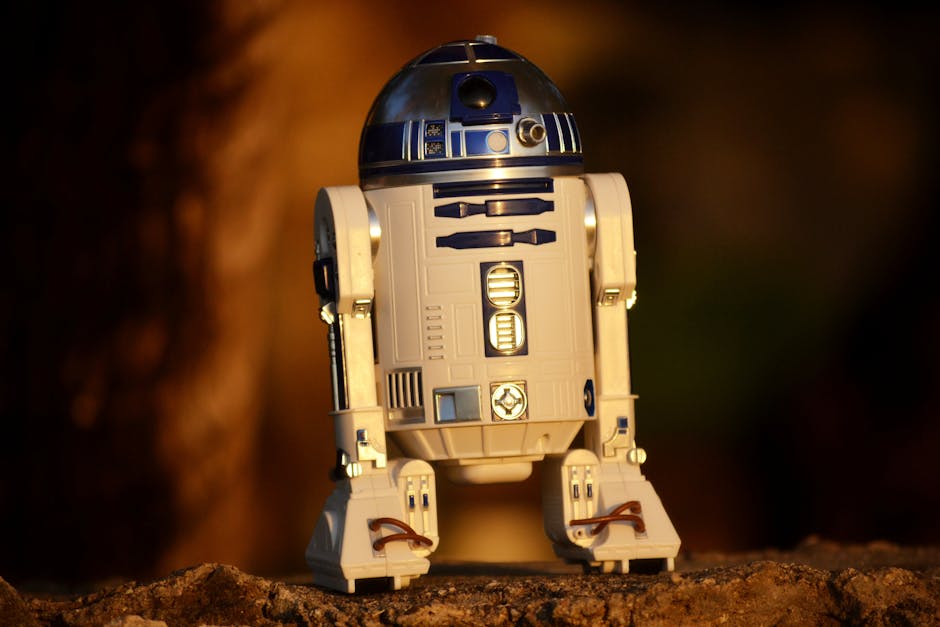
Exploring Artificial Intelligence: Unleashing the Benefits and Weighing the Pros and Cons
The Fundamental Principles of Artificial Intelligence: Understanding How Machines Learn
artificial intelligence (AI) has become an integral part of our lives, from voice assistants to autonomous vehicles. To understand how this powerful technology functions, it's essential to grasp its fundamental principles—the way machines learn. This article aims to explore these principles in plain, straightforward terms, shedding light on the mechanisms that enable AI systems to make intelligent decisions.At its core, AI is built upon a concept called machine learning—a process that allows machines to acquire knowledge and improve their performance via experience. Machine learning algorithms, inspired by human cognition, are trained on vast amounts of data to identify patterns and make predictions or decisions.
- Supervised Learning: In this popular machine learning technique, models are trained using labeled data that humans have already classified or labeled accurately. The algorithm learns by associating input data with predefined output labels until it can accurately predict labels for new, unseen inputs.
- Unsupervised Learning: In contrast to supervised learning, unsupervised learning involves training models on unlabeled data. These models aim to discover hidden patterns or structures within the data without any predetermined labels. Unsupervised learning allows machines to group similar instances together or find anomalies in a dataset.
- Reinforcement Learning: Inspired by concepts from psychology and control theory, reinforcement learning relies on models interacting with an environment and learning through feedback mechanisms such as rewards and penalties. The algorithm explores possible actions within the environment and receives positive feedback when it makes correct decisions.
- Neural Networks: Taking inspiration from the human brain's structure, neural networks are composed of interconnected artificial neurons—layers stacked upon each other. These networks learn directly from data by adjusting the strengths of connections between neurons—an ability known as "weights"—to detect complex patterns and make informed judgments.
- Deep Learning: A subset of neural networks, deep learning refers to architectures with several layers (deep layers) composed of multiple neurons. Deep learning excels at automatically extracting intricate features from raw data, enabling more accurate predictions or decisions in various domains.
- Feature Extraction: As the human brain processes sensory inputs, AI models require feature extraction to analyze and understand raw data comprehensively. By extracting essential characteristics or features, machines can effectively represent complex data patterns.
- Natural Language Processing (NLP): NLP enables AI systems to understand and generate human language. Techniques such as sentiment analysis, language translation, and text generation facilitate this process, opening doors to applications like chatbots, language assistants, and language processing tools.
- Computer Vision: This field focuses on teaching machines to interpret visual data such as images and videos. Applications range from object detection and image recognition to facial identification and autonomous vehicles' perception systems.
- Data Preprocessing: High-quality, well-prepared data is crucial for machine learning success. Data preprocessing involves tasks like removing noise, handling missing values, scaling features, encoding categorical variables, and dividing datasets into subsets for training, validation, and testing.
- Overfitting and Underfitting: These are common challenges in machine learning models. Overfitting occurs when the model performs exceptionally well on the training data but poorly on new/unseen data due to overlearning patterns peculiar to the training set. Underfitting happens when the model fails to capture the patterns in the training data and performs poorly overall.
Understanding these fundamental principles empowers us to appreciate how machines learn in AI systems. It fosters informed discussions around AI's impact on society and aids in recognizing opportunities and limitations of this transformative technology.

AI in Everyday Life: How Artificial Intelligence is Transforming Our Daily Activities
artificial intelligence (AI) has swiftly infiltrated numerous aspects of our everyday lives, revolutionizing the ways we interact, work, and play. From simple smartphone functions to complex automation systems, AI is omnipresent, seamlessly integrating into our daily activities.In communication, AI powers virtual assistants like Siri, Alexa, or Google Assistant. These intuitive applications process natural language queries and assist us in finding information, setting reminders, and even managing smart home devices. We can effortlessly send messages or make calls by simply speaking to our phones with the help of AI technologies.
Internet and social media platforms also rely heavily on AI algorithms. They analyze user behavior, preferences, and content to enhance browsing experiences. Algorithms recommend personalized news articles, movies, or products that align with our interests. Social media platforms also use AI to curate our news feeds and tailor advertising content specifically designed to entice us based on our online activities.
The convenience of online shopping owes much to AI as well. E-commerce platforms utilize intelligent algorithms to predict consumer behavior, giving rise to personalized shopping experiences. From product recommendations based on past purchases to virtual fitting rooms using augmented reality, AI allows us to have a seamless and tailored experience while simplifying decision-making processes.
AI is transforming the healthcare industry too. Medical professionals employ advanced AI-infused tools for disease diagnosis and treatment decision making. Algorithms can quickly sift through vast amounts of patient data, identifying patterns and predicting potential risks or outcomes with greater accuracy than humans alone. AI also aids in drug development by analyzing molecular structures and identifying potential drug candidates more efficiently.
Smart homes equipped with devices like smart thermostats or security systems are another facet where AI enhances daily life. These technologies adapt to homeowners' habits and preferences, automatically adjusting temperature or detecting unusual activities through pattern recognition algorithms. Such applications simplify our lives while reducing energy consumption and ensuring security.
Educational institutions have also integrated AI for personalized learning experiences. Intelligent tutoring systems adapt the curriculum based on individual student progress and learning capabilities. Virtual reality and augmented reality platforms are widely employed, creating immersive and interactive learning environments. AI can provide instant feedback to students, empowering them to learn at their own pace.
Transportation systems benefit significantly from AI advancement. From driver assistance systems that alert us to potential hazards to autonomous vehicles roaming our streets, AI optimizes both safety and efficiency in the transportation sector. Traffic management programs use AI to analyze live traffic data, predict congestion, and recommend alternate routes, thereby keeping us moving smoothly through our daily commutes.
Lastly, entertainment and media consumption have flourished thanks to AI. Content streaming services like Netflix or Spotify employ recommendation algorithms to suggest movies, shows, or music based on our preferences and previous choices. AI is also used in video games, creating increasingly advanced virtual environments and intelligent opponents that challenge gamers in innovative ways.
The scope of AI's influence is vast and diverse, underscoring its ongoing transformation of everyday life. Given its continuing progress, AI will undoubtedly continue to cement its position as an integral part of our lives, reshaping the future landscape of daily activities in unforeseen ways.
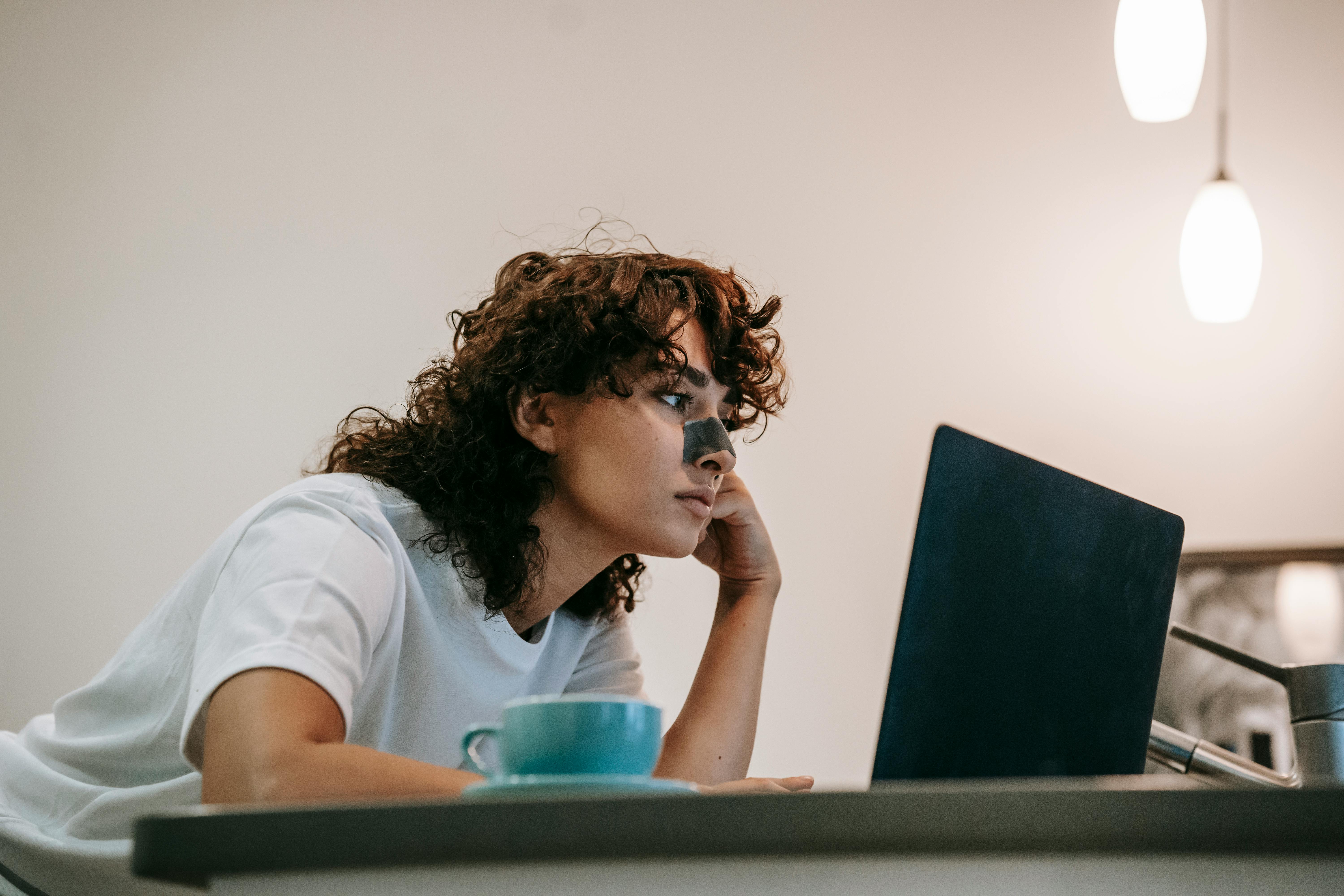
The Ethical Implications of AI: Balancing Innovation with Humanity
artificial intelligence (AI) has become an increasingly vital field with numerous applications, from automating mundane tasks to assisting in complex decision-making processes. However, the advancement of AI raises formidable ethical considerations that must be addressed. Balancing innovation with humanity is crucial to ensure its responsible development and deployment. Here are key aspects regarding the ethical implications of AI:- Privacy: AI systems often require vast amounts of data to deliver accurate results. This raises concerns related to privacy and the protection of personal information. The collection, storage, and usage of data must be done transparently, with clear consent from individuals while safeguarding their rights.
- Bias and Fairness: AI models can inadvertently inherit biases present in training data or the algorithms themselves. Discriminatory decisions stemming from such biases can perpetuate social inequalities or amplify existing prejudices. Efforts must be made to develop unbiased AI models and ensure fairness in their use by considering diverse perspectives.
- Employment Disruption: While AI opens avenues for innovation and efficiency, it also carries the potential to displace human workers. Automation may lead to job losses across various industries, necessitating measures to retrain and upskill individuals for new opportunities.
- Accountability: As machines autonomously make decisions, it becomes challenging to assign accountability for their actions. Transparency regarding how AI systems arrive at their conclusions is essential, enabling society to understand and challenge any harmful decisions made by AI algorithms.
- Human Agency: The reliance on AI systems can reduce individual autonomy if humans become solely dependent on technology for critical decision-making processes. Ensuring that humans remain in control while using AI as an aid rather than replacement is critical for preserving human agency.
- Safety and Security: The rapidly advancing field of AI creates potential risks in terms of safety and security. Malicious actors could exploit vulnerabilities in AI systems, causing harm or potentially sabotaging important infrastructures. Safeguards need to be implemented to mitigate these risks and ensure the resilience of AI systems against attacks.
- Expanding the Digital Divide: Unequal access to AI technologies could exacerbate the existing digital divide, further disenfranchising marginalized communities who lack resources or connectivity. Ensuring equitable distribution of AI benefits while addressing accessibility and affordability is imperative.
- Long-term Implications: As AI continues to evolve, potential consequences that may emerge in the future need consideration. Understanding the impact of widespread adoption, including unintended consequences and long-term effects on various aspects of society, will be crucial for navigating ethical paths.
Balancing innovation in AI with humanity requires continual dialogue among developers, researchers, policymakers, and society at large. Ethical frameworks, regulations, and international collaborations should drive responsible innovation emphasizing transparency, fairness, privacy, accountability, and societal well-being alongside technological advancement.

The Future of Employment: How AI is Shaping Job Markets and Workforces Globally
artificial intelligence (AI) is poised to revolutionize job markets and reshape workforces worldwide. With its rapid advancements, AI technology is seeping into various industries, causing both excitement and concern about its impact on employment. While the future holds tremendous potential, it also presents challenges and uncertainties.Thanks to AI's ability to automate tasks that were traditionally carried out by humans, certain jobs are at risk of becoming redundant. Repetitive, time-consuming tasks like data entry, assembly line work, or basic customer support can now be efficiently handled by intelligent machines and algorithms. As a result, jobs in these areas may significantly decline, leading to widespread automation-driven unemployment.
However, it's crucial to note that while certain roles may vanish, new ones will emerge. The rise of AI has sparked demand for professionals capable of designing, developing, and maintaining advanced AI systems. Experts predict an explosive growth in AI-related job opportunities in the near future. AI specialists will be needed across industries to implement and optimize AI solutions specifically tailored to meet unique business requirements.
Additionally, as AI changes the nature of work, there will be an increased demand for workers with skills complementary to AI technologies. Creative and critical thinking, complex problem-solving abilities, emotional intelligence, adaptability, and originality are qualities that are difficult to replicate within automated systems. Therefore, these skill sets become even more valuable as they cannot be easily replaced by machines.
Furthermore, the advent of AI can lead to significant changes in the global workforce dynamics. Emerging economies might find themselves at an advantage due to their ability to adapt swiftly and leverage AI technologies efficiently. Countries that invest in building strong educational frameworks centered around cognitive thinking skills are likely to harness the full potential of AI and compete effectively in this transformed job market.
While some predict worrisome challenges such as mass unemployment due to automation and AI's scalability potential inhibiting growth sectors that heavily rely on human labor (e.g., manufacturing), others foresee a different scenario. They envision AI augmenting human capabilities rather than merely supplanting them. Such proponents argue that human-to-AI collaboration is likely to create new job opportunities and redefine the concept of work itself.
Adapting to the future workplace will require a proactive approach. It involves reskilling and upskilling current workers to ensure they remain competitive and versatile in upcoming AI-driven industries. The education sector will have to adapt its curriculum to encompass technical skills related to AI and soft skills integral to succeeding in a hybrid human-AI workforce.
Ethical considerations are vital when discussing the future of employment and AI's influence on it. As we embrace increased automation, transparency, fairness, and human accountability should remain paramount. Ensuring that AI-based systems benefit society at large, from economic gains to improved quality of life, necessitates responsible regulations and continuous oversight over their deployment.
In summary, the future of employment is undoubtedly being shaped by artificial intelligence. Though concerns regarding job displacement persist, AI adoption presents immense potential for economic growth and can augment human capabilities. Preparing for this transformative future requires balancing technical expertise with critical thinking skills while upholding ethics and regulation as crucial guideposts. By doing so, we pave the way for harmonious integration of AI into global job markets and workforces.
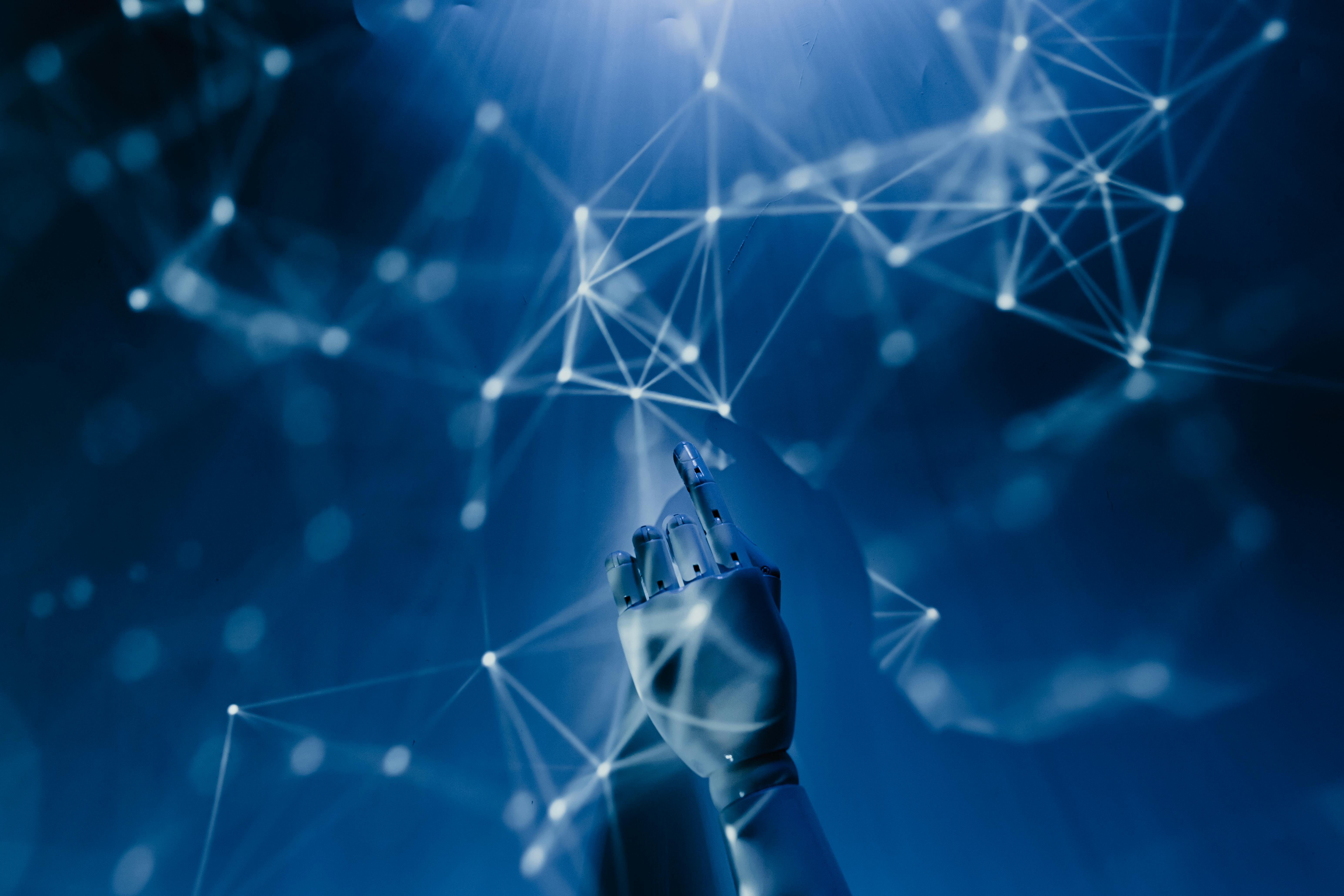
AI and Data Privacy: Safeguarding Personal Information in the Age of Intelligent Machines
artificial intelligence (AI) has gradually permeated every aspect of our lives, revolutionizing various industries and affecting how we interact with technology. However, this rapid advancement in AI also brings about significant concerns and challenges, especially pertaining to data privacy.Safeguarding personal information has become a crucial issue in the age of intelligent machines. As AI systems collect and process vast amounts of data, the need to protect individuals' privacy becomes paramount. Despite its numerous benefits, AI can pose risks when it comes to handling sensitive information.
One common concern is the potential for data breaches and unauthorized access. With the massive amount of personal data collected by AI systems, including personal preferences, behavioral patterns, and even biometric information, there is an increased risk that such data falls into the wrong hands. This could lead to identity theft, fraud, or other malicious activities.
Another challenge is the inherent bias found in AI algorithms. As these systems rely on historical data to make predictions or decisions, they might inadvertently perpetuate existing biases or discriminate against certain groups. For example, flawed facial recognition systems have disproportionately misidentified individuals from marginalized communities. Safeguarding personal information must involve addressing these biases to ensure fairness and prevent discrimination.
Besides unintentional bias, there's also the threat of deliberate misuse of personal data. Companies or organizations may attempt to exploit user information for targeted advertising, manipulation, or surveillance purposes without consent. Protecting data privacy involves formulating regulations and ethical guidelines to prevent such misuse and ensure transparency in the use of personal information.
Developing secure frameworks for AI systems is essential in safeguarding data privacy. This includes implementing robust encryption methods during data collection, storage, and transmission processes to protect sensitive information from unauthorized access. Employing techniques like differential privacy can help anonymize individual datasets while still allowing meaningful analysis on aggregated data.
One possible approach that strikes a balance between AI efficacy and privacy concerns is edge computing. By processing and analyzing data locally on individual devices rather than relying on cloud computing, personal information remains within the user's control, minimizing the risk of privacy breaches. This approach avoids large, centralized datasets, reducing the potential harm in case of a security breach.
To achieve sustainable data privacy, transparency plays a vital role. Users must receive clear explanations regarding how their data is collected, used, and shared by AI systems. Providing individuals with privacy settings and options to control their data is crucial in empowering them to make informed decisions about their information.
Moreover, incorporating privacy protection mechanisms directly into the design phase of AI algorithms is necessary. Employing techniques like privacy by design at early stages ensures that privacy considerations become an integral part of AI system development.
Data privacy concerns in an AI-driven world also call for comprehensive legal frameworks. Governments need to establish robust regulations protecting individuals from misuse of their personal information and ensuring accountability among companies dealing with AI technologies. Striking a careful balance between innovation and regulation will be pivotal in fostering trust and ultimately safeguarding personal information in the age of intelligent machines.
In conclusion, as AI continues to proliferate our society, it is imperative to prioritize data privacy. Addressing concerns related to data breaches, bias, deliberate misuse, security frameworks, user transparency, and legal regulations are fundamental steps towards protecting personal information. By effectively safeguarding data privacy within the realm of AI, we can fully realize the immense benefits this technology has to offer without compromising the trust and dignity of individuals.
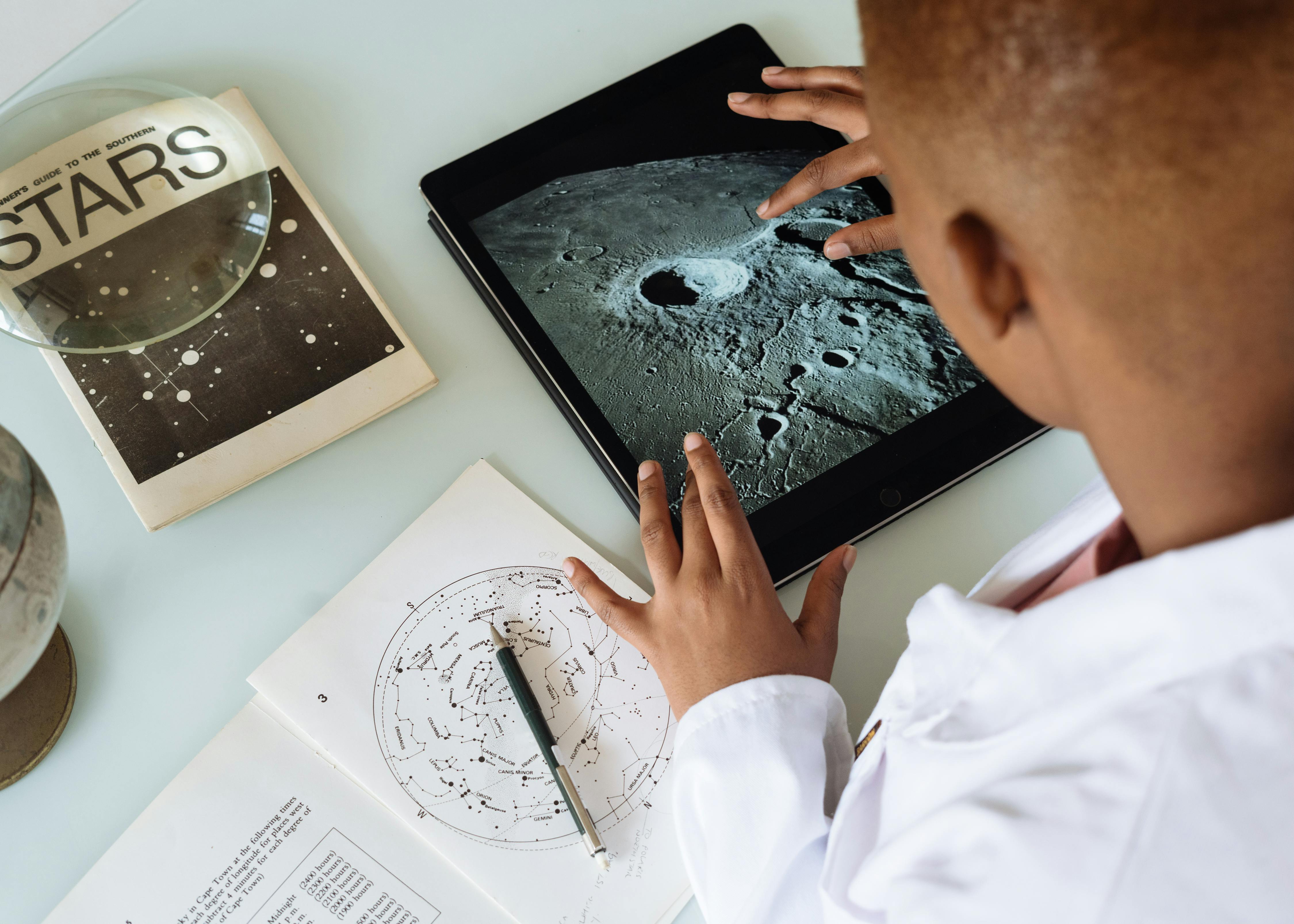
Artificial Intelligence in Healthcare: Revolutionizing Diagnoses, Treatments, and Patient Care
artificial intelligence (AI) has brought forth numerous advancements in healthcare, revolutionizing the ways diagnoses are made, treatments are administered, and patient care is provided. The integration of AI in healthcare has unlocked immense potential to improve outcomes and streamline processes within the medical field.One vital area where AI has made a significant impact is in the realm of diagnosis. By analyzing vast amounts of data and comparing it to known patterns, AI algorithms can identify irregularities and potential diseases with impressive accuracy. This includes reading scans and X-rays, interpreting lab results, and recognizing uncommon symptoms that may otherwise go unnoticed. AI can quickly alert physicians to noteworthy findings, aiding them in making faster and more accurate diagnoses.
Moreover, AI's ability to learn from data enables it to become progressively more precise as it encounters new cases. Through a process known as machine learning, AI can continuously update its algorithms based on the input received from doctors and experts, constantly improving its diagnostic capabilities.
Treatments have also seen significant improvements with the assistance of AI-powered systems. Treatment plans can be crafted precisely tailored to individual patients using predictive analytics techniques, analyzing vast arrays of patient records, genomic data, and medical research. This allows doctors to offer personalized treatment alternatives and select the most effective interventions for better outcomes. AI can even help prevent adverse drug reactions by cross-referencing medication histories and highlighting potential risks unique to certain individuals.
Additionally, AI has accelerated drug discovery processes. It enables researchers to analyze complex molecular structures efficiently and predict how these compounds will interact with the human body. Programs utilizing AI algorithms can rapidly search through massive databases of chemical structures to identify promising drug candidates that may have previously gone unnoticed due to human limitations.
Beyond diagnoses and treatments, AI plays a crucial role in transforming patient care. Chatbots equipped with natural language processing provide patients with on-demand assistance around the clock, answering questions about symptoms, medications, or appointments. These chatbots also aid in educating patients about their conditions by providing information in a comprehensive and relatable manner.
Moreover, AI-powered devices continuously monitor patients remotely, enabling timely interventions in case of emergencies or sudden complications. The data collected from wearables, such as heart rate monitors or sleep trackers, can be analyzed in real-time by AI algorithms, alerting healthcare providers of any concerning changes that necessitate prompt attention. Early intervention enhances patient safety and allows for appropriate care adjustments before emergencies arise.
The integration of AI in healthcare is revolutionizing the industry in countless ways. It enhances diagnostic accuracy, tailors treatments to individual patients, expedites drug discovery, enables round-the-clock patient support, and provides real-time monitoring. As this technology continues to advance, we can expect a future wherein AI plays an even more significant role in improving medical outcomes while efficiently enhancing overall patient care.
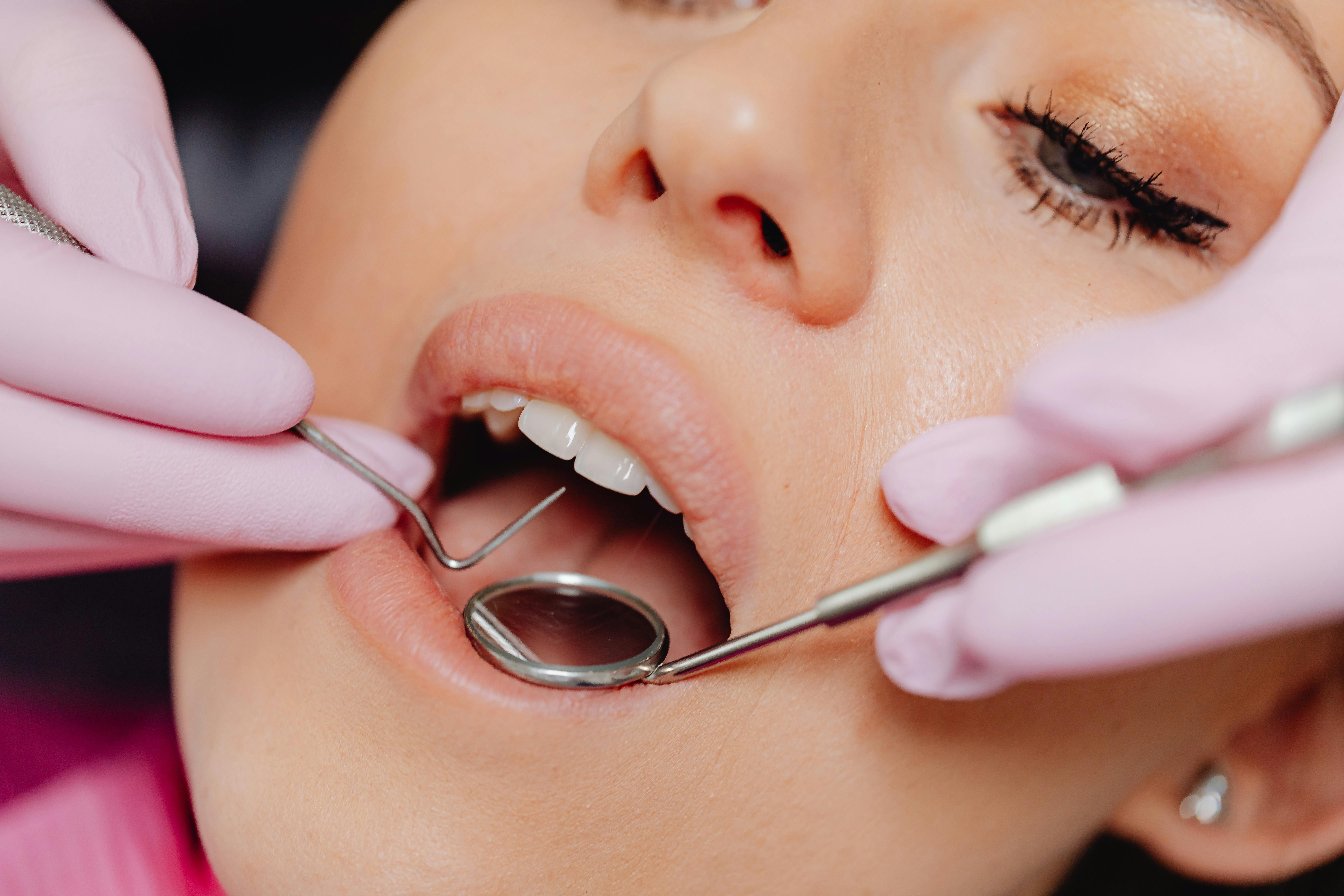
The Role of AI in Environmental Conservation: From Wildlife Protection to Climate Change Mitigation
artificial intelligence (AI) has emerged as a powerful tool in various fields, and its potential for environmental conservation is undeniable. From safeguarding wildlife to addressing climate change concerns, AI is proving to be a valuable asset in our efforts towards a sustainable future.Wildlife protection is one significant area where AI is making a difference. Poaching poses a severe threat to endangered species, but AI-driven technologies like image recognition and machine learning algorithms enable efficient monitoring systems. By analyzing surveillance footage and identifying potential risks, AI helps detect illegal activities and alerts authorities for immediate action. Furthermore, AI-powered drones and cameras aid in monitoring protected areas, capturing real-time images of poachers or tracking movements of animals without disturbing their habitats.
When it comes to climate change mitigation, AI plays a crucial role in gathering, analyzing, and predicting massive volumes of data related to environmental patterns. Machine learning algorithms can process data from satellites, weather stations, and other sources to forecast the impact of climate change accurately. With this information, policymakers are better equipped to develop effective strategies for reducing greenhouse gas emissions and adapting to climate variability.
Similarly, managing energy consumption is vital in combating climate change. AI algorithms can optimize energy usage by monitoring consumption patterns and identifying areas where efficiency can be improved. This further aids companies and individuals in making informed decisions about consuming resources responsibly while minimizing their ecological footprint.
In agriculture, AI offers tremendous potential by optimizing processes such as crop management and pest control. Intelligent sensors analyze soil conditions, weather patterns, and crop health data to provide valuable insights for farmers. With this knowledge at hand, farmers can make precise decisions regarding irrigation, fertilization, and pesticide use—maximizing yields while minimizing environmental harm caused by excessive chemical use.
AI-powered platforms also promote citizen participation in environmental conservation efforts. For instance, smartphone applications equipped with image recognition technology encourage users to report instances of illegal logging or wildlife trafficking. Through crowdsourcing data collection, AI facilitates a more seamless and synchronized approach to conservation.
In conclusion, AI is proving to be an invaluable ally in environmental conservation. From protecting and monitoring wildlife to aiding climate change mitigation efforts, AI-based solutions are transformative. As technology continues to advance, it holds tremendous potential for addressing the planet's most pressing ecological challenges. By harnessing AI's power, we can strive towards a more sustainable future for all.
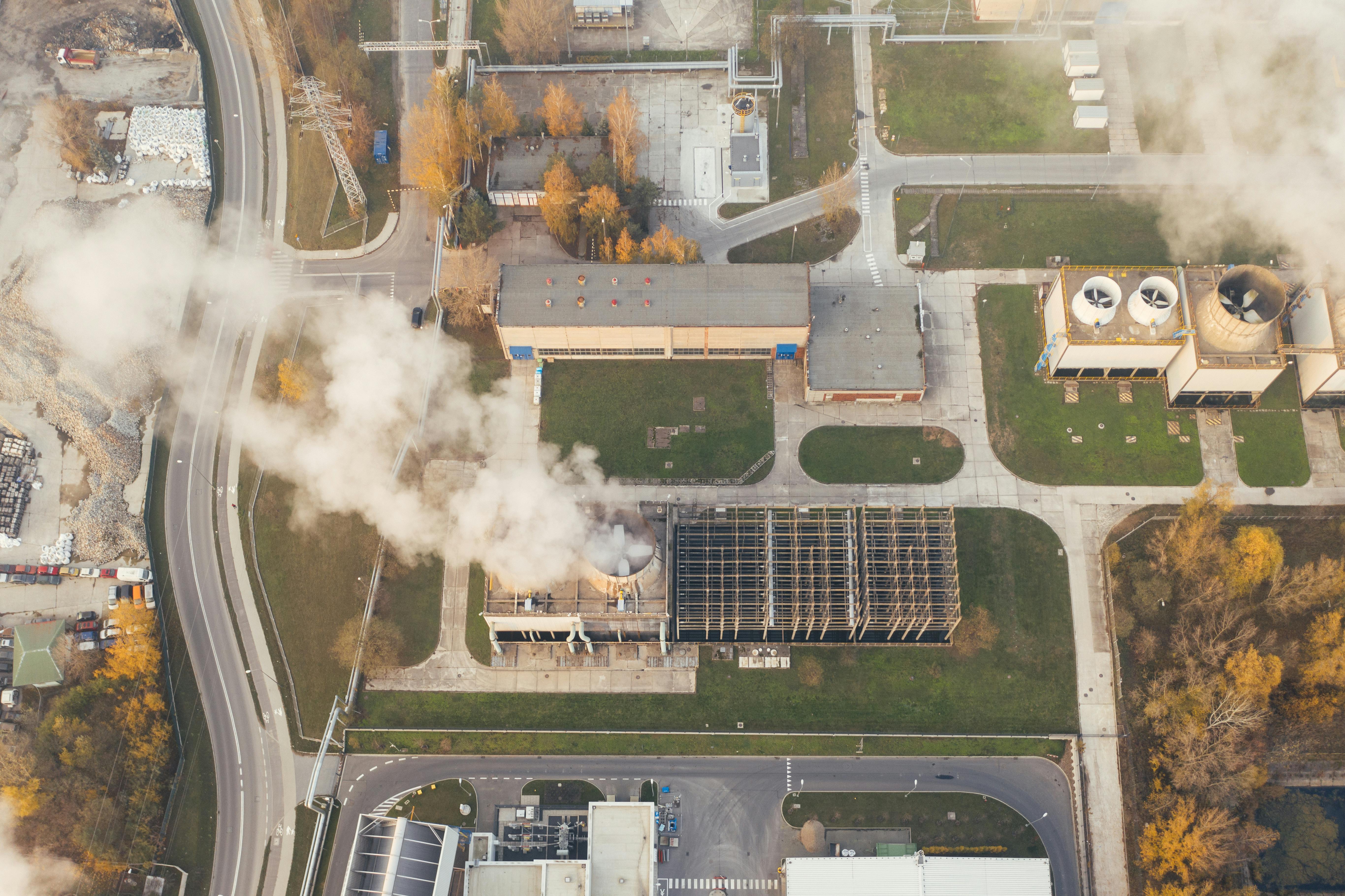
Navigating the Legal Landscape: Intellectual Property and Liability Issues in AI Development
artificial intelligence (AI) has become an integral part of various industries, bringing immense benefits for society. However, with these advancements come challenging legal considerations that need to be addressed. In particular, intellectual property (IP) and liability issues have emerged as crucial aspects within AI development.When it comes to AI and IP, there are a few key elements to understand. First and foremost is the concept of patents. Patents serve as legal protection for new inventions, including AI technology. Companies engaging in AI development may need to obtain patents to safeguard their unique algorithms or models. The challenge lies in determining what can be patented, especially considering the dynamic and rapidly evolving nature of AI applications.
Another vital aspect of IP in AI is copyright law. Copyright protects original creative works, such as software codes or AI-generated content. These laws aim to balance the rights of creators while allowing fair access and use. In the context of AI development, ensuring that copyright principles are respected becomes crucial, whether when training algorithms using copyrighted datasets or generating new works autonomously.
Additionally, trademark law is relevant to AI-driven products and services. Trademarks offer protection for brand names, logos, or slogans associated with goods or services offered by businesses. In an era where AI plays a significant role in aiding marketing and customer interactions, businesses must ensure that their utilization of trademarks complies with legal requirements to avoid confusion or infringement claims.
Moving on to liability issues in AI development, ensuring appropriate accountability and responsibility is pivotal due to the potential risks involved. For instance, if an autonomous AI system were to cause harm, determining who is liable becomes complex since traditional legal frameworks may not adequately address such scenarios.
Liability concerns extend further if biases or discriminatory patterns within AI systems impact individuals or groups unfairly. Addressing these concerns requires careful monitoring during the development phase alongside ethical guidelines and legal parameters defined ahead of time.
Regulatory compliance is also crucial in navigating the legal landscape surrounding AI development. Governments around the world are taking steps to formulate regulations that address both the beneficial and potential risks of AI technologies. Developers must be aware of existing laws, privacy regulations, and other legal considerations unique to their respective jurisdictions when pushing forward with AI projects.
Navigating the legal landscape of AI development demands proactive measures taken by developers, businesses, and policymakers alike. A multidimensional approach involving collaboration with legal experts, staying updated on intellectual property rights, promoting transparency, complying with regulations, and ethical practices can help mitigate infringements, protect IP interests, and navigate liabilities inherent to AI technology. Only through responsible development and continuous adaptation can AI truly thrive while ensuring a fair and legally safe environment.
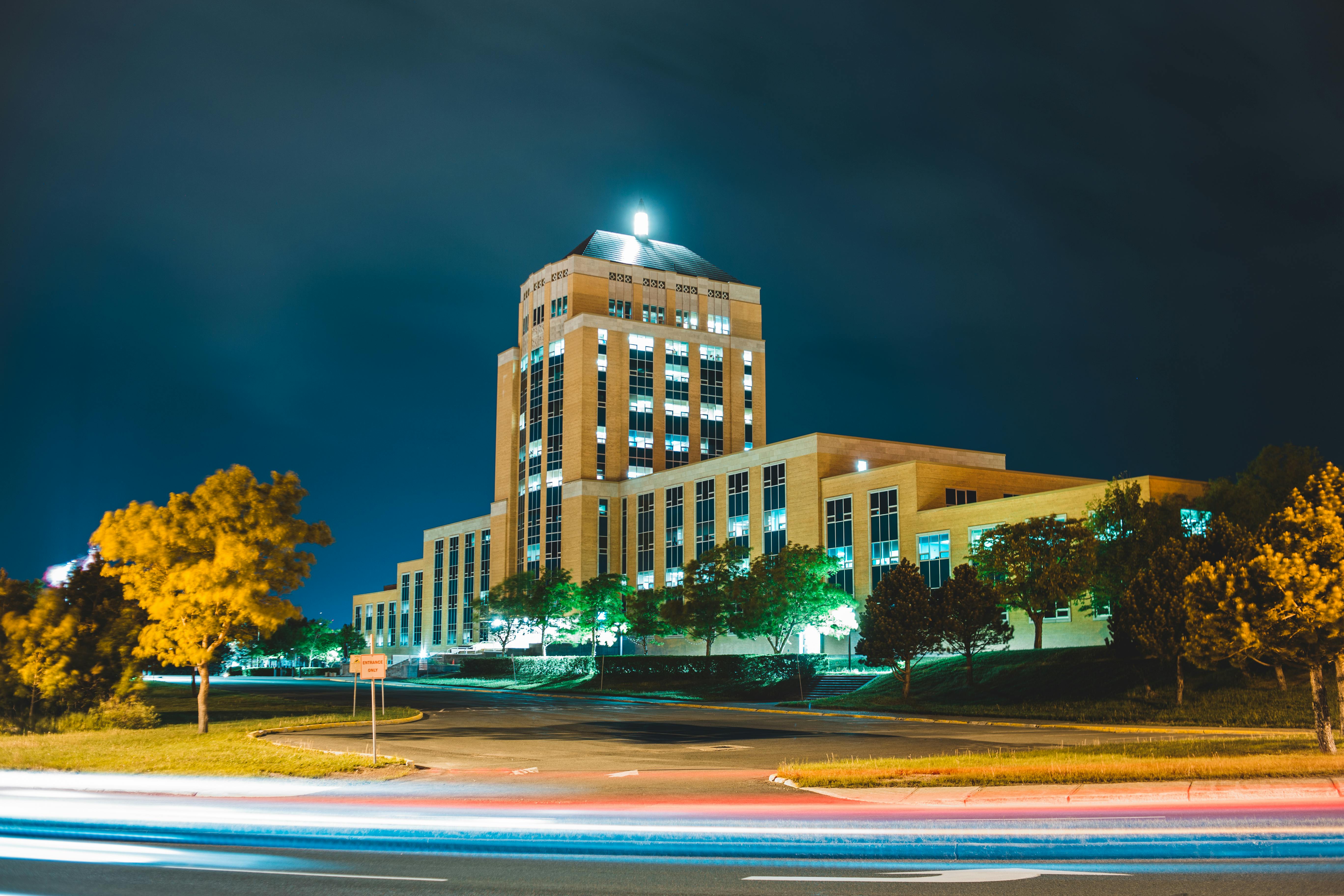
Human-AI Interaction: Enhancing User Experience Through Smart Technologies
Human-AI Interaction, also known as HAI, focuses on the relationship between humans and artificial intelligence systems. It is aimed at enhancing user experience through the use of smart technologies.HAI seeks to create seamless and intuitive interactions between people and AI systems. This involves enabling AI systems to understand human emotions, intentions, and context better, thereby allowing them to provide relevant and personalized responses.
To enhance user experience, researchers and developers focus on several aspects of HAI:
- Natural Language Processing (NLP): NLP enables AI systems to understand and process human language in a way that is both meaningful and informative. This improvement in AI understanding empowers users to interact with AI systems using natural language, making communication more fluid.
- Voice Recognition: Making use of voice recognition allows users to have direct conversations with AI systems by simply speaking to them. This technology facilitates hands-free interactions and fosters a more intuitive user experience.
- User Context Awareness: Understanding the broader context in which an interaction takes place enhances the capabilities of AI systems to respond meaningfully. By leveraging information about a user's location, preferences, history, among other factors, AI systems can provide more accurate and personalized responses.
- Emotion Recognition: Recognizing human emotions during interaction contributes significantly to an improved user experience. When AI understands how a person feels, it can tailor responses accordingly and provide empathetic and supportive interactions.
- Explainable AI: Users crave transparency when interacting with AI systems. Developments in explainable AI aim to bridge this gap by allowing humans to understand the reasoning behind AI decisions. Clear explanations create trust between users and intelligent systems.
- Ethical Considerations: Ensuring positive engagement between humans and AI calls for addressing ethical aspects of conversation design, data privacy, and mitigating bias in algorithmic decision-making.
- User-Centered Design: Adopting a user-centered design approach prioritizes the needs of users at every stage of development. It involves gathering user feedback, conducting usability testing and user research to create intuitive interfaces and personalized experiences.
- Adaptive Interfaces: Building interfaces that adapt to users' preferences, capabilities, and contexts helps enhance user experience. Providing customization options allows users to tailor AI systems to their needs and further improve their interaction quality.
In summary, Human-AI Interaction aims to enhance user experience by developing AI systems that can understand natural language, recognize emotion, adapt to user preferences, cultivate transparency, and foster ethically responsible interactions. Combining these smart technologies allows for more advanced and engaging interactions between humans and artificial intelligence.
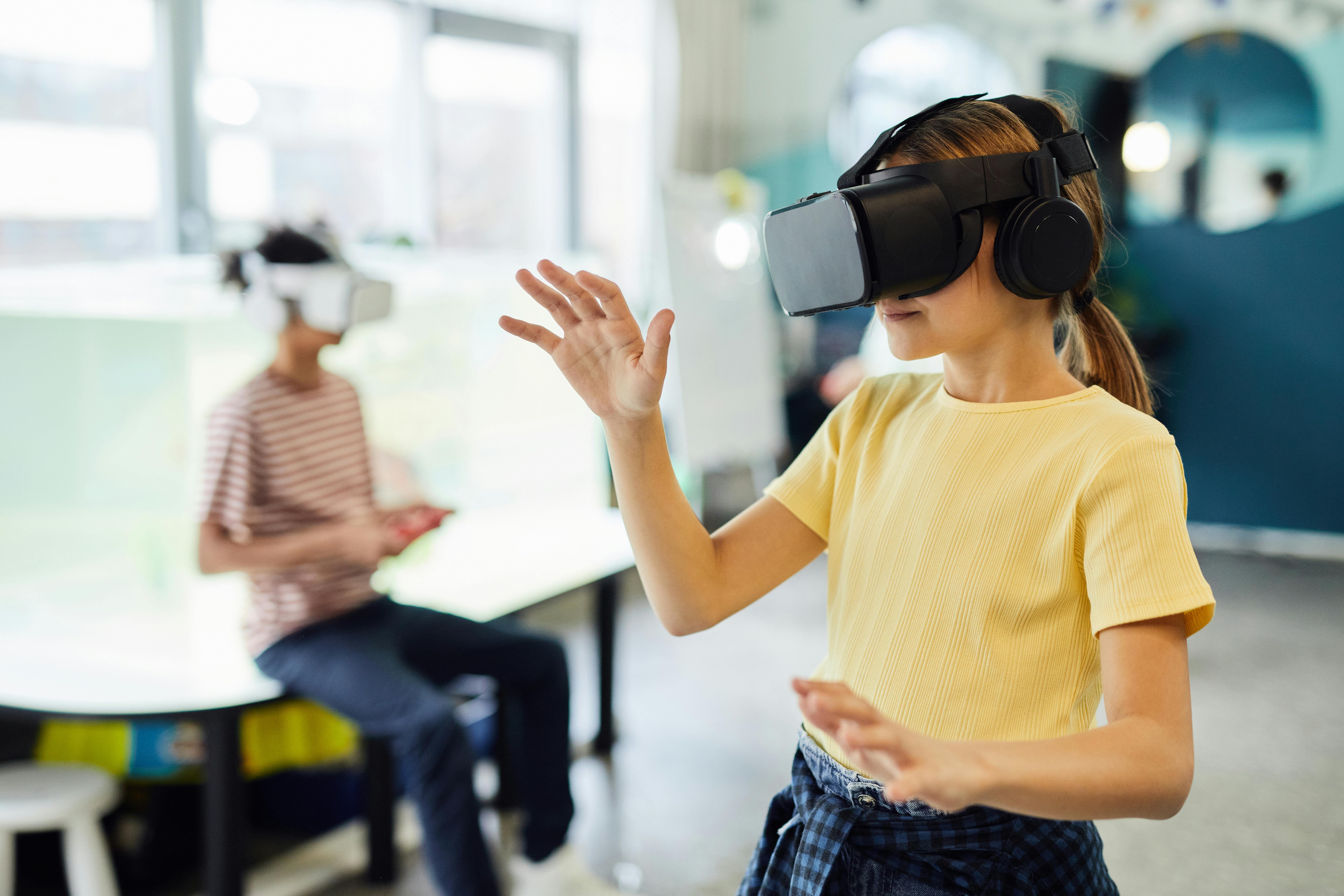
Bias and Fairness in Artificial Intelligence: Tackling Inequality in AI Algorithms
Bias and fairness in artificial intelligence (AI) have become increasingly concerning issues as AI technology has advanced. Tackling inequality in AI algorithms is crucial to ensuring that AI systems do not perpetuate biases prevalent in society. Here's an overview of the concepts related to bias and fairness in AI:- Introduction:
Bias refers to the presence of unfair or prejudiced behaviors or decisions based on certain characteristics, such as gender, race, or age. In AI algorithms, bias can mimic and amplify existing societal inequities, resulting in unjust outcomes. - Types of Bias:
a) Implicit Bias: These are unconscious biases based on personal preferences and stereotypes that can affect decision-making.
b) Dataset Bias: When training data is biased, the AI learns from systemic societal inequalities encoded within that data.
c) Technical Bias: Bias can creep into algorithms through the framing of problems, choice of variables, or flawed methodologies.
3. Sources of Bias in AI Algorithms:
a) Data Collection: Biased data collection methods, skewed sampling procedures, or historical data reflecting inequitable human decisions can introduce bias.
b) Preprocessing: The techniques applied before inputting the data into the algorithm may inadvertently introduce bias if not handled carefully.
c) Algorithmic Design: Biases might emerge due to certain assumptions made during algorithm design, inadequate representation across various groups, or unaddressed feedback loops.
4. Impact of Bias:
Biased AI systems reinforce inequality by perpetuating existing disparities. They can result in biased decision-making related to hiring practices, lending policies, criminal justice systems, and more – thus delivering unequal treatment to different individuals or groups.
5. Challenges in Addressing Bias:
a) Transparency: Lack of understanding about how algorithms make predictions makes it challenging to identify biased outcomes.
b) Trade-offs: Pursuing perfect fairness might entail unintended consequences or a trade-off between different fairness dimensions, leading to ethical dilemmas.
c) Interpretability: Certain sophisticated AI models lack interpretability, making it difficult to ascertain why or how biased decisions were reached.
6. Approaches to Tackle Bias:
a) Diverse and Representative Datasets: Collecting diverse data that includes underrepresented groups can help mitigate dataset bias.
b) Fairness Metrics and Evaluation: Developing fairness metrics to measure algorithmic performance and evaluating the impact on different demographic groups is important.
c) Algorithmic Interventions: Techniques such as reweighing samples, modifying features, or fine-tuning the model can be employed to reduce biased outcomes.
d) Independent Audits: Third-party audits and evaluations can help identify bias in AI systems and hold developers accountable.
7. Ethical Considerations:
The responsibility of addressing bias and ensuring fairness in AI algorithms lies with both developers and policymakers. Addressing discrimination requires critical ethical considerations at every step – from data collection to training, implementation, and continuous monitoring.
In conclusion, ensuring fairness in AI algorithms necessitates proactive measures like diversifying datasets, incorporating fairness metrics, and conducting regular audits. By deploying unbiased AI systems, we move towards building a more equitable society where access to opportunities is not hindered by discriminatory technology.
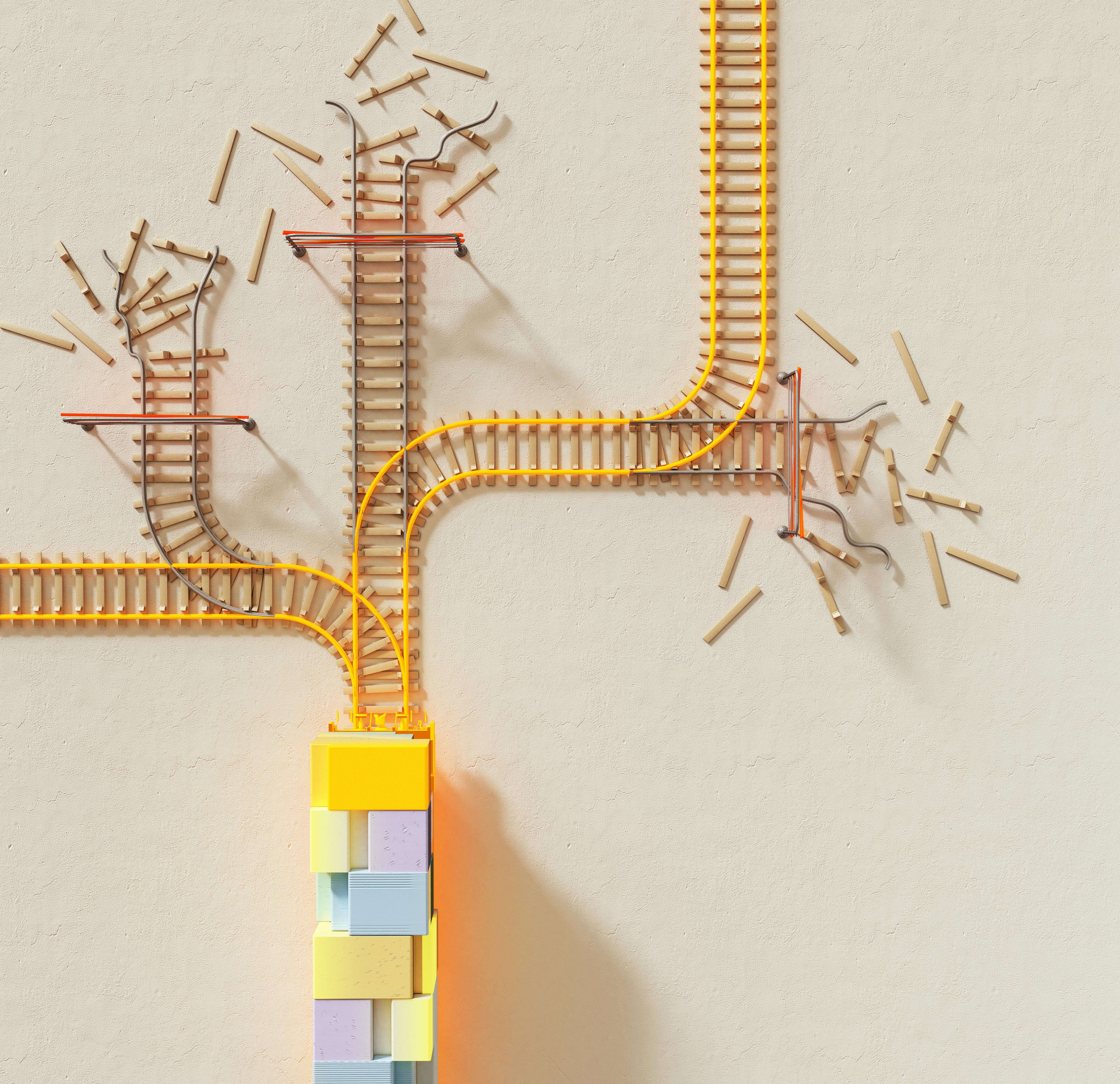
The Global Race for AI Supremacy: National Strategies and Their Impact on Worldwide Innovation
artificial intelligence (AI) has emerged as a breakthrough technology with far-reaching implications across various sectors. Its potential has ignited a fierce global race, where nations strive to gain supremacy in AI research, development, and implementation. Understanding the national strategies behind this race and their impact on worldwide innovation is crucial to grasp the future landscape of AI.Many countries have recognized AI's transformative power and have made significant investments in research and development. The United States dominates the race, thanks to its robust innovation ecosystem, top-tier universities, and abundant venture capital. Key industry giants are based in the US, attracting top computing talent and fostering AI advancements. The American strategy focuses on private-public partnerships, emphasizing technical leadership, ethical standards, and data-driven innovation.
China displays a strong AI ambition, driven by its scale and rapid economic growth. The Chinese government views AI as pivotal for future competitiveness and has designated it a strategic priority. State funding fuels extensive AI investments, supporting research institutions and companies alike. China aims for technological self-sufficiency while simultaneously capturing the global market. This involves heavy investments in AI hardware, applications in surveillance systems, facial recognition technology, autonomous vehicles, and various other areas.
Europe also actively participates in the race with its initiatives such as the European Union's AI High-Level Expert Group and member states' local projects. Acknowledging ethical considerations, EU emphasizes a human-centric approach when deploying AI technologies. Research collaborations among European nations aim to foster shared knowledge while retaining data privacy and user rights. Moreover, Europe seeks to empower small and medium-sized enterprises by promoting ethical practices in emerging AI sectors.
Countries like Canada have emerged as key players in the AI race due to their supportive policies and vibrant research communities. Canada's commitment to universal healthcare acts as an incentive for researchers to deploy AI-driven solutions efficiently. It provides access to health records for large-scale data analysis, spurring innovation in healthcare and AI applications. Other countries, including Japan and South Korea, have also invested substantially in AI research to secure their positions in this competitive landscape.
The impact of national strategies on worldwide innovation is multi-faceted. Competition within the race drives progress, pushing nations to excel in AI technologies, infrastructure, and talent attraction. The sharing of knowledge and collaboration between participating countries allows for cross-pollination of ideas, accelerating innovation. However, concerns related to data protection, privacy, ethics, and technological inequalities need to be addressed collaboratively to avoid fragmentation and ensure responsible development.
In conclusion, the global race for AI supremacy signifies a concerted effort by nations worldwide to gain a competitive edge. The strategies pursued by different countries encompass technological advancements, ethical considerations, funding, talent acquisition, and more. As this race progresses, cooperation for shared knowledge and responsible development becomes vital to maximize the benefits of AI while addressing its social implications. Only through international collaboration can we navigate this evolving technological landscape successfully.
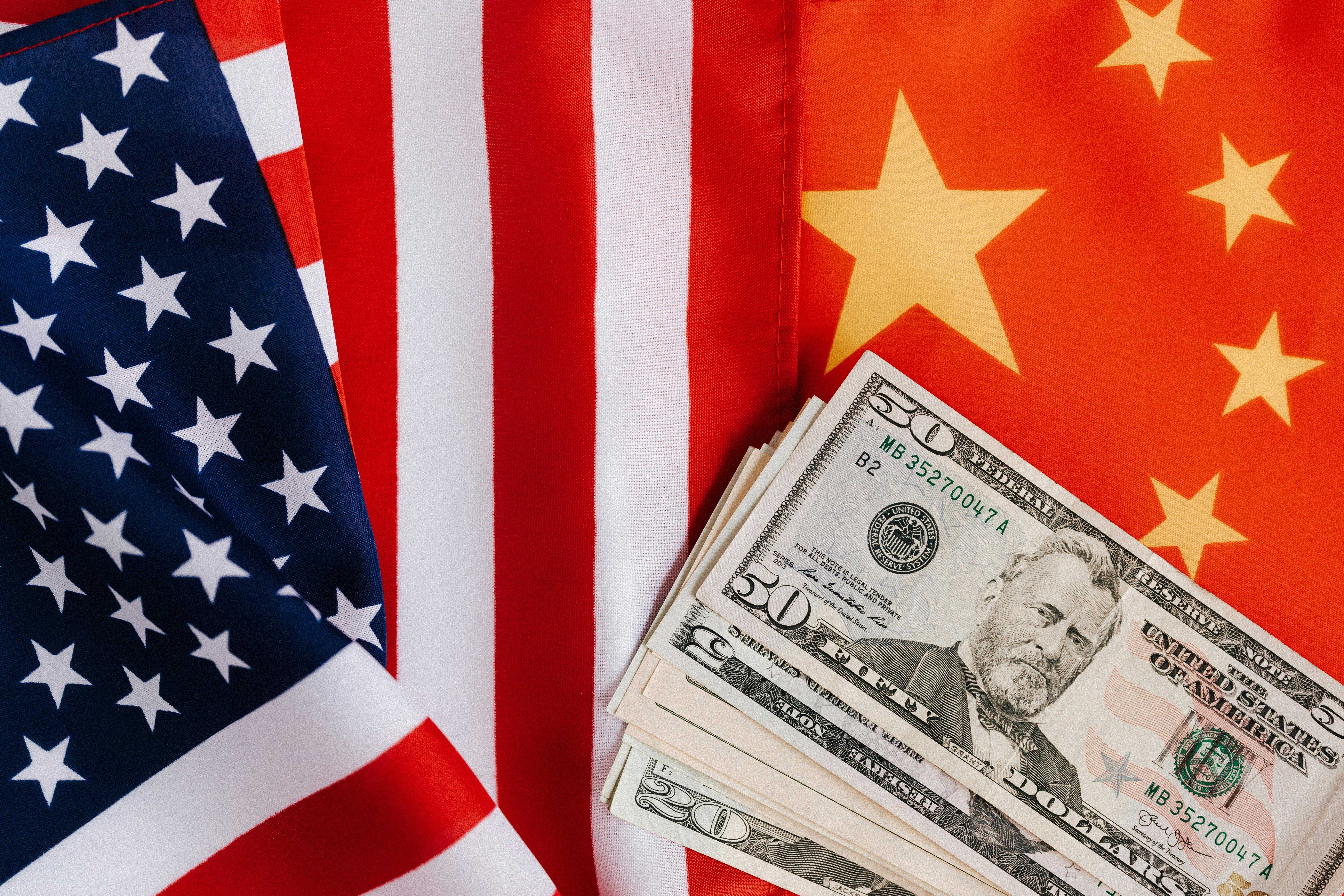
Investing in AI Technology: Opportunities, Risks, and Future Trends for Investors
artificial intelligence (AI) has swiftly emerged as a transformative technology with vast potential across numerous industries. For investors, this presents enticing opportunities alongside inherent risks. Understanding these dynamics and future trends is crucial when considering investing in AI technology.Opportunities:
- Increased efficiency: AI drives automation, streamlining processes and augmenting productivity in various sectors such as manufacturing, logistics, and customer support.
- Enhanced decision-making: AI enables data analysis at unparalleled speed and accuracy, empowering businesses to make data-driven decisions that can lead to improved outcomes.
- Healthcare advancements: Investing in AI technologies allows for better diagnostics, research, drug discovery, and personalized treatments contributing to significant advancements in healthcare.
- Autonomous vehicles: The flourishing autonomous vehicle industry demonstrates the potential for monetizing AI-powered products that have far-reaching implications on transportation systems globally.
- Smart homes and cities: AI can create smarter living environments, enabling connected devices to enhance comfort, security, and energy management.
Risks:
- Ethical implications: The deployment of AI technology raises concerns involving privacy, bias, and job displacement. Inadequate regulation and oversight may create unforeseen consequences or amplify existing societal inequalities.
- Technological limitations: Despite significant progress, AI still faces limitations in handling ambiguous situations or making independent judgments. It is imperative to consider the boundaries of AI before investing.
- Economic disruptions: Job displacement due to automation could result in economic challenges if adequate measures aren't taken to bridge skill gaps or provide alternative employment opportunities.
- Uncertain returns: Predicting the financial success of a particular AI investment can be difficult due to rapidly evolving technologies and unpredictable market dynamics.
Future Trends:
- Natural Language Processing (NLP): Advancements in NLP will facilitate more seamless interactions between humans and machines by enhancing language understanding and generating contextually accurate responses.
- AI democratization: As AI technologies mature, they are becoming more accessible and affordable to a broader range of businesses, creating new opportunities for investors across various sectors.
- AI in personalized medicine: Investing in precision medicine, where therapies are tailored to individual patient characteristics, holds immense promise by leveraging AI-enabled analysis of massive datasets.
- Robotics and automation: Investors directing funds towards AI-powered robotics and automation stand to capitalize on evolving trends that reshape industries like manufacturing, agriculture, and healthcare.
In conclusion, investing in AI technology offers diverse opportunities for investors seeking new growth avenues. However, it is essential to cautiously assess the associated risks and carefully monitor future trends to maximize investment potential while ensuring ethical considerations are addressed.
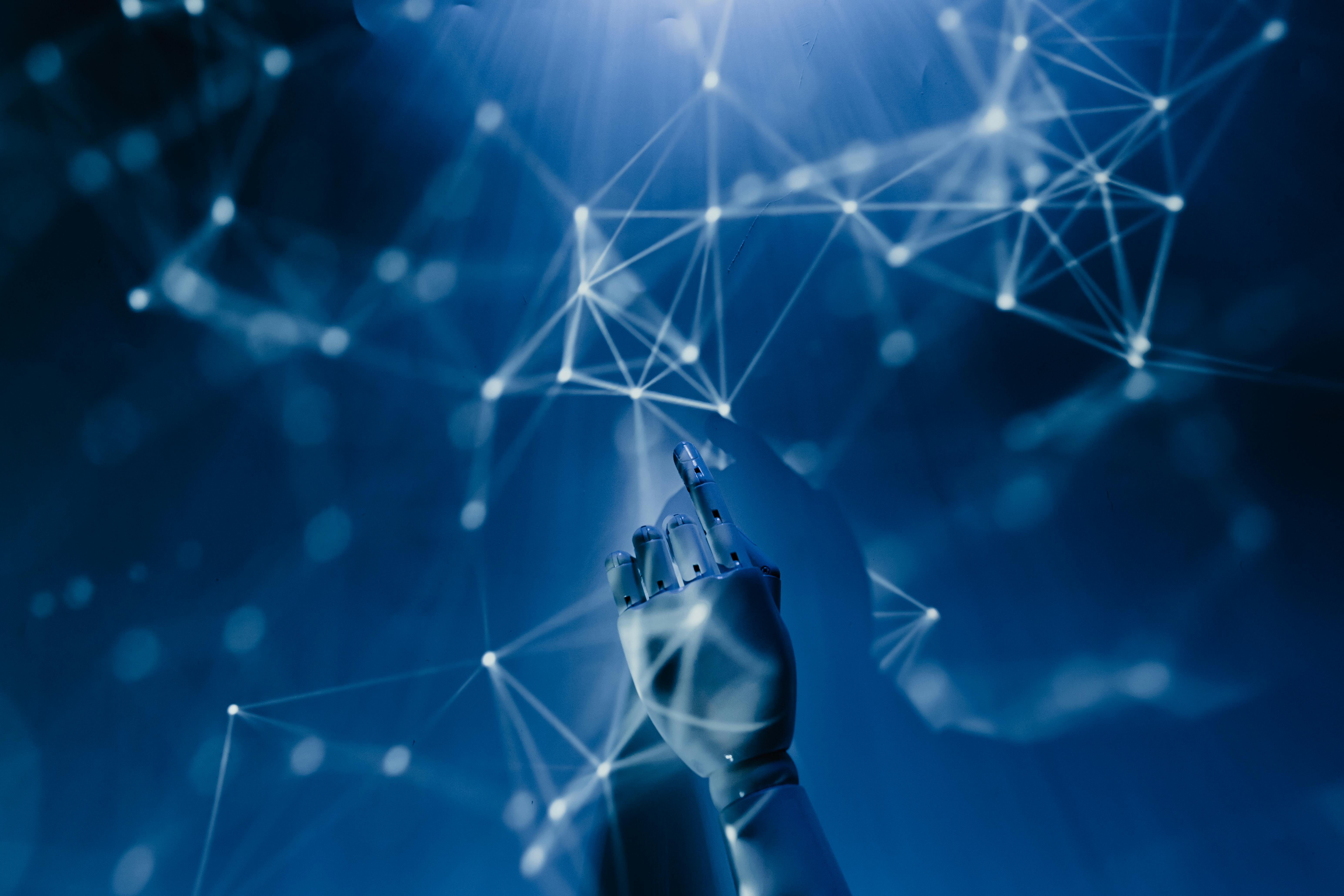
Bridging the Digital Divide: Ensuring Equal Access to AI Advancements Across Socioeconomic Strata
The digital divide, a term referring to the inequality in access to information and communication technologies (ICTs), has become a pressing concern in the era of artificial intelligence (AI) advancements. The rapid evolution and penetration of AI technologies have the potential to exacerbate existing socioeconomic disparities if not addressed properly. To ensure equal access to AI advancements across all strata, it is crucial to understand the challenges, work toward alleviating them, and implement inclusive policies.At its core, AI is built on diverse data sets that power machine learning algorithms, enabling computers to perform tasks that would typically require human intelligence. By automating and enhancing various processes, AI has opened doors to new opportunities across industries. However, this progress is meaningful only if it is accessible to all socioeconomic backgrounds.
The digital divide encompasses multiple dimensions, including infrastructural limitations, connectivity disparities, affordability concerns, and lack of digital literacy. High-speed internet access continues to elude many marginalized communities and even certain rural areas. Unequal distribution of affordable devices capable of running advanced AI applications poses another hurdle. Moreover, limited technological literacy is a major challenge faced by individuals who are less exposed to technology or underserved by traditional education systems.
To bridge this gap, collaborative efforts are necessary from governments, tech companies, educational institutions, and nonprofits. Policy interventions should focus on infrastructure development for underserved areas and remote regions, aiming at expanding broadband coverage universally. Moreover, promoting affordability should involve subsidizing devices or encouraging the development of low-cost alternatives without compromising functionality.
Elevating digital literacy becomes crucial in this context. Initiatives should be taken to educate individuals from all walks of life on the intricacies of AI technology and its potential benefits. This can include integrating technology training programs into schools and community centers. Furthermore, campaigns aimed at fostering diversity within AI research and development can yield alternative perspectives, addressing biases that disproportionately affect certain socioeconomic groups.
To succeed in bridging the digital divide and ensuring equal access to AI advancements, collaboration must extend beyond traditional boundaries. Partnerships between state and non-state actors need to be fostered, shaping inclusive policies and programs. By promoting a level playing field, we can eliminate the hurdles hindering disadvantaged individuals from enjoying and benefiting from advancements in AI technology.
In conclusion, the digital divide when it comes to AI access across socioeconomic strata requires attention and actions. Overcoming infrastructural limitations, ensuring widespread connectivity, promoting affordability, and enhancing digital literacy are key factors in making progress. By focusing on collaborative efforts and inclusive policies, we can bridge the gap and create a future where AI advancements are accessible to everyone, regardless of their socioeconomic background.
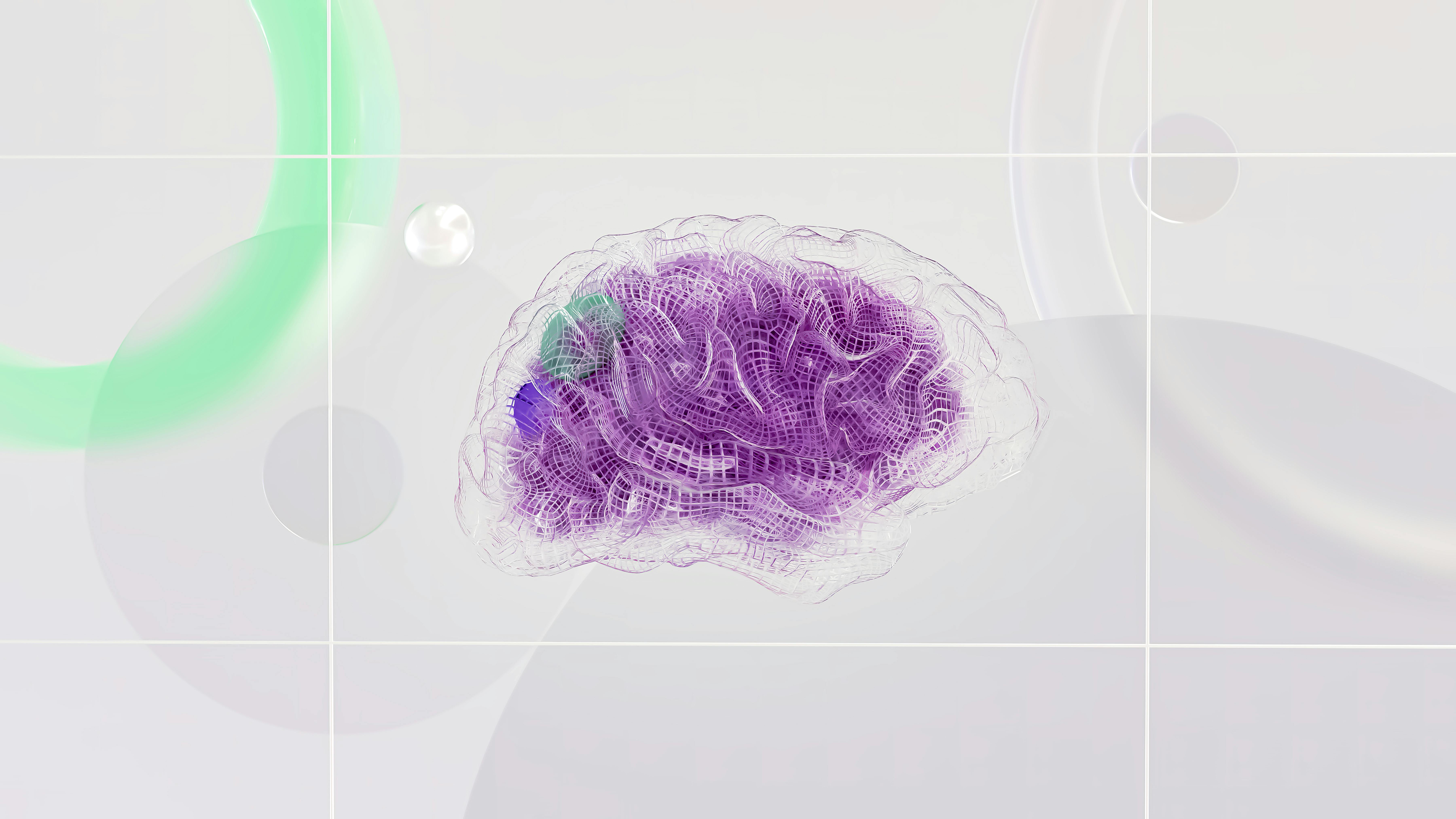
Cultivating Trust in AI Systems Through Transparency and Accountability Mechanisms
When it comes to artificial intelligence (AI) systems, cultivating trust becomes crucial in ensuring their acceptance and successful integration into our lives. Trust is especially important because AI algorithms can sometimes be complex and difficult to comprehend for the average user. Transparency and accountability mechanisms play a pivotal role in building trust between humans and AI systems. Here are several key aspects to consider in this regard.- Understandable Explanations: A transparent AI system should provide understandable explanations for its decision-making process. Users must be able to understand why an AI system reached a certain conclusion or recommendation. By revealing the logic behind the decision, users can better evaluate the accuracy and reliability of the AI system.
- Clear Data Source: It is vital for AI systems to clearly communicate the sources of data on which they are trained. Users need to know which datasets were used, how they were collected, and any potential bias present in the data. This information helps users assess if the AI system has been trained on a comprehensive and diverse dataset.
- Disclosure of Algorithm Performance: Transparency also involves sharing performance details of AI algorithms. By disclosing information on an AI system's accuracy, sensitivity levels, and limitations, users gain insight into its capabilities. This empowers users to make informed decisions about when and how to trust the AI system.
- Open Peer Review: One way to enhance trust is through open peer review processes. Independent researchers examining the underlying algorithm of an AI system can help reveal any biases or shortcomings, as well as provide recommendations for improvement. Open discussions and constructive critiques facilitate accountability and foster transparency in developing more reliable systems.
- Ethical Considerations: Accountability mechanisms include guidelines that ensure ethical behavior from AI developers and operators. Compliance with regulations that protect individuals' privacy rights and prevent discrimination is essential to maintain trust in AI systems.
- User Control & Customization: To build trust, AI systems should allow users some level of control over their functioning. This can include explainability features that provide more detailed reasoning for decisions or recommendations, as well as open policy settings that enable reconfiguring the AI system's behavior within defined boundaries. Allowing users to customize their AI interaction promotes a sense of empowerment and bolster trust.
- Thorough Testing: Rigorous testing is crucial to evaluate the performance, robustness, and potential biases of AI systems. Independent auditing and certification processes can ensure AI algorithms meet prescribed standards. These examinations enhance accountability and increase transparency, reassuring users about an AI system's reliability.
Transparency and accountability mechanisms are fundamental in cultivating trust in AI technologies. By incorporating these mechanisms into AI development and deployment, we can bridge the gap between human understanding and AI decision-making processes. Such efforts not only foster trust but also enable us to leverage AI systems confidently and responsibly for a multitude of applications across different domains.
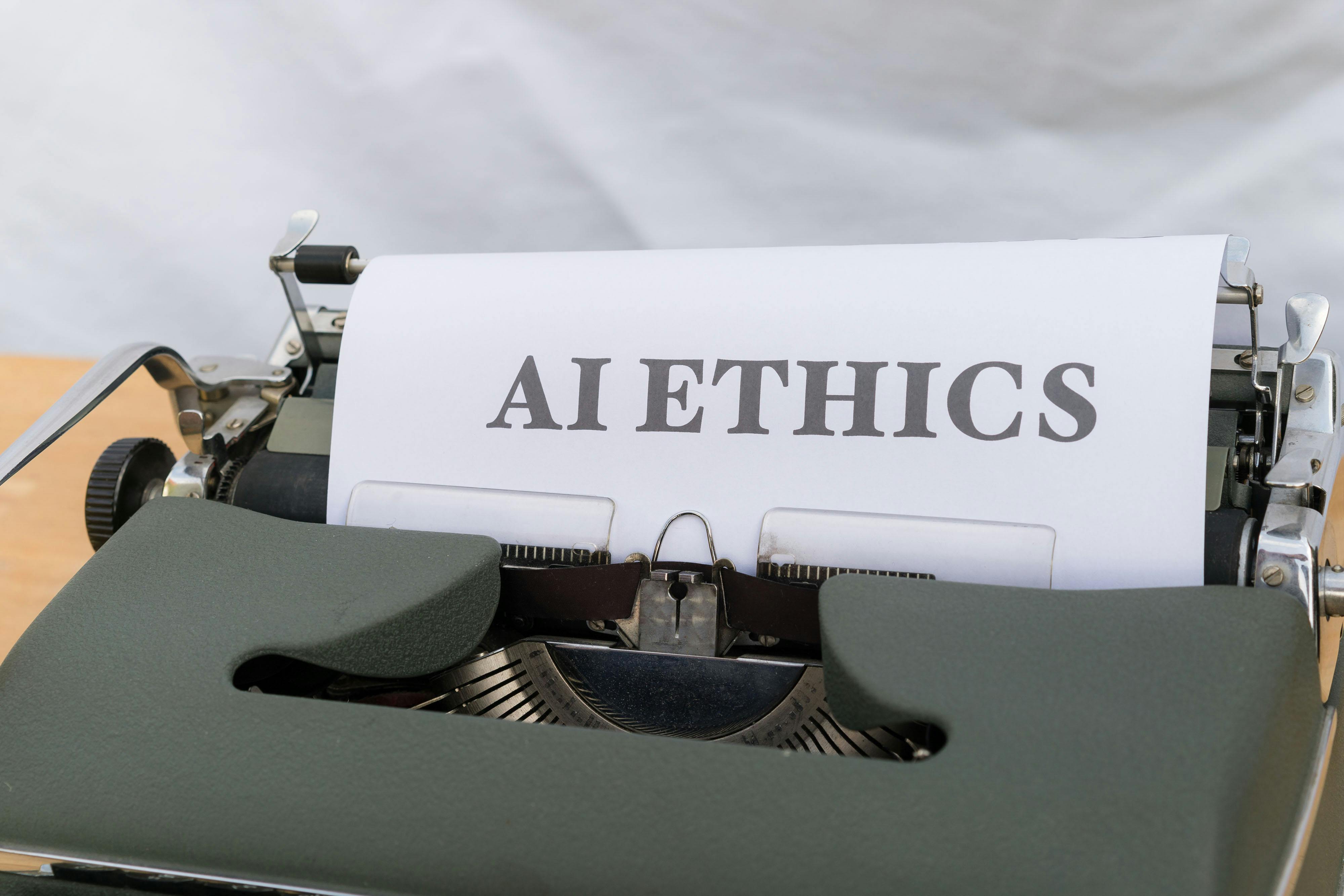
Exponential Technologies and AI: Exploring the Convergence of Machine Learning with Blockchain, VR, and IoT
Exponential technologies, when combined with artificial intelligence (AI), have immense potential for transforming various aspects of our lives. One such area of exploration involves the convergence of machine learning with blockchain, virtual reality (VR), and the internet of things (IoT).At its core, AI refers to the simulation of human intelligence processes by machines. Machine learning, a subset of AI, allows systems to learn from data patterns and make informed decisions without explicit programming. As AI technologies progress, the concept of using machine learning in conjunction with other exponential technologies is gaining prominence.
Blockchain, a decentralized peer-to-peer network technology, is fundamentally focused on enhancing security, transparency, and trust in various applications. The marriage of machine learning and blockchain introduces new possibilities – offering decentralized AI systems that are governed by user consensus rather than centralized authorities. This convergence allows for increased trust in AI outputs, enhanced privacy protection through encrypted data handling, and improved auditability of decisions made by AI models.
VR technology immerses users in computer-generated three-dimensional environments that closely mimic reality or simulate diverse scenarios. Combining AI with VR can offer rich interactive experiences. By leveraging machine learning capabilities in VR systems, users can benefit from personalized experiences that adapt to their preferences and behaviors. AI-powered VR can also facilitate realistic simulations, thus playing a significant role in training individuals for complex tasks, such as medical procedures or hazardous operations.
The IoT ecosystem entails interconnected devices capable of sharing data over networks autonomously. Integrating AI capabilities into IoT devices allows for smarter decision-making at the edge nodes, reducing the need for central data processing. Machine learning algorithms applied to IoT systems can enable real-time analytics, anomaly detection, predictive maintenance, and improved energy efficiency. This convergence paves the way for intelligent homes, smart cities, and advanced industrial automation with self-learning abilities.
Together, these converging technologies create an environment where various sectors can benefit greatly. For example, a healthcare system utilizing all three technologies could employ AI-powered VR simulations for medical training, securely store patient data through blockchain, and enable IoT-enabled wearables to monitor patients' vital signs. Similarly, transportation industries can implement AI algorithms that optimize traffic flow by harnessing data from interconnected vehicles and urban infrastructure, secured by blockchain.
Despite the immense potential, challenges remain in integrating these technologies seamlessly. Adapting AI algorithms to function efficiently on low-power IoT devices is an ongoing concern, as is ensuring scalability and maintaining user privacy within blockchain networks. Additionally, the rapid advancement of these technologies calls for comprehensive legal and ethical frameworks to address emerging challenges related to bias, accountability, and safety.
In conclusion, the convergence of machine learning with blockchain, VR, and IoT holds vast and exciting possibilities. This integration allows for enhanced security, personalized experiences, and smarter decision-making across various domains. However, it also brings forth noteworthy challenges that require balanced consideration as we accelerate our journey towards a future driven by interconnected exponential technologies.
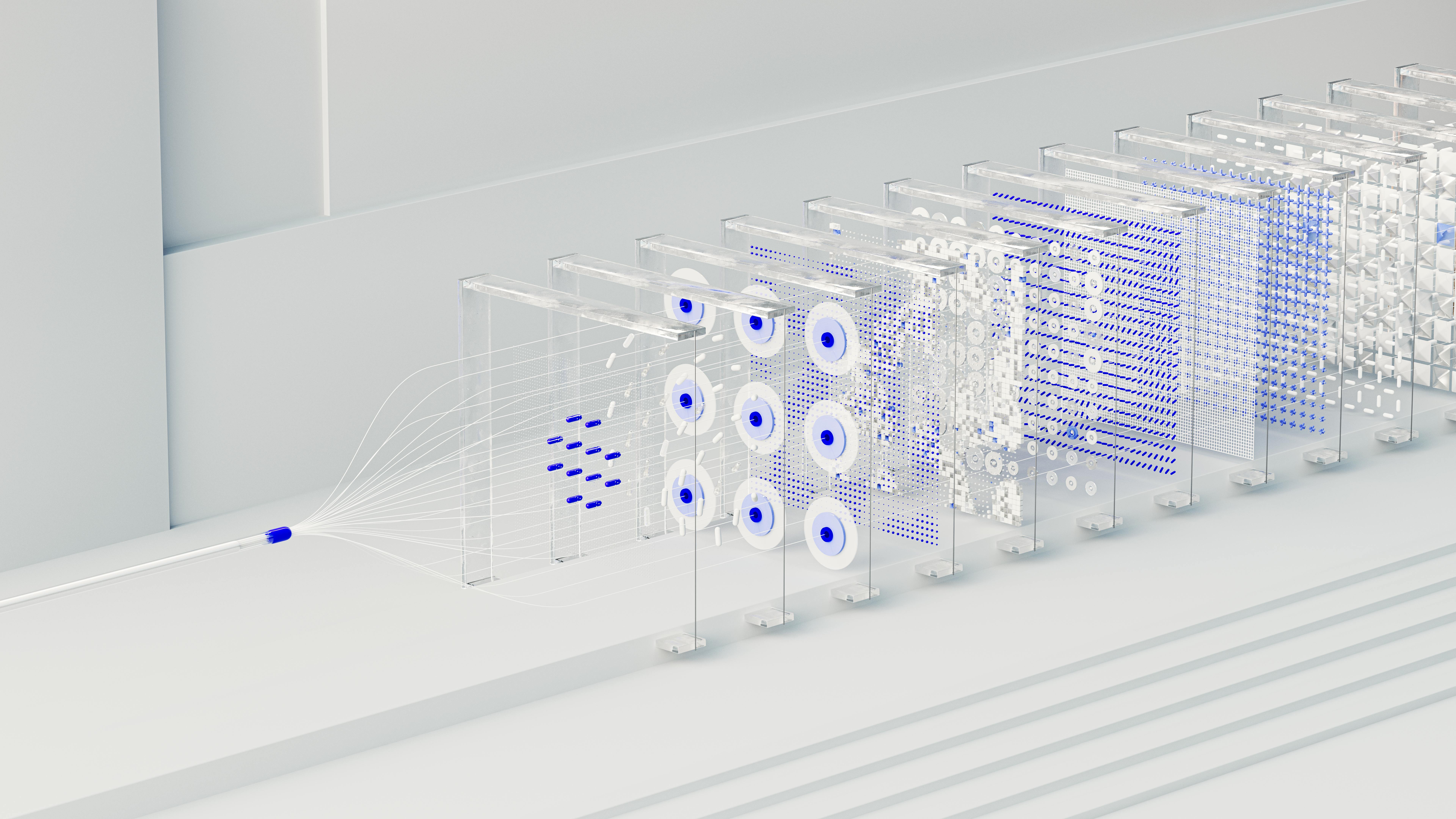