with advanced personalization features. This technology equips marketers with the ability to target specific audience segments using AI-enhanced algorithms intelligently. Adobe Target provides mobile app testing as well, ensuring optimization across all platforms.
Dynamic Yield specializes in employing personalization techniques alongside A/B testing. This all-encompassing platform captures user data in real-time to deliver tailored experiences at scale. Its smart algorithms allow businesses to automatically adapt content based on users' preferences, boosting conversions significantly.
Lastly, Mixpanel's A/B Testing functionality empowers organizations to experiment and iterate on different features or user journeys. This technology enables targeted messaging to specific user cohorts and supplies valuable insights into engagement metrics. Additionally, Mixpanel facilitates trend analysis and funnel tracking, guiding companies towards high-impact optimizations.
In conclusion, A/B testing tools and technologies play an integral role in streamlining the optimization process. These innovative platforms, such as Google Optimize, Optimizely, VWO, Adobe Target, Dynamic Yield, and Mixpanel, simplify complex tests while providing elaborate insights to enhance customer experiences and drive successful outcomes.
Tailoring A/B Tests for Different Industries: Best PracticesTailoring A/B tests for Different Industries: Best Practices
A/B testing is a valuable analytical tool used to assess and evaluate the performance of different versions of a web page or application. However, it is crucial to understand that every industry operates uniquely and has its own set of requirements. To effectively tailor A/B tests for different industries, consider the following best practices:
1. Industry-specific goals: Each industry has its specific objectives and key performance indicators (KPIs) that dictate success. Tailor your A/B tests to contribute directly to these goals. For example, an e-commerce site might focus on increasing conversions, while a media company could prioritize user engagement and time spent on content.
2. Understand your target audience: Extensive knowledge about your audience's preferences, behaviors, and biases is essential. Conduct robust market research to identify specific metrics and behaviors relevant to your industry.
3. Segmenting audiences: Depending on your industry, audience segmentation can be highly effective in tailoring A/B tests. Split users into distinct groups based on demographics, geographics, or their stage in the buyer's journey. This allows you to create targeted experiments catering to different segments.
4. Design captivating variations: The visual appeal and user experience of your website or application significantly impact conversion rates and engagement levels. Craft visually appealing variations that align with industry norms and best practices to capture users' attention effectively.
5. Consider mobile responsiveness: With expanding smartphone usage, ensure that your A/B tests cater to mobile devices as well. Test how variations in design impact the user experience across various screen sizes and operating systems commonly used within your target industry.
6. Iterative testing: Almost never settle on the first variation tested in an A/B experiment. Continuously iterate upon successful findings to refine and optimize outcomes in a rapidly evolving world.
7. Size matters...sample size! The sample size plays a vital role in achieving statistically significant results from your A/B tests. Ensure your sample size adequately represents your industry's target audience to generate meaningful and actionable findings.
8. Timing and seasonality: Take into account potential seasonal variations that might influence user behavior. Accounting for these trends could prevent inaccurate conclusions based on temporary situations.
9. Data-driven decision-making: Stay committed to making decisions based on data rather than personal opinions or assumptions. Rely on quantitative and qualitative data generated from your A/B tests to uncover insights specific to your industry.
10. Monitor long-term effects: Short-term test results may not always align with long-term impacts. Keep track of how variations affect sustainability, customer satisfaction, and overall business objectives in the long run.
11. Learn from other industries: Embrace cross-industry learning by observing successful tactics implemented by others. Often, valuable insights learned in one sector can be adapted and successfully applied to different industries.
By adhering to these best practices and tailoring your A/B tests according to specific industry requirements, you can maximize the effectiveness of your tests and accomplish desired goals in a more targeted manner.Beyond the Basics: Advanced Techniques in A/B TestingA/B testing has proven to be a useful method for understanding user behavior and making data-driven decisions. It allows businesses to test different versions of their website or app and analyze which one performs better, ultimately improving conversions and user experience. While the concept of A/B testing is fairly easy to understand, there are advanced techniques that go beyond the basics, unlocking even more insights and potential benefits.
Advanced techniques in A/B testing involve strategic approaches to optimize experiments further and get more accurate results. These techniques often require more advanced statistical knowledge and proper testing methodologies. Let's delve into some of these advanced techniques that can take your A/B testing to the next level:
1. Sequential Testing: Unlike traditional A/B testing, sequential testing allows you to make decisions before waiting for a fixed sample size. This technique helps you determine the optimal conversion rate quickly by continuously analyzing incoming data and making real-time decisions. It saves time because you can stop a test as soon as conclusive results are obtained, rather than waiting for all data points.
2. Multi-Armed Bandit Testing: This technique involves dynamically allocating traffic between different variations based on their performance during the test. Instead of running multiple equal groups simultaneously like in typical A/B testing, multi-armed bandit testing shifts the balance towards the most promising variations over time. As a result, you can mitigate potential losses caused by inferior variations during the early stages of a test.
3. Bayesian A/B Testing: Bayesian statistics looks at probability differentially before and after new data is included. This approach allows businesses to estimate conversion rates accurately with smaller sample sizes while still taking prior estimations into account. It also enables continuously updating beliefs about variations during an ongoing A/B tests, providing a refined understanding of what's working best.
4. Fractional Factorial Design: This technique allows you to reduce the number of variations required for testing multiple elements or factors within an experiment exponentially. By strategically selecting which combinations to test, you can effectively identify the main contributing factors and interactions between them with minimal resources expended.
5. Personalization and Segmentation: Advanced A/B testing considers personalizing experiences for different user segments. Rather than testing for the entire user base, you divide it into smaller segments with specific demographics or behaviors and tailor the variations accordingly. This emphasizes the idea that a one-size-fits-all approach may not be effective, as different segments may respond better to specific variations.
6. Incrementality Testing: Incrementality testing is about figuring out whether your experiment itself causes the determined change in user behavior or if external factors, such as seasonality or promotions, are responsible. By conducting controlled experiments alongside outside influences, you can accurately attribute changes to the experiment itself and assess its true impact.
Remember, advanced A/B testing techniques require careful consideration of statistical significance, sample sizes, and thorough analysis. Engaging with an experienced data scientist or expert in A/B testing can help ensure accurate implementation and interpretation of results. By leveraging these advanced techniques, you can gain deeper insights into user preferences and optimize your digital experiences effectively.
Integrating A/B Testing into Your Marketing Strategy: A Step-by-Step GuideIntegrating A/B testing into Your Marketing Strategy: A Step-by-Step Guide
When it comes to optimizing conversion rates and fine-tuning your marketing strategies, A/B testing is a powerful tool that can make a significant impact. By comparing multiple versions of a webpage or marketing campaign, you can gather valuable data and insights to understand what works best for your audience. Here's a detailed guide on integrating A/B testing into your marketing strategy:
1. Define Your Objective:
- Begin by clearly defining your goal or objective for the test. Identify what you want to achieve and outline the metrics or Key Performance Indicators (KPIs) that will help measure success.
2. Decide on the Element to Test:
- Evaluate different elements of your marketing efforts that you believe can lead to improvement. This may include web page layouts, headlines, copywriting, design elements, call-to-action buttons, email subject lines, or even pricing structures.
3. Create Variations:
- Develop multiple variations of the element you have chosen to test. For example, if you are testing a landing page headline, create two or more alternatives that present slightly different messaging approaches.
4. Set Up the Test:
- Utilize a reliable A/B testing tool or platform to set up and conduct your experiment. This may involve modifying website elements using web development tools or selecting email automation software capable of A/B testing functionality.
5. Split Traffic Randomly:
- Ensure that incoming traffic is evenly split between the different variations of the element being tested. Randomly assign each visitor or online user to one version over another to minimize biases.
6. Collect Data:
- Allow the test to run for a sufficient duration so that you can collect enough data to make statistically significant conclusions. Gather information on user behavior, engagement, conversion rates, and other relevant metrics.
7. Analyze Results:
- Evaluate the data collected during the A/B test and assess the statistical significance of the findings. Identify any trends or patterns by comparing conversion rates, engagement levels, and other relevant metrics among the different variations.
8. Draw Conclusions:
- Based on the analysis, draw conclusions regarding the performance of each variation tested. Determine which version performed better in achieving your predefined objective. You may find that one variation outperforms others significantly or that no statistically significant differences exist.
9. Implement Winner:
- After identifying a winning version, implement it fully into your marketing strategy. Replace the original element with the variant that demonstrated better performance based on your objective.
10. Continue Testing Iteratively:
- A/B testing should be an ongoing process to maximize optimization potential. Keep testing new ideas and variations to continuously refine your marketing strategies and improve conversion rates over time.
By integrating A/B testing into your marketing strategy using this step-by-step guide, you can make data-driven decisions and optimize your efforts to achieve better results. Remember to take a systematic approach, be patient, and keep iterating based on insights gained from each test.Overcoming challenges in Multi-Variate (MVT) vs. A/B testing comparisonsOvercoming challenges in Multi-Variate (MVT) vs. A/B testing comparisons can be a topic of great importance when it comes to optimizing and testing various elements of a website or application. Both methods have their own set of advantages and challenges, but understanding these challenges can help you make informed decisions for your testing strategy. Here are some points to consider:
A/B Testing challenges:
- Statistical significance: A key challenge in A/B testing is ensuring that the results are statistically significant. Testing variations on a website or application with a smaller sample size might skew the results and render them inconclusive.
- Limited scope: A/B testing usually focuses on testing one variable at a time, which means it might take longer to reach conclusive results, especially if changes result in small shifts in user behavior.
- Inefficient for interactions: If the changes being tested involve multiple elements or interactions across different user paths, A/B testing might not efficiently capture the full impact of those changes.
Multivariate Testing (MVT) challenges:
- Complexity: Compared to A/B testing, MVT can be more complex due to the larger number of variables being tested simultaneously. Designing MVT experiments requires careful planning and analysis to ensure accurate interpretation of results.
- Sample size requirements: MVT may often require a larger sample size compared to A/B testing in order to achieve statistical significance across multiple combinations of variables being tested.
- Test duration: As MVT involves multiple variables interacting with each other, determination of real impact and performance may take longer than traditional A/B tests.
Solutions and considerations:
- Clear goals: Before choosing between MVT and A/B testing, clearly define your goals. Determine which specific elements or interactions you want to optimize.
- Prioritization: Prioritize your experiments based on their potential impact. This will help you decide whether an A/B test is feasible for smaller changes or if MVT is better suited for larger, more complex changes.
- Strategic combination: Sometimes, a combination of both MVT and A/B testing can be beneficial. For significant changes, start with an MVT to get an understanding of interactions and initial insights. Then, follow it up with focused A/B tests to validate specific changes or modifications.
Ultimately, overcoming challenges in Multi-Variate (MVT) vs. A/B testing comparisons requires a thorough understanding of your specific optimization goals, careful planning and execution, as well as a data-driven approach to draw meaningful conclusions and make informed decisions about optimizing your website or application.Measuring and Interpreting Results from Your A/B Tests CorrectlyMeasuring and interpreting results from your A/B tests correctly is of utmost importance to make informed decisions and optimize your strategies. A/B testing is a valuable tool that allows you to compare two versions (A and B) of a variable, such as a webpage, email campaign, or advertisement, and determine which one performs better.
When conducting an A/B test, it's crucial to establish clear goals and hypotheses. Determine the specific metrics or key performance indicators (KPIs) you want to measure, such as conversion rate, click-through rate, or revenue. These goals will help you assess the effectiveness of each version.
Once the A/B test is launched and runs for a sufficient duration, it's time to analyze the results accurately. Start by examining the statistical significance of the findings. Statistical significance indicates the likelihood that any observed differences in results are not due to chance.
You can use statistical hypothesis testing techniques such as t-tests or chi-square tests to evaluate the significance of differences between the control group (version A) and experimental group (version B). This analysis will help you understand if the variations observed in the results are reliable or simply due to random fluctuations.
However, significance alone is not enough; you also need to consider practical significance. Practical significance refers to whether the observed differences have any meaningful impact on your target metrics. Even statistically significant changes may not necessarily be significant in practical terms. Always consider the magnitude of the effect when interpreting results.
Another essential point is not to prematurely stop an A/B test based on early positive or negative trends. Give your test enough time to collect sufficient data for accurate analysis and avoid drawing conclusions too soon. However, if there are significant differences early on that align with past knowledge and expectations, it might be worthwhile stopping the test after ensuring statistical rigor.
Additionally, segment your audience when interpreting results to gain deeper insights into user behavior. Analyze how different segments performed under each variant separately. It helps reveal whether a differential impact exists among specific segments and may support personalized strategies.
Keep in mind that A/B testing is an iterative process. Learn from each test, apply the knowledge gained to subsequent iterations, and refine your strategies continuously based on solid data-driven insights. Aim for gradual improvements rather than expecting dramatic changes overnight.
Lastly, never neglect qualitative feedback to complement your quantitative data. Collect user feedback through surveys, interviews, or usability tests to uncover insights that quantitative analysis alone may miss. Such qualitative data provides context and adds depth to the interpretation of your A/B test results.
In conclusion, proper measurement and interpretation play a critical role in A/B testing. Establish clear goals, analyze statistical and practical significance, ensure sufficient sample size and testing duration, segment the audience, learn from previous tests, and consider both qualitative and quantitative factors. These practices will improve the accuracy of your interpretations and drive more effective decision-making for your website optimization or marketing efforts.
A/B testing is an essential component of a data-driven marketing strategy. It allows businesses to experiment with different variations of a webpage, email campaign, or advertisement to identify which version performs better. By comparing two versions – A and B – marketers can make informed decisions backed by quantitative insights. To grasp the fundamentals of A/B testing, consider the following key points:
1. Objective: Before embarking on an A/B test, it is crucial to define a clear objective. Whether it's increasing click-through rates, improving conversions, or enhancing user engagement, a specific goal will shape the entire test.
2. Hypothesis: Based on your objective, develop a hypothesis – an educated guess about which variation will achieve the desired outcome. This forms the foundation for your A/B test and provides a direction for analysis.
3. Variables: Identify the variables you want to test within your content or interface, such as headline, call-to-action button color, layout, or pricing structure. Choose one variable at a time for accurate measurement and conclusive results.
4. Test Groups: Split your audience into two randomly assigned groups – one exposed to the control version (A) and the other to the variation (B). It is crucial to ensure both groups are representative and equal in size to draw meaningful conclusions.
5. Duration: Determine the appropriate duration for your A/B test based on sufficient sample size and statistical significance. Running tests too briefly might not provide reliable results, while excessively long tests can delay decision-making processes.
6. Measurement Metrics: Define which metrics you will track during the testing phase to measure performance accurately. These metrics could include click-through rates, conversion rates, bounce rates, form completions, or revenue generated.
7. Statistical Analysis: Once the test is complete, conduct statistical analysis on the accumulated data to ascertain any significant differences between versions A and B of your content or interface. Statistical significance helps determine if any observed variations are reliable or merely random results.
8. Learnings and Insights: Analyze the test results to uncover insights on user behavior, preferences, and their impact on conversions or engagement. Learning from every A/B test gives valuable guidance for future optimization strategies.
9. Documentation: Record and organize your A/B testing processes, including hypotheses, variables tested, metrics measured, and results obtained. This documentation enables an informed understanding of previous tests and establishes a reproducible framework for future experiments.
10. Iterative Testing: A/B testing is an ongoing process; it should be embraced as an iterative practice aimed at continuous improvement. By consistently experimenting with different ideas, marketers can steadily refine their content or interfaces to obtain optimal results.
11. Factors to Consider: Lastly, keep in mind various factors while conducting A/B tests, such as traffic volume, target audience segments, potential biases, test frequency, and the limitations of platforms or tools used for testing.
By understanding these fundamentals of A/B testing, marketers can make well-informed decisions driven by data rather than assumptions. This empirical approach empowers businesses to optimize their digital presence, enhance user experiences, and achieve better outcomes in a constantly evolving market landscape.
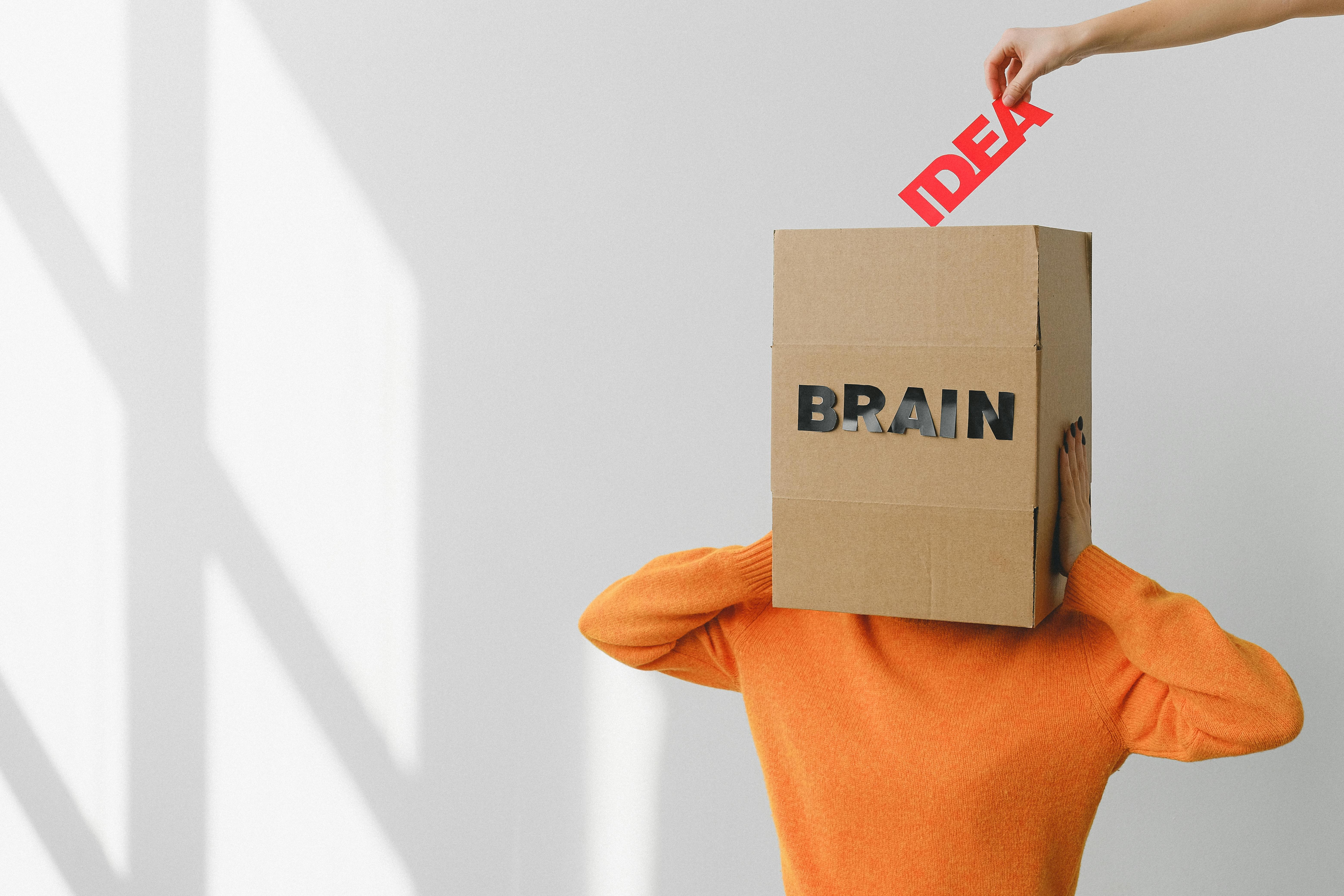
The primary goal of A/B testing is to compare variations in real-world scenarios and identify which one produces statistically significant results. This testing technique allows organizations to understand how changes like different button colors, altered layouts, revised headlines, or even modified pricing strategies can impact conversions, click-through rates (CTRs), engagement levels, or other key performance indicators (KPIs).
To conduct an A/B test, companies need to create two versions of their webpages (A and B) that differ only in the specific element or feature they want to evaluate. Elements commonly tested include design aspects, content positioning, call-to-action (CTA) wording, navigation menus, and more. Randomly dividing visitors into two groups (with an equal audience size) ensures unbiased results.
To measure the performance of these two versions accurately, it's imperative to define clear conversion goals and decide which metrics to observe closely during the test. Whether it be newsletter sign-ups, purchases made, or time spent on a page, having predefined objectives and metrics allows for better interpretation of the test results.
One essential principle in A/B testing is conducting experiments over a certain period to ensure diverse user behaviors and minimize external factors that could influence the outcomes. Seasonal trends or special promotions might affect user behavior disproportionately if the testing period is too short.
The success of an A/B test hinges on the accurate collection and analysis of reliable data. Fortunately, there are numerous tools available for implementing A/B tests efficiently. These tools assist businesses in monitoring user interactions and generating reliable statistics, ensuring statistical significance and reliable conclusions.
While A/B testing can significantly enhance user experience and drive conversion rates, it is crucial to understand that it is an ongoing process. Once a test yields conclusive results, companies should implement the preferred version and continue iterating to find further improvements. Regularly conducting A/B tests allows businesses to stay up-to-date with user expectations and preferences, ensuring their websites or apps continuously evolve for optimum user satisfaction.
In conclusion, A/B testing is a valuable technique that enables businesses to make informed decisions based on actual user feedback. By implementing this method effectively, companies can optimize their digital assets and enhance the overall user experience, leading to increased engagement, conversions, and measurable success.Balancing Cost vs. Benefit in A/B Testing StrategiesBalancing Cost vs. Benefit in A/B testing Strategies
A fundamental aspect of conducting successful A/B testing is finding the delicate balance between the costs and benefits associated with implementing and running your experiments. In this process, you strive to optimize your outcomes while maintaining a reasonable expenditure of resources. Here's what you need to know about balancing cost versus benefit in A/B testing strategies.
1. Defining Costs:
Costs in A/B testing refer to the resources you invest, such as time, money, manpower, and technology. Identifying and estimating the upfront and ongoing costs involved will allow you to make informed decisions throughout the testing process.
2. Understanding Benefits:
Benefits encompass the positive impact or uplift achieved through effective A/B testing. These benefits vary depending on your objective, whether it involves increasing conversion rates, improving user experience, boosting revenue, or achieving other key performance indicators for your business.
3. Finding the Right Balance:
A successful A/B testing strategy requires finding an equilibrium that maximizes benefit while keeping costs under control. This balance can be approached from two perspectives: cost control and benefit maximization.
4. Cost Control:
To control costs effectively, start by carefully crafting your hypotheses and identifying areas of focus to ensure you're not wasting resources on irrelevant variables. You can also prioritize test ideas based on their potential impact and feasibility in order to allocate resources wisely.
5. Ensuring Statistically Significant Results:
Minimizing costs means conducting tests long enough to gather statistically significant results without unnecessarily prolonging them. This requires estimating sample sizes accurately with statistical confidence levels in mind.
6. Simplicity and Iteration:
Keeping tests simple can help reduce costs and speed up iterations. Complex A/B tests may require more extensive engineering effort or additional technological resources, which may not always be justifiable. Adopting an iterative approach where you continually learn from previous experiments can maximize benefits without unnecessary expenses.
7. Analyzing Results Efficiently:
Perform detailed post-test analyses to extract meaningful insights without wasting time or resources. Prioritize the analysis of metrics directly linked to your main objectives, ensuring that any actionable findings can be exploited to your advantage.
8. Strategic Roadmapping:
Balance cost and benefit by having a well-defined roadmap that outlines the order and priority of tests. This type of strategy can prevent overlapping experiments, optimize resource allocation, and provide a clear path for achieving desired outcomes.
9. Continuous Monitoring and Adaptability:
Constantly monitor your A/B tests to detect any significant deviation from your expectations or emerging trends. This allows for timely adaptation and minimizes costs related to chasing false positives or negatives.
10. Learning from Failed Tests:
Failed A/B tests can also generate valuable knowledge. Instead of considering them as a complete loss, analyze them carefully to understand why they failed and how you can refine future experiments based on the lessons learned.
In conclusion, balancing the cost versus benefit in A/B testing strategies is essential for optimizing outcomes within the allocated resources. By controlling costs, ensuring statistically significant results through efficient test setups, emphasizing simplicity, analyzing results strategically, and continuously learning from experiments, you can strike the right equilibrium between cost-effectiveness and the achievement of desired benefits.Navigating the Pros and Cons of A/B TestingA/B testing is a vital component of the testing and optimization process for any website or digital marketing campaign. It involves comparing two versions of a webpage or an ad to determine which one performs better in terms of engagement, conversions, or other predefined goals.
On the positive side, A/B testing allows businesses to make data-driven decisions. By splitting traffic between two variations and measuring user behavior, companies can obtain valuable insights into customer preferences. This data serves as evidence for implementing changes that have a high probability of generating positive results.
One major advantage of A/B testing is that it helps optimize user experience. By analyzing user interactions on different versions, businesses can fine-tune their websites or applications by making changes that resonate better with their target audience. Whether it's adjusting button placements, color schemes, or font sizes, A/B testing allows for continuous improvement and enhances overall user satisfaction.
Another pro is that A/B testing helps improve conversion rates. By understanding which version of a webpage attracts higher engagement and a greater number of conversions, businesses can adapt their strategies accordingly. This approach significantly reduces the risk involved in making site-wide changes based solely on assumptions or guesswork.
Additionally, A/B testing enables companies to explore creative avenues by experimenting with different design elements, content layouts, or even promotional messages. By monitoring user response to these variations, businesses can identify innovative and effective ways to communicate their offerings and capture the attention of potential customers.
However, there are some cons and challenges to consider when embarking on A/B testing. One limitation is that significant traffic volumes are required to achieve statistically significant results. When dealing with low-traffic websites or specialized niche markets, obtaining actionable insights from A/B tests becomes more challenging due to decreased sample sizes.
Time constraints can also pose a drawback. Properly planning and executing an A/B test demands adequate time and resources. From formulating hypotheses to designing variations, setting up tracking mechanisms, acquiring statistically sound samples, and analyzing results, the process requires patience and diligence. Rushed or poorly designed tests might yield inconclusive or misleading findings.
Conditions may also change over time. Visitors' preferences and behavior can evolve, rendering previously successful A/B testing results irrelevant or outdated. Continuous monitoring and retesting become necessary to ensure ongoing optimization.
Another potential drawback is that A/B testing can lead to a conservative mindset, limiting innovation. Relying solely on incremental changes based on past data might hinder exploration of entirely new ideas or strategies that have yet to be proven.
Despite these challenges, navigating the pros and cons of A/B testing intelligently allows companies to harness its benefits successfully. A data-informed decision-making process, combined with creativity and sound judgment, paves the way for continued improvement of user experiences, increased conversions, and more effective marketing efforts overall.Real-Life Success Stories: Transformative Impact of A/B Testingtestimonials of individuals or companies achieving remarkable success through A/B testing are proof of its transformative impact. Through analyzing user behavior and manipulating variables, A/B testing evaluates different versions of a webpage or app to determine which performs better in terms of conversions, engagement, or revenue generation.
One such success story involves a popular e-commerce company. By implementing A/B testing to modify their checkout process, they significantly increased their conversion rates. By carefully testing iterations and analyzing user feedback, they were able to refine the purchasing experience, remove potential friction points, and boost their overall sales.
Another example is a content-based website that relied heavily on advertising revenue. They utilized A/B testing to optimize ad placement, format, and design. This exploration resulted in notable increases in click-through rates (CTRs) and revenue per page view. By continuously experimenting with different arrangements and studying analytical data, they ultimately reached a more effective and profitable advertisement setup.
A mobile app development company utilized A/B testing to improve user engagement and reduce churn rate. They experimented with various push notification strategies and designs to entice users to take certain actions within the app. After analyzing test results and learning from user preferences, they managed to personalize notifications while minimizing annoyance, leading to substantially higher app usage and reduced customer churn.
In yet another instance, a large retailer implemented A/B testing on their search algorithm to enhance their website's functionality. By conducting tests on alternative search result layouts and algorithms, they improved search accuracy and relevance. This overhaul resulted in customers finding products more efficiently, driving up overall sales and customer satisfaction levels.
Notably, a subscription-based service was facing a significant drop-off rate during the onboarding stage. Upon implementing A/B testing to explore different registration forms and modifications, they successfully decreased churn and achieved higher user retention rates. Refined onboarding experiences not only retained more users but also guided them toward successful outcomes within the platform.
Each of these real-life success stories highlights the power of A/B testing to revolutionize business operations. By diligently experimenting, measuring, and adapting, these companies transformed their conversions rates, engagement metrics, revenue figures, and overall success levels.
In conclusion, A/B testing has a transformative impact on businesses by unlocking their full potential through data-driven decision-making. Companies can learn from these success stories and implement similar strategies to identify areas of improvement, tailor experiences to individual preferences, and drive both customer satisfaction and financial gains.
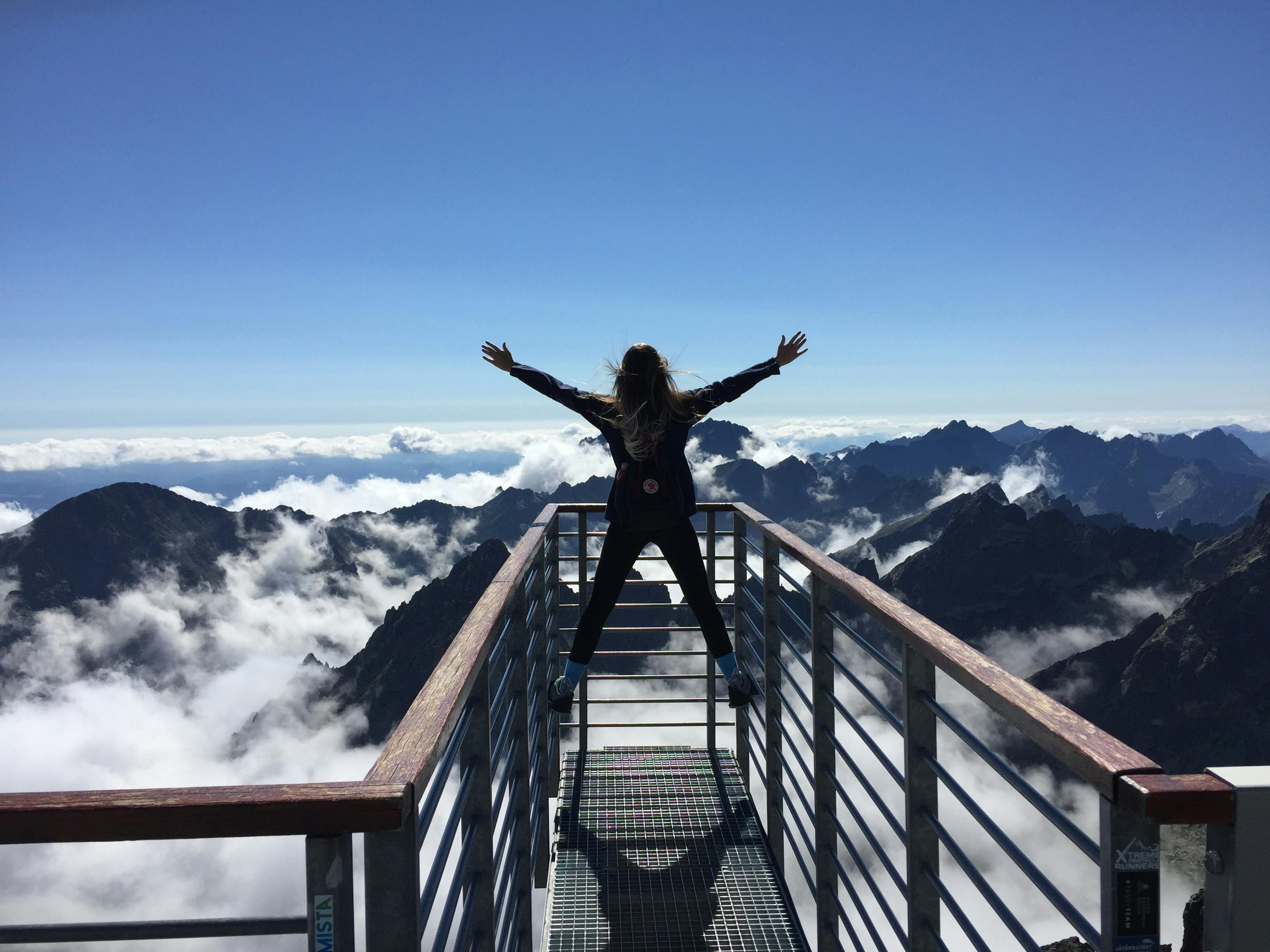
At its core, A/B testing involves the creation of two or more variants (A and B) to test against each other. These variants typically differ in a single variable, such as website layout, call-to-action buttons, or email subject lines. The goal is to determine which variant performs better by comparing the results from both groups.
Data lies at the heart of A/B testing. A variety of metrics can be tracked and analyzed depending on the nature of the experiment. This can include conversion rates, click-through rates, bounce rates, average revenue per user, and many others. By quantifying these metrics for each variant and analyzing the data collected, businesses gain valuable insights into the effectiveness of their changes.
A/B testing delivers reliable and tangible results that eliminate speculation and assumptions. Through data collection and analysis, it provides objective evidence for decision making. It allows companies to understand how changes impact user behavior and overall performance, leading to optimized strategies with proven outcomes.
This scientific approach fosters iterative improvement since A/B testing should be an ongoing process rather than a one-time event. By constantly refining and experimenting with different variations, businesses strive for continuous success through incremental enhancements.
Moreover, A/B testing democratizes decision making. Instead of relying solely on intuition or biases from key stakeholders, organizations can rely on solid evidence provided by statistical analysis. This reduces internal dispute and supports a culture of data-driven decision making within companies.
Ethical considerations play a crucial role in utilizing A/B testing effectively. Ensuring proper consent and transparency with users involved in experiments is essential. Respecting privacy concerns and ensuring data protection should be prioritized when designing, conducting, and analyzing A/B tests.
Overall, A/B testing underscores the importance of data-driven decision making for businesses striving for success. It allows companies to make informed choices based on evidence-backed results, leading to optimized strategies and improved outcomes. By democratizing decision making and driving iterative refinement, A/B testing contributes substantially to a company's growth and achievement objectives.
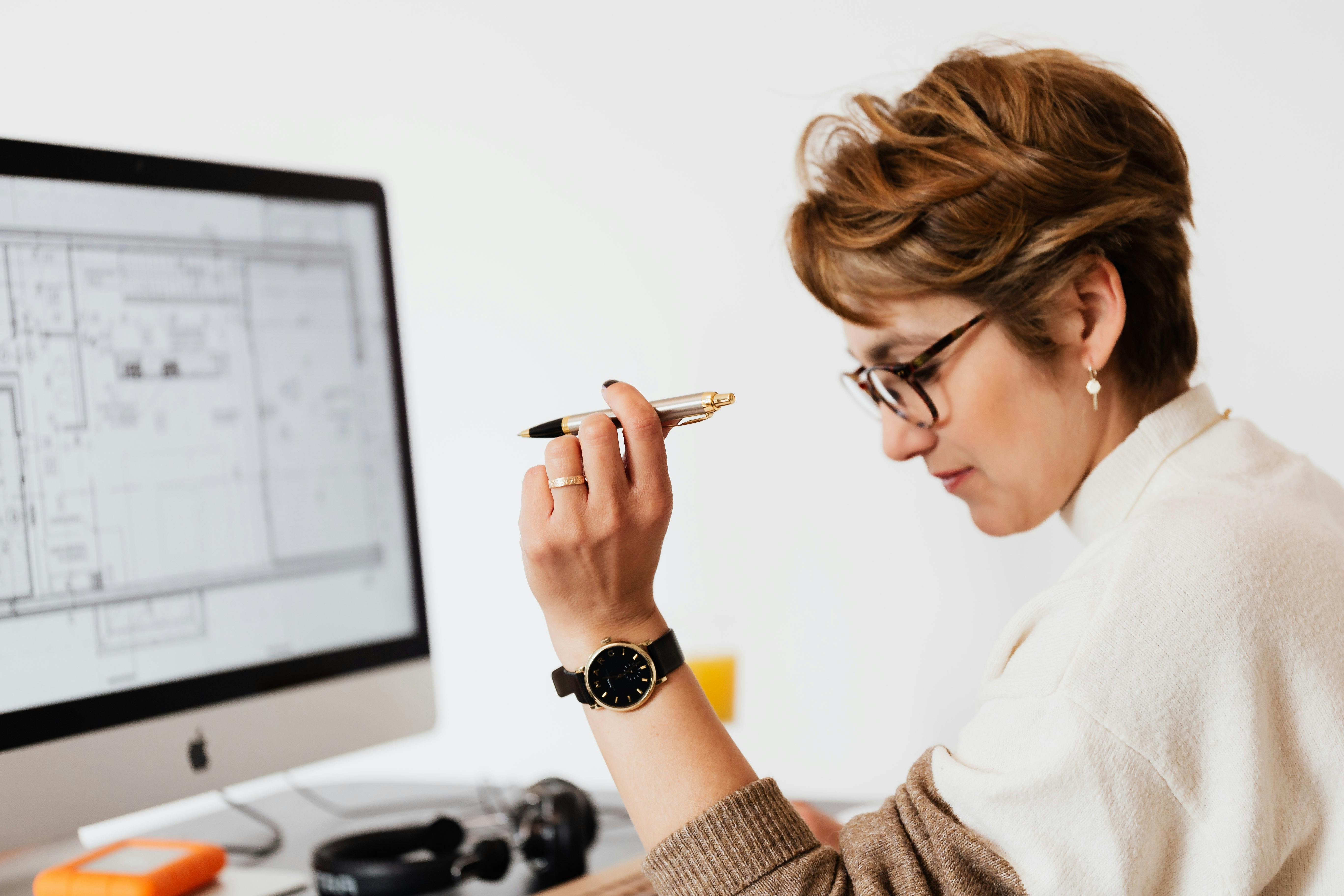
Introduction:
A/B testing campaigns can be highly effective in optimizing marketing strategies by leveraging data-driven insights. However, these campaigns can easily fall prey to common pitfalls, leading to inaccurate results and potentially detrimental decisions. In this blog, we will discuss vital tips to help you avoid these common pitfalls and make the most out of your A/B testing campaigns.
1. Insufficient Sample Size:
When conducting an A/B test, it is crucial to ensure that your sample size is sufficiently large for statistically significant results. Insufficient sample sizes can result in unreliable outcomes that may not accurately represent your target audience's preferences, leading to flawed conclusions and decision-making.
2. Testing Multiple Variables Simultaneously:
Testing more than one variable at a time within a single A/B test often arises as a significant pitfall. This approach hinders effective analysis since it becomes challenging to attribute changes in outcomes to specific variables. To generate meaningful insights, it is best to focus on a single variable while keeping other elements constant.
3. Randomization Failure:
Failure to implement randomization properly can introduce biases into your A/B tests. Random assignments ensure that each group has an equal likelihood of being exposed to different variants, making the results fair and reliable. Failing to adhere to this principle may yield skewed or false conclusions.
4. Misinterpreting Results:
Analyzing data from an A/B test requires a thoughtful approach. Rushed or biased interpretations can adversely impact decision-making processes. Remember that correlation does not always imply causation, so exercise caution when deriving insights from your results.
5. Ignoring Long-term Impact:
A successful A/B testing campaign should account for potential long-term implications of changes made during the testing phase. It may be essential to examine different metrics beyond immediate gains or losses to understand the broader effects on customer behavior, retention, or overall conversion rates.
6. Overlooking Contextual Factors:
A/B testing campaigns conducted in isolation, disregarding broader contextual factors, may not deliver accurate results. External factors like seasonality, holidays, or other ongoing marketing initiatives can significantly influence user behavior, rendering your A/B test conclusions misleading. Considering these factors is crucial for obtaining meaningful and actionable insights.
7. Prejudiced Sample Selection:
During participant selection for your A/B tests, avoiding biased subject recruitment is vital. Biases stemming from demographic imbalance or selective sampling can taint your test's validity by introducing unique characteristics that are not representative of the target audience. Strive for randomness to avoid skewed outcomes.
8. Premature Conclusions:
Resisting the urge to reach premature conclusions during an A/B testing campaign is crucial. It is advisable to wait for tests to run their full course and collect sufficient data before drawing definitive results. Premature decisions based on limited data can lead to suboptimal strategies and missed opportunities.
Conclusion:
A/B testing can be a potent tool, aiding marketers in refining tactics and strategies to enhance user engagement, conversions, and overall business growth. By considering these common pitfalls and adopting best practices, you can enjoy greater success in your A/B testing campaigns and leverage them effectively to boost your marketing efforts. Remember, compelling insights come from careful execution and unbiased interpretation of results.
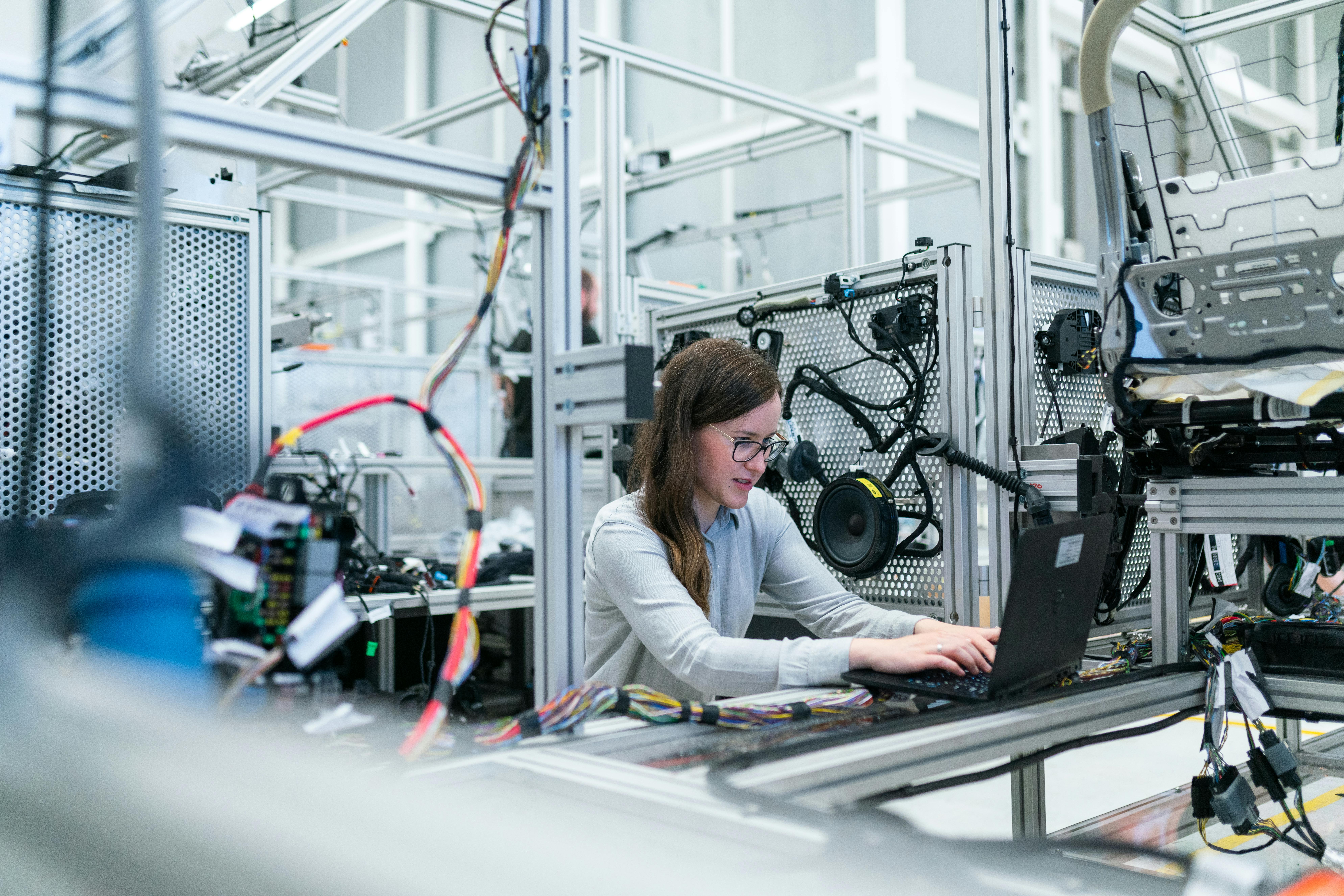
Transparency:
To ensure transparency, it is important to clearly inform users about the ongoing A/B test. Communicate how their data will be used, what changes they might notice, and their ability to opt-out if desired. By providing this information, users become aware of their participation in an experiment rather than being subjectively tested without their knowledge.
Informed Consent:
Prioritize informed consent by making sure users understand the purpose and potential impact of the A/B test before participating. Obtain explicit permission from users to include them in any such experimental procedures. By respecting users' autonomy and allowing them to choose whether to participate or not, you maintain ethical standards.
Minimal Risk:
A/B tests should ideally pose minimal risk to participants’ privacy and well-being. Minimize the collection of personally identifiable information (PII) unless necessary for the experiment. Always ensure that data collected is treated with the utmost confidentiality, protected from unauthorized access or misuse.
Avoidance of Harm:
Prevent any harm resulting from the A/B testing process. Monitor the potential adverse consequences of any changes being evaluated and take measures to mitigate negative impacts on users. Assess and minimize disruption or frustration caused by altered user experiences.
Data Protection:
Protect user data by utilizing appropriate security measures to prevent unauthorized access, manipulation, or sharing of sensitive information. Anonymize user data whenever possible while preserving the integrity of the experiment results.
End-to-End Encryption:
Employ end-to-end encryption when transmitting user information during A/B tests to ensure data security. Use secure protocols and established encryption techniques to safeguard user privacy and data.
Fairness:
Ensure fairness when designing A/B tests by distributing changes and exposures evenly across different user segments, preventing an unjust disadvantage for certain individuals or groups. Avoid discriminatory practices or biased outcomes that could negatively impact certain sections of your user base.
Data Deletion:
When an A/B test is finished, delete unnecessary user data collected during the experiment promptly. Only retain anonymized information essential for analysis and interpretation of results while adhering to applicable data protection regulations.
Clear Impact Assessment:
Always assess and analyze the potential impact of each A/B test beforehand. Consider the ethical implications, consider alternatives, and predict possible consequences before implementing any changes. Regularly evaluate the ongoing experimentation processes to address emerging ethical concerns.
Continuous Improvement:
Maintain an ongoing commitment to ethical considerations in your A/B testing practices. Continuously review and upgrade your privacy policies, data handling processes, and consent mechanisms based on evolving regulations, industry standards, and input from human rights advocacy groups.
By incorporating these ethical practices into your A/B testing routines, you can promote trust with users, safeguard their privacy, and provide fair experiences while collecting valuable insights for improving business outcomes.
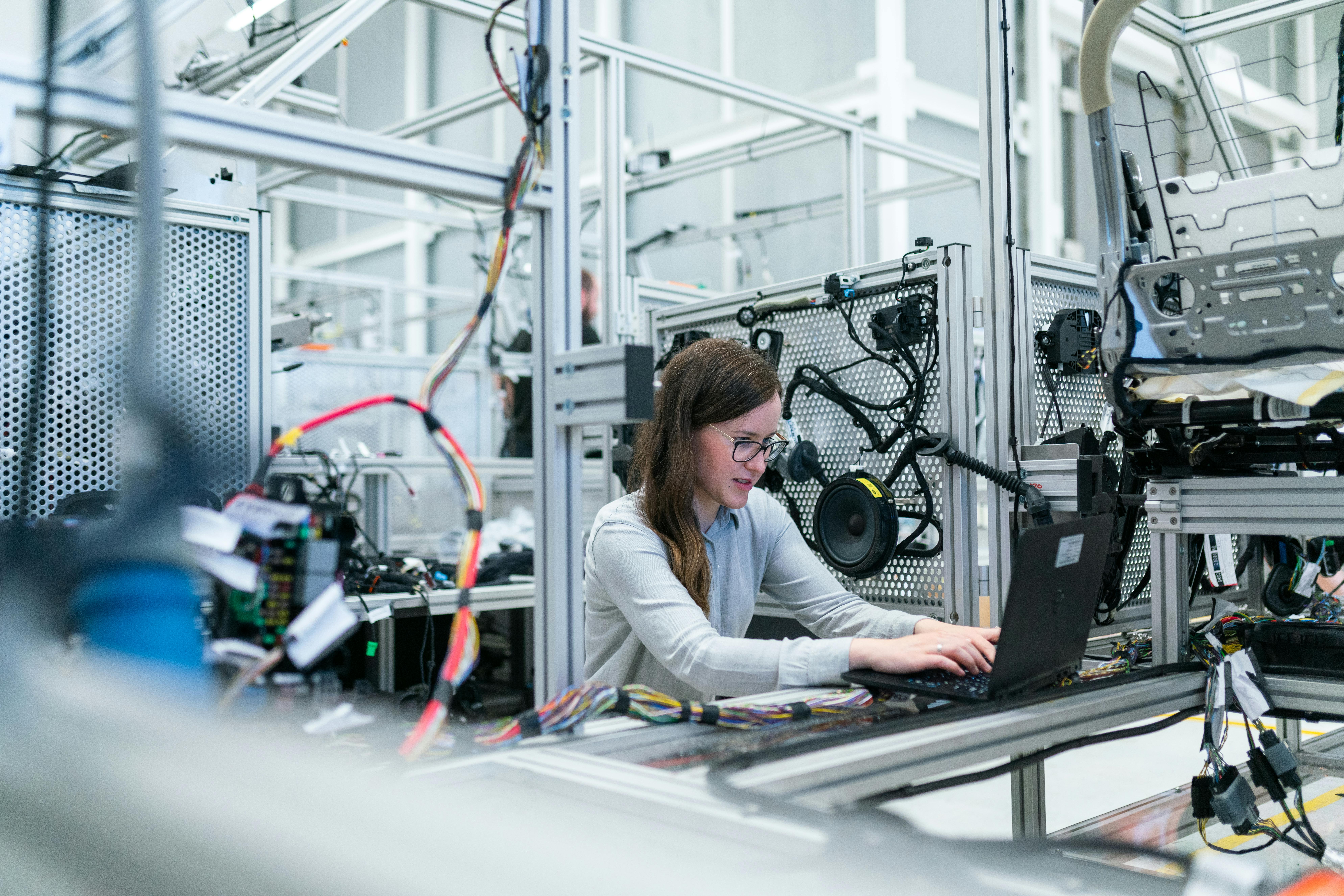
One commonly-used tool is Google Optimize, a web testing and personalization platform. It integrates seamlessly with Google Analytics, presenting a user-friendly interface that simplifies the creation and execution of A/B tests. With its editor mode, marketers can make changes directly on the website without the need for coding skills.
Optimizely is another popular A/B testing tool utilized across various industries. Its powerful features enable businesses to experiment product improvements, test different user experiences, and optimize content performance effortlessly. The platform supports multivariable testing, allowing teams to examine multiple variations at once.
VWO (Visual Website Optimizer) is a versatile A/B testing software known for its dynamic and comprehensive functionalities. This tool encompasses heatmaps, click maps, and advanced targeting options, enabling marketers to deep dive into user behavior. Alongside its seamless integration, VWO offers quick test setup through a visual editor.
Adobe Target combines A/B testing capabilities with advanced personalization features. This technology equips marketers with the ability to target specific audience segments using AI-enhanced algorithms intelligently. Adobe Target provides mobile app testing as well, ensuring optimization across all platforms.
Dynamic Yield specializes in employing personalization techniques alongside A/B testing. This all-encompassing platform captures user data in real-time to deliver tailored experiences at scale. Its smart algorithms allow businesses to automatically adapt content based on users' preferences, boosting conversions significantly.
Lastly, Mixpanel's A/B Testing functionality empowers organizations to experiment and iterate on different features or user journeys. This technology enables targeted messaging to specific user cohorts and supplies valuable insights into engagement metrics. Additionally, Mixpanel facilitates trend analysis and funnel tracking, guiding companies towards high-impact optimizations.
In conclusion, A/B testing tools and technologies play an integral role in streamlining the optimization process. These innovative platforms, such as Google Optimize, Optimizely, VWO, Adobe Target, Dynamic Yield, and Mixpanel, simplify complex tests while providing elaborate insights to enhance customer experiences and drive successful outcomes.
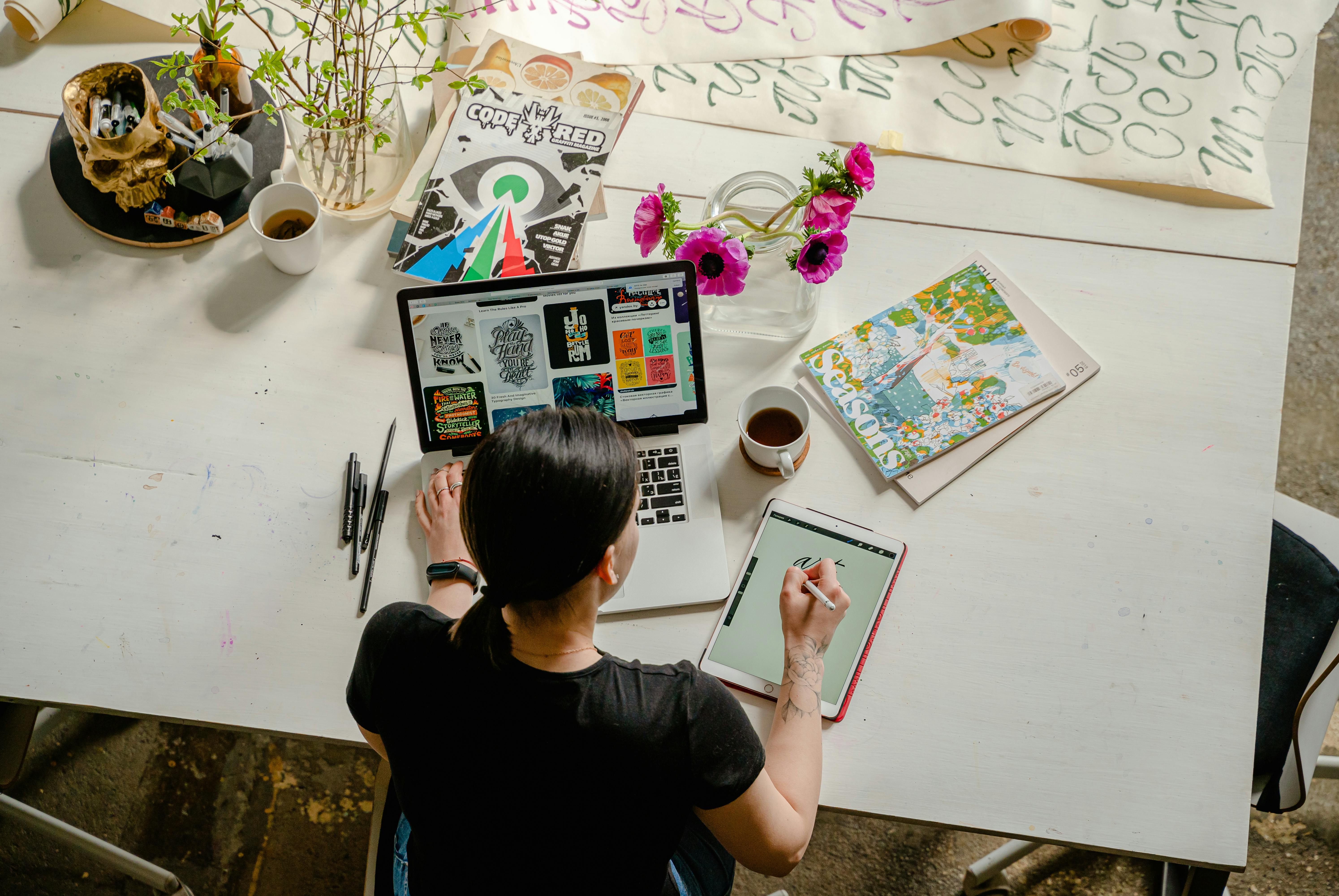
A/B testing is a valuable analytical tool used to assess and evaluate the performance of different versions of a web page or application. However, it is crucial to understand that every industry operates uniquely and has its own set of requirements. To effectively tailor A/B tests for different industries, consider the following best practices:
1. Industry-specific goals: Each industry has its specific objectives and key performance indicators (KPIs) that dictate success. Tailor your A/B tests to contribute directly to these goals. For example, an e-commerce site might focus on increasing conversions, while a media company could prioritize user engagement and time spent on content.
2. Understand your target audience: Extensive knowledge about your audience's preferences, behaviors, and biases is essential. Conduct robust market research to identify specific metrics and behaviors relevant to your industry.
3. Segmenting audiences: Depending on your industry, audience segmentation can be highly effective in tailoring A/B tests. Split users into distinct groups based on demographics, geographics, or their stage in the buyer's journey. This allows you to create targeted experiments catering to different segments.
4. Design captivating variations: The visual appeal and user experience of your website or application significantly impact conversion rates and engagement levels. Craft visually appealing variations that align with industry norms and best practices to capture users' attention effectively.
5. Consider mobile responsiveness: With expanding smartphone usage, ensure that your A/B tests cater to mobile devices as well. Test how variations in design impact the user experience across various screen sizes and operating systems commonly used within your target industry.
6. Iterative testing: Almost never settle on the first variation tested in an A/B experiment. Continuously iterate upon successful findings to refine and optimize outcomes in a rapidly evolving world.
7. Size matters...sample size! The sample size plays a vital role in achieving statistically significant results from your A/B tests. Ensure your sample size adequately represents your industry's target audience to generate meaningful and actionable findings.
8. Timing and seasonality: Take into account potential seasonal variations that might influence user behavior. Accounting for these trends could prevent inaccurate conclusions based on temporary situations.
9. Data-driven decision-making: Stay committed to making decisions based on data rather than personal opinions or assumptions. Rely on quantitative and qualitative data generated from your A/B tests to uncover insights specific to your industry.
10. Monitor long-term effects: Short-term test results may not always align with long-term impacts. Keep track of how variations affect sustainability, customer satisfaction, and overall business objectives in the long run.
11. Learn from other industries: Embrace cross-industry learning by observing successful tactics implemented by others. Often, valuable insights learned in one sector can be adapted and successfully applied to different industries.
By adhering to these best practices and tailoring your A/B tests according to specific industry requirements, you can maximize the effectiveness of your tests and accomplish desired goals in a more targeted manner.Beyond the Basics: Advanced Techniques in A/B TestingA/B testing has proven to be a useful method for understanding user behavior and making data-driven decisions. It allows businesses to test different versions of their website or app and analyze which one performs better, ultimately improving conversions and user experience. While the concept of A/B testing is fairly easy to understand, there are advanced techniques that go beyond the basics, unlocking even more insights and potential benefits.
Advanced techniques in A/B testing involve strategic approaches to optimize experiments further and get more accurate results. These techniques often require more advanced statistical knowledge and proper testing methodologies. Let's delve into some of these advanced techniques that can take your A/B testing to the next level:
1. Sequential Testing: Unlike traditional A/B testing, sequential testing allows you to make decisions before waiting for a fixed sample size. This technique helps you determine the optimal conversion rate quickly by continuously analyzing incoming data and making real-time decisions. It saves time because you can stop a test as soon as conclusive results are obtained, rather than waiting for all data points.
2. Multi-Armed Bandit Testing: This technique involves dynamically allocating traffic between different variations based on their performance during the test. Instead of running multiple equal groups simultaneously like in typical A/B testing, multi-armed bandit testing shifts the balance towards the most promising variations over time. As a result, you can mitigate potential losses caused by inferior variations during the early stages of a test.
3. Bayesian A/B Testing: Bayesian statistics looks at probability differentially before and after new data is included. This approach allows businesses to estimate conversion rates accurately with smaller sample sizes while still taking prior estimations into account. It also enables continuously updating beliefs about variations during an ongoing A/B tests, providing a refined understanding of what's working best.
4. Fractional Factorial Design: This technique allows you to reduce the number of variations required for testing multiple elements or factors within an experiment exponentially. By strategically selecting which combinations to test, you can effectively identify the main contributing factors and interactions between them with minimal resources expended.
5. Personalization and Segmentation: Advanced A/B testing considers personalizing experiences for different user segments. Rather than testing for the entire user base, you divide it into smaller segments with specific demographics or behaviors and tailor the variations accordingly. This emphasizes the idea that a one-size-fits-all approach may not be effective, as different segments may respond better to specific variations.
6. Incrementality Testing: Incrementality testing is about figuring out whether your experiment itself causes the determined change in user behavior or if external factors, such as seasonality or promotions, are responsible. By conducting controlled experiments alongside outside influences, you can accurately attribute changes to the experiment itself and assess its true impact.
Remember, advanced A/B testing techniques require careful consideration of statistical significance, sample sizes, and thorough analysis. Engaging with an experienced data scientist or expert in A/B testing can help ensure accurate implementation and interpretation of results. By leveraging these advanced techniques, you can gain deeper insights into user preferences and optimize your digital experiences effectively.
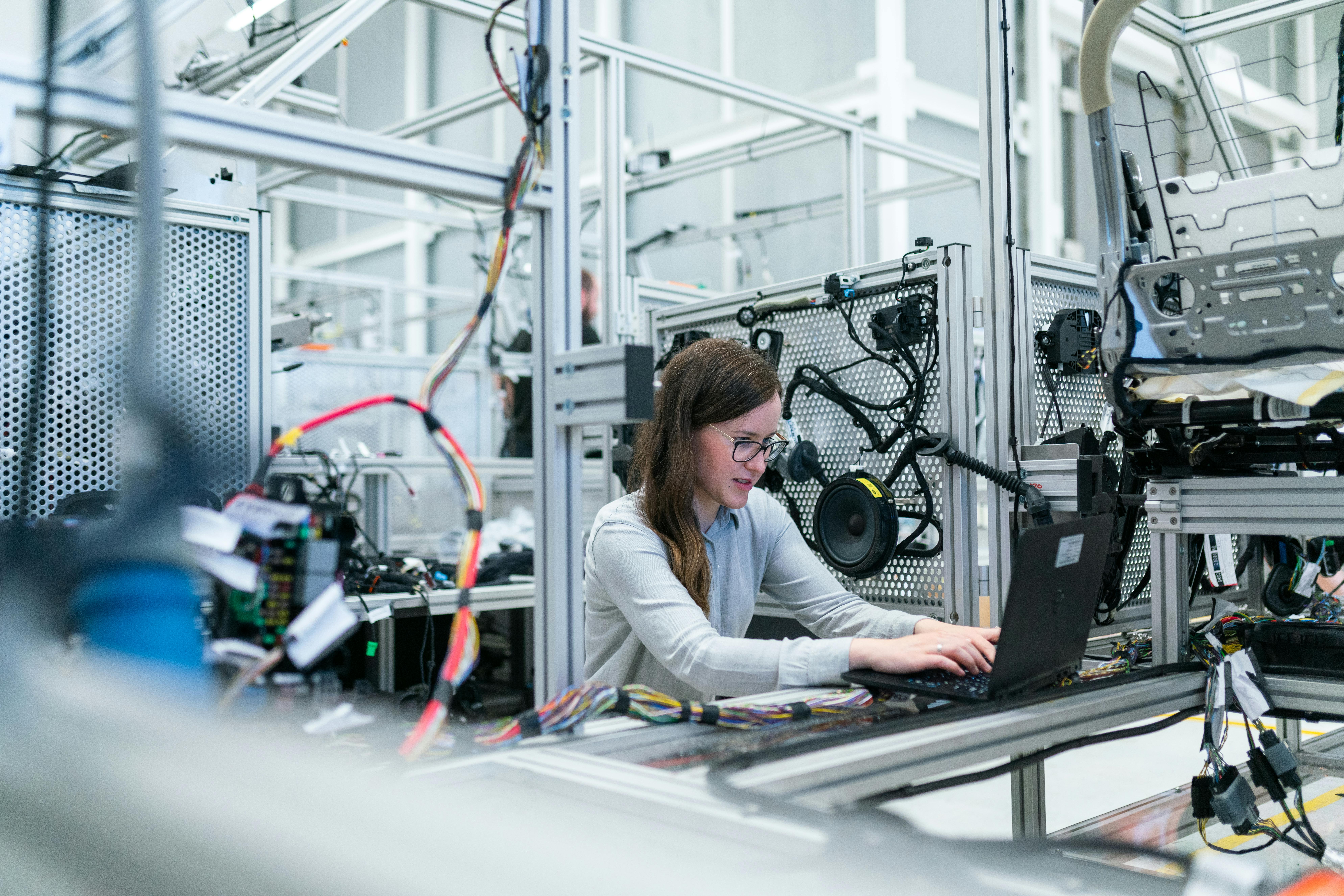
When it comes to optimizing conversion rates and fine-tuning your marketing strategies, A/B testing is a powerful tool that can make a significant impact. By comparing multiple versions of a webpage or marketing campaign, you can gather valuable data and insights to understand what works best for your audience. Here's a detailed guide on integrating A/B testing into your marketing strategy:
1. Define Your Objective:
- Begin by clearly defining your goal or objective for the test. Identify what you want to achieve and outline the metrics or Key Performance Indicators (KPIs) that will help measure success.
2. Decide on the Element to Test:
- Evaluate different elements of your marketing efforts that you believe can lead to improvement. This may include web page layouts, headlines, copywriting, design elements, call-to-action buttons, email subject lines, or even pricing structures.
3. Create Variations:
- Develop multiple variations of the element you have chosen to test. For example, if you are testing a landing page headline, create two or more alternatives that present slightly different messaging approaches.
4. Set Up the Test:
- Utilize a reliable A/B testing tool or platform to set up and conduct your experiment. This may involve modifying website elements using web development tools or selecting email automation software capable of A/B testing functionality.
5. Split Traffic Randomly:
- Ensure that incoming traffic is evenly split between the different variations of the element being tested. Randomly assign each visitor or online user to one version over another to minimize biases.
6. Collect Data:
- Allow the test to run for a sufficient duration so that you can collect enough data to make statistically significant conclusions. Gather information on user behavior, engagement, conversion rates, and other relevant metrics.
7. Analyze Results:
- Evaluate the data collected during the A/B test and assess the statistical significance of the findings. Identify any trends or patterns by comparing conversion rates, engagement levels, and other relevant metrics among the different variations.
8. Draw Conclusions:
- Based on the analysis, draw conclusions regarding the performance of each variation tested. Determine which version performed better in achieving your predefined objective. You may find that one variation outperforms others significantly or that no statistically significant differences exist.
9. Implement Winner:
- After identifying a winning version, implement it fully into your marketing strategy. Replace the original element with the variant that demonstrated better performance based on your objective.
10. Continue Testing Iteratively:
- A/B testing should be an ongoing process to maximize optimization potential. Keep testing new ideas and variations to continuously refine your marketing strategies and improve conversion rates over time.
By integrating A/B testing into your marketing strategy using this step-by-step guide, you can make data-driven decisions and optimize your efforts to achieve better results. Remember to take a systematic approach, be patient, and keep iterating based on insights gained from each test.Overcoming challenges in Multi-Variate (MVT) vs. A/B testing comparisonsOvercoming challenges in Multi-Variate (MVT) vs. A/B testing comparisons can be a topic of great importance when it comes to optimizing and testing various elements of a website or application. Both methods have their own set of advantages and challenges, but understanding these challenges can help you make informed decisions for your testing strategy. Here are some points to consider:
A/B Testing challenges:
- Statistical significance: A key challenge in A/B testing is ensuring that the results are statistically significant. Testing variations on a website or application with a smaller sample size might skew the results and render them inconclusive.
- Limited scope: A/B testing usually focuses on testing one variable at a time, which means it might take longer to reach conclusive results, especially if changes result in small shifts in user behavior.
- Inefficient for interactions: If the changes being tested involve multiple elements or interactions across different user paths, A/B testing might not efficiently capture the full impact of those changes.
Multivariate Testing (MVT) challenges:
- Complexity: Compared to A/B testing, MVT can be more complex due to the larger number of variables being tested simultaneously. Designing MVT experiments requires careful planning and analysis to ensure accurate interpretation of results.
- Sample size requirements: MVT may often require a larger sample size compared to A/B testing in order to achieve statistical significance across multiple combinations of variables being tested.
- Test duration: As MVT involves multiple variables interacting with each other, determination of real impact and performance may take longer than traditional A/B tests.
Solutions and considerations:
- Clear goals: Before choosing between MVT and A/B testing, clearly define your goals. Determine which specific elements or interactions you want to optimize.
- Prioritization: Prioritize your experiments based on their potential impact. This will help you decide whether an A/B test is feasible for smaller changes or if MVT is better suited for larger, more complex changes.
- Strategic combination: Sometimes, a combination of both MVT and A/B testing can be beneficial. For significant changes, start with an MVT to get an understanding of interactions and initial insights. Then, follow it up with focused A/B tests to validate specific changes or modifications.
Ultimately, overcoming challenges in Multi-Variate (MVT) vs. A/B testing comparisons requires a thorough understanding of your specific optimization goals, careful planning and execution, as well as a data-driven approach to draw meaningful conclusions and make informed decisions about optimizing your website or application.Measuring and Interpreting Results from Your A/B Tests CorrectlyMeasuring and interpreting results from your A/B tests correctly is of utmost importance to make informed decisions and optimize your strategies. A/B testing is a valuable tool that allows you to compare two versions (A and B) of a variable, such as a webpage, email campaign, or advertisement, and determine which one performs better.
When conducting an A/B test, it's crucial to establish clear goals and hypotheses. Determine the specific metrics or key performance indicators (KPIs) you want to measure, such as conversion rate, click-through rate, or revenue. These goals will help you assess the effectiveness of each version.
Once the A/B test is launched and runs for a sufficient duration, it's time to analyze the results accurately. Start by examining the statistical significance of the findings. Statistical significance indicates the likelihood that any observed differences in results are not due to chance.
You can use statistical hypothesis testing techniques such as t-tests or chi-square tests to evaluate the significance of differences between the control group (version A) and experimental group (version B). This analysis will help you understand if the variations observed in the results are reliable or simply due to random fluctuations.
However, significance alone is not enough; you also need to consider practical significance. Practical significance refers to whether the observed differences have any meaningful impact on your target metrics. Even statistically significant changes may not necessarily be significant in practical terms. Always consider the magnitude of the effect when interpreting results.
Another essential point is not to prematurely stop an A/B test based on early positive or negative trends. Give your test enough time to collect sufficient data for accurate analysis and avoid drawing conclusions too soon. However, if there are significant differences early on that align with past knowledge and expectations, it might be worthwhile stopping the test after ensuring statistical rigor.
Additionally, segment your audience when interpreting results to gain deeper insights into user behavior. Analyze how different segments performed under each variant separately. It helps reveal whether a differential impact exists among specific segments and may support personalized strategies.
Keep in mind that A/B testing is an iterative process. Learn from each test, apply the knowledge gained to subsequent iterations, and refine your strategies continuously based on solid data-driven insights. Aim for gradual improvements rather than expecting dramatic changes overnight.
Lastly, never neglect qualitative feedback to complement your quantitative data. Collect user feedback through surveys, interviews, or usability tests to uncover insights that quantitative analysis alone may miss. Such qualitative data provides context and adds depth to the interpretation of your A/B test results.
In conclusion, proper measurement and interpretation play a critical role in A/B testing. Establish clear goals, analyze statistical and practical significance, ensure sufficient sample size and testing duration, segment the audience, learn from previous tests, and consider both qualitative and quantitative factors. These practices will improve the accuracy of your interpretations and drive more effective decision-making for your website optimization or marketing efforts.